Handling Class Imbalance in Machine Learning-based Prediction Models: A Case Study in Asthma Management
2023 45TH ANNUAL INTERNATIONAL CONFERENCE OF THE IEEE ENGINEERING IN MEDICINE & BIOLOGY SOCIETY, EMBC(2023)
摘要
A data-driven prediction tool has the potential to provide early warning of an asthma attack and improve asthma management and outcomes. Most previous machine learning (ML)-based studies for asthma attack prediction have reported a severe class imbalance, with major implications for model performance. We aimed to undertake a systematic comparison of several class imbalance handling techniques in the context of risk prediction models for asthma prognosis. We used data from 9,835 asthma patients extracted from the Medical Information Mart for Intensive Care (MIMIC) IV database and deployed five class imbalance handling methods based on synthetic minority oversampling technique (SMOTE) and cost function customisation. We then compared their performances in improving two-class classifier models developed using logistic regression (LR) and extreme gradient boosting (XGBoost) for three different prediction tasks with varying severity of class imbalance (proportion of majority class ranging from 90.86% to 98.98%). The cost function customisation technique substantially outperformed the SMOTE-based methods in all tasks. XGBoost combined with cost function customisation achieved the highest prediction performance for the outcome with the most extreme class imbalance ratio (AUC = 0.72). Our findings suggest that the cost function customisation-based approach to tackle class imbalance provides substantially better performance compared to oversampling in the context of asthma management.
更多查看译文
AI 理解论文
溯源树
样例
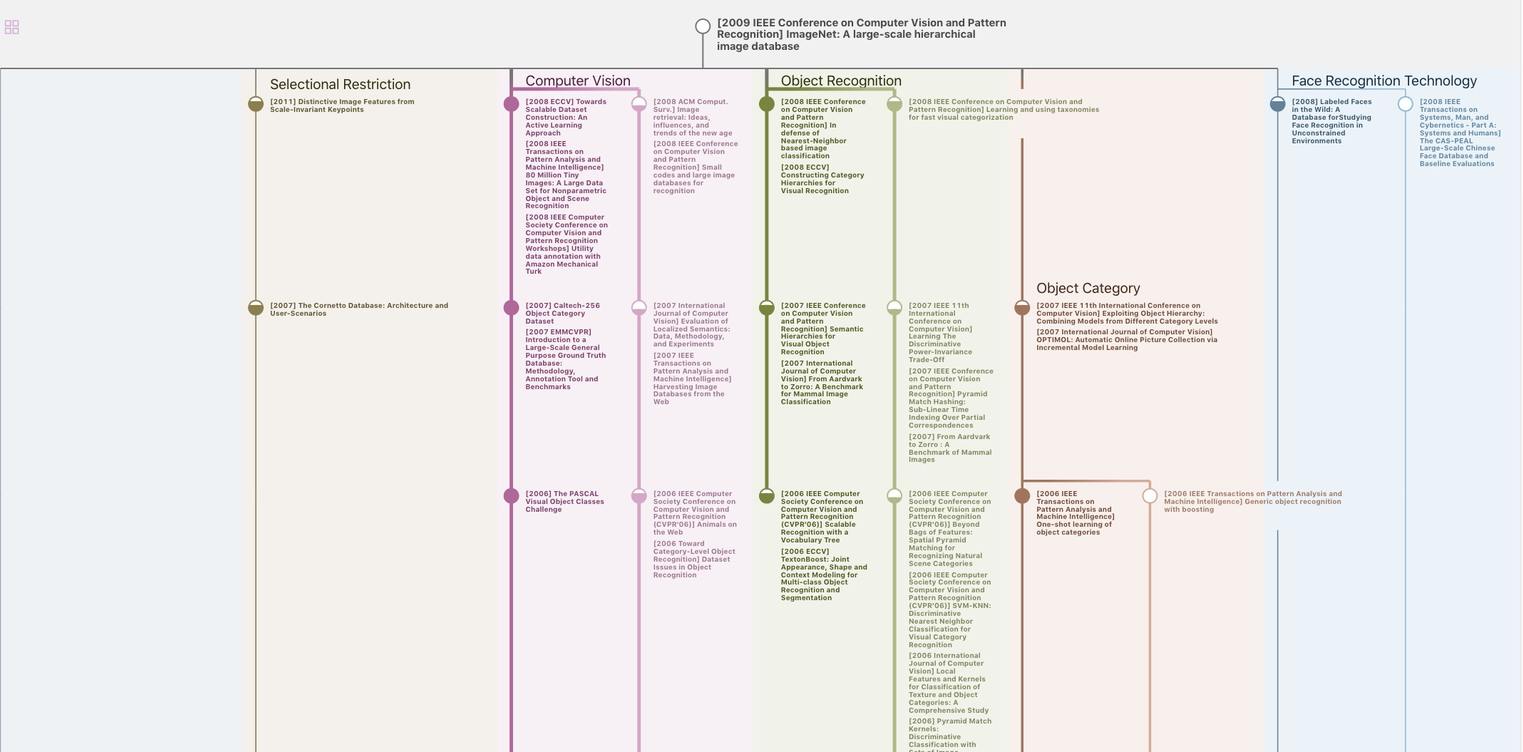
生成溯源树,研究论文发展脉络
Chat Paper
正在生成论文摘要