Sparse feature selection via local feature and high-order label correlation
Applied Intelligence(2024)
摘要
Recently, some existing feature selection approaches neglect the correlation among labels, and almost manifold-based multilabel learning models do not considered the relationship between features and labels, which result in reducing classification effect. To overcome the shortcomings, our work develops a fresh sparse feature selection approach via local feature and high-order label correlation. First, the sparse processing is performed by applying the l 2,1 norm on the weight coefficient matrix, and a loss function between the sample and label matrices can be established to explore the potential relationship between features and labels. The designed loss function can be directly sparse by the weight coefficient matrix, which calculates this weight of each feature, and then some features with higher scores are selected. Second, the combination of manifold learning and Laplacian score is used to deal with local features to make full use of local feature correlation. The manifold regularization for the embedded feature selection can guide exploring potential real labels and selecting different features for individual labels. Finally, to safeguard the rank of the high-order label matrix from being damaged, a self-representation strategy is employed. Then the high-order label weight matrix and the label error term are defined to enhance the accuracy of label self-representation and correct the deviation between the self-representation scheme and the real label, and the Frobenius and the l 2 norm regularization can avoid those trivial solutions and overfitting issues. A representation function of high-order label correlation is proposed based on the self-representation strategy, which can accurately represent the potential information between high-order labels. Thus, those local features are scored by the Laplacian score for different features to select an optimal feature subset with higher scores. Experiments on 16 multilabel datasets illustrate that our constructed algorithm will be efficient in obtaining important feature set and implementing powerful classification efficacy on multilabel classification.
更多查看译文
关键词
Feature selection,Loss function,Manifold learning,High-order label correlation
AI 理解论文
溯源树
样例
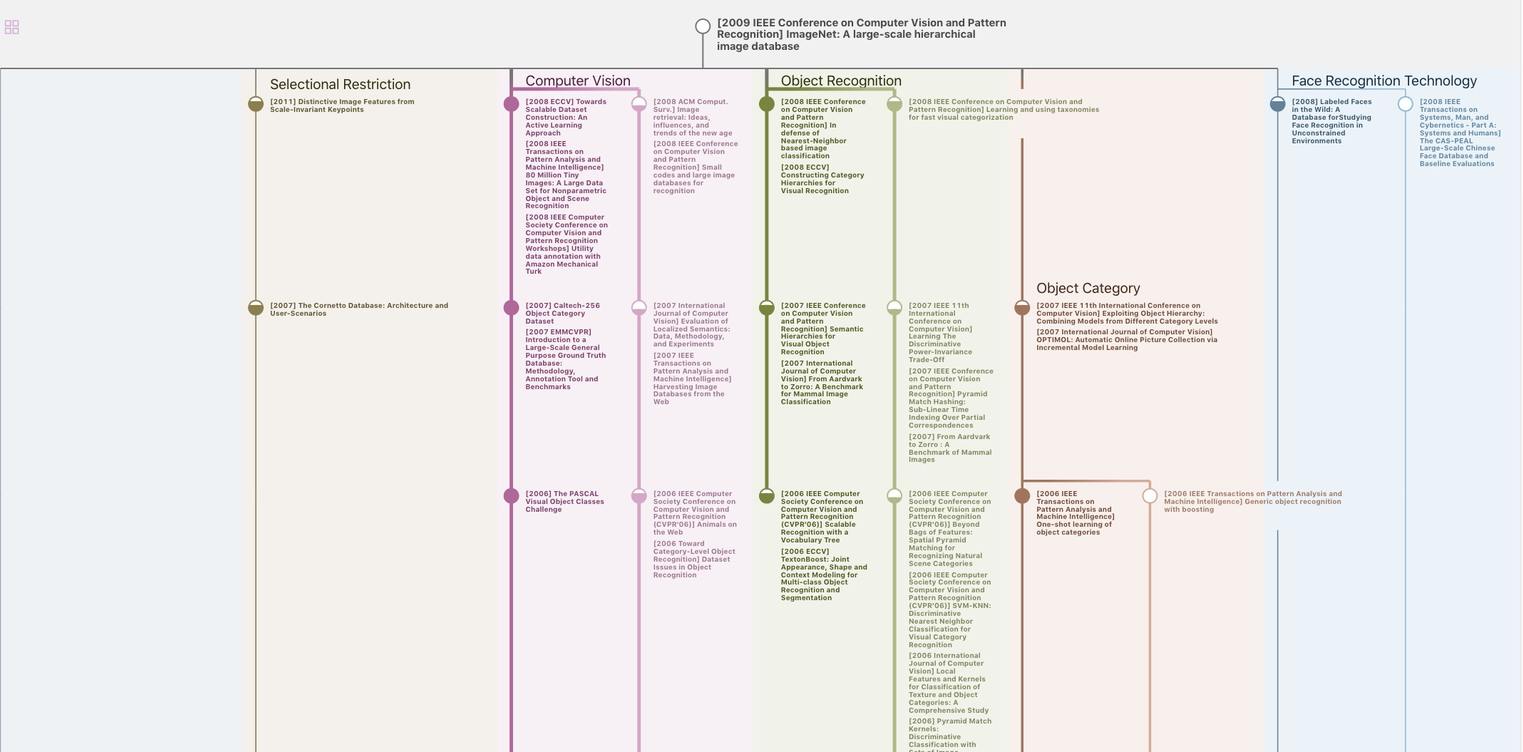
生成溯源树,研究论文发展脉络
Chat Paper
正在生成论文摘要