Multi-and many-objective optimization: present and future in de novo drug design
FRONTIERS IN CHEMISTRY(2023)
摘要
de novo Drug Design (dnDD) aims to create new molecules that satisfy multiple conflicting objectives. Since several desired properties can be considered in the optimization process, dnDD is naturally categorized as a many-objective optimization problem (ManyOOP), where more than three objectives must be simultaneously optimized. However, a large number of objectives typically pose several challenges that affect the choice and the design of optimization methodologies. Herein, we cover the application of multi- and many-objective optimization methods, particularly those based on Evolutionary Computation and Machine Learning techniques, to enlighten their potential application in dnDD. Additionally, we comprehensively analyze how molecular properties used in the optimization process are applied as either objectives or constraints to the problem. Finally, we discuss future research in many-objective optimization for dnDD, highlighting two important possible impacts: i) its integration with the development of multi-target approaches to accelerate the discovery of innovative and more efficacious drug therapies and ii) its role as a catalyst for new developments in more fundamental and general methodological frameworks in the field.
更多查看译文
关键词
drug discovery,de novo drug design,evolutionary algorithms,multi-objective optimization,many-objective optimization
AI 理解论文
溯源树
样例
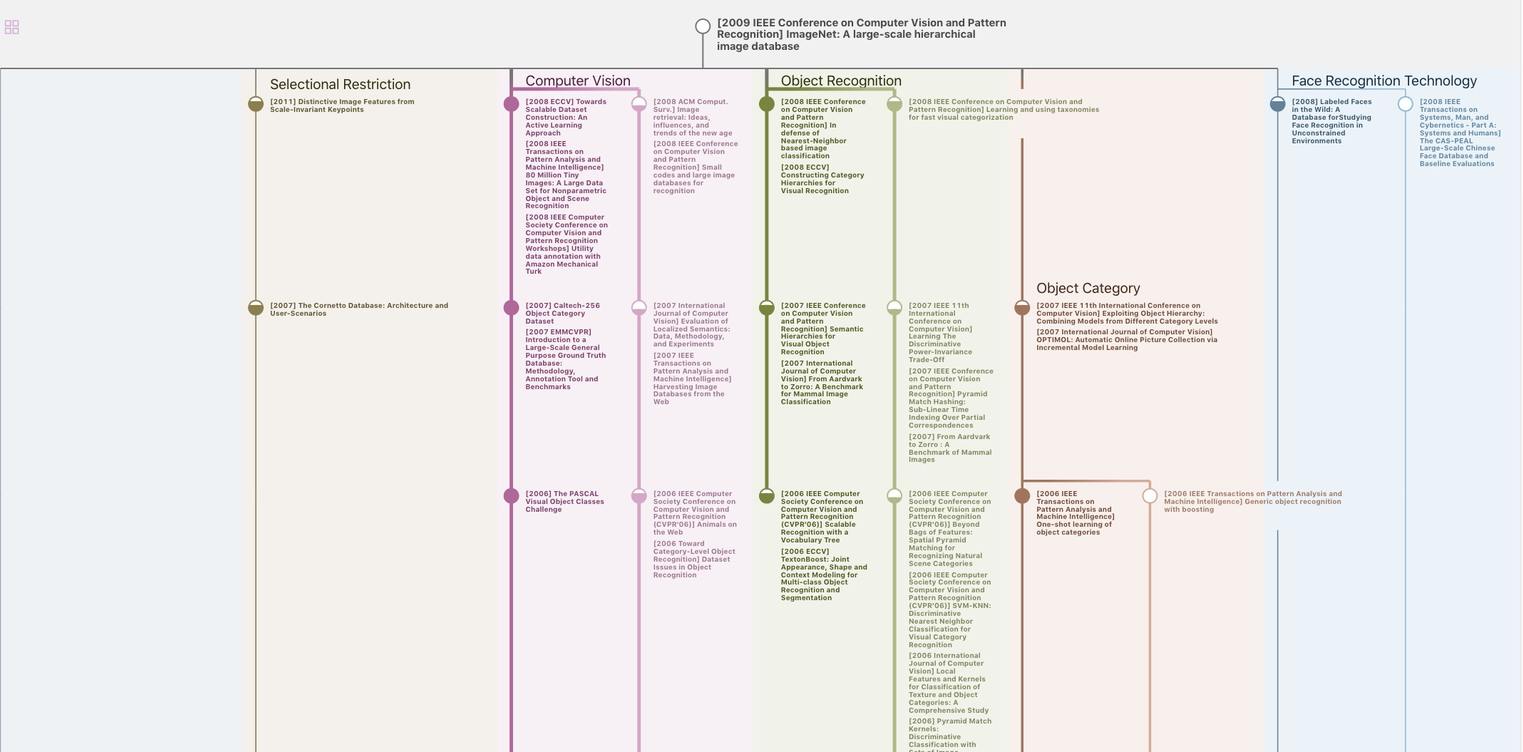
生成溯源树,研究论文发展脉络
Chat Paper
正在生成论文摘要