DTP-Net: Learning to Reconstruct EEG Signals in Time-Frequency Domain by Multi-Scale Feature Reuse.
IEEE journal of biomedical and health informatics(2024)
Abstract
Electroencephalography (EEG) signals are prone to contamination by noise, such as ocular and muscle artifacts. Minimizing these artifacts is crucial for EEG-based downstream applications like disease diagnosis and brain-computer interface (BCI). This paper presents a new EEG denoising model, DTP-Net. It is a fully convolutional neural network comprising Densely-connected Temporal Pyramids (DTPs) placed between two learnable time-frequency transformations. In the time-frequency domain, DTPs facilitate efficient propagation of multi-scale features extracted from EEG signals of any length, leading to effective noise reduction. Comprehensive experiments on two public semi-simulated datasets demonstrate that the proposed DTP-Net consistently outperforms existing state-of-the-art methods on metrics including relative root mean square error (RRMSE) and signal-to-noise ratio improvement ( ∆SNR). Moreover, the proposed DTP-Net is applied to a BCI classification task, yielding an improvement of up to 5.55% in accuracy. This confirms the potential of DTP-Net for applications in the fields of EEG-based neuroscience and neuro-engineering. An in-depth analysis further illustrates the representation learning behavior of each module in DTP-Net, demonstrating its robustness and reliability.
MoreTranslated text
Key words
Electroencephalography (EEG),artifact removal,deep learning,fully convolutional neural network,signal reconstruction,time-frequency domain
AI Read Science
Must-Reading Tree
Example
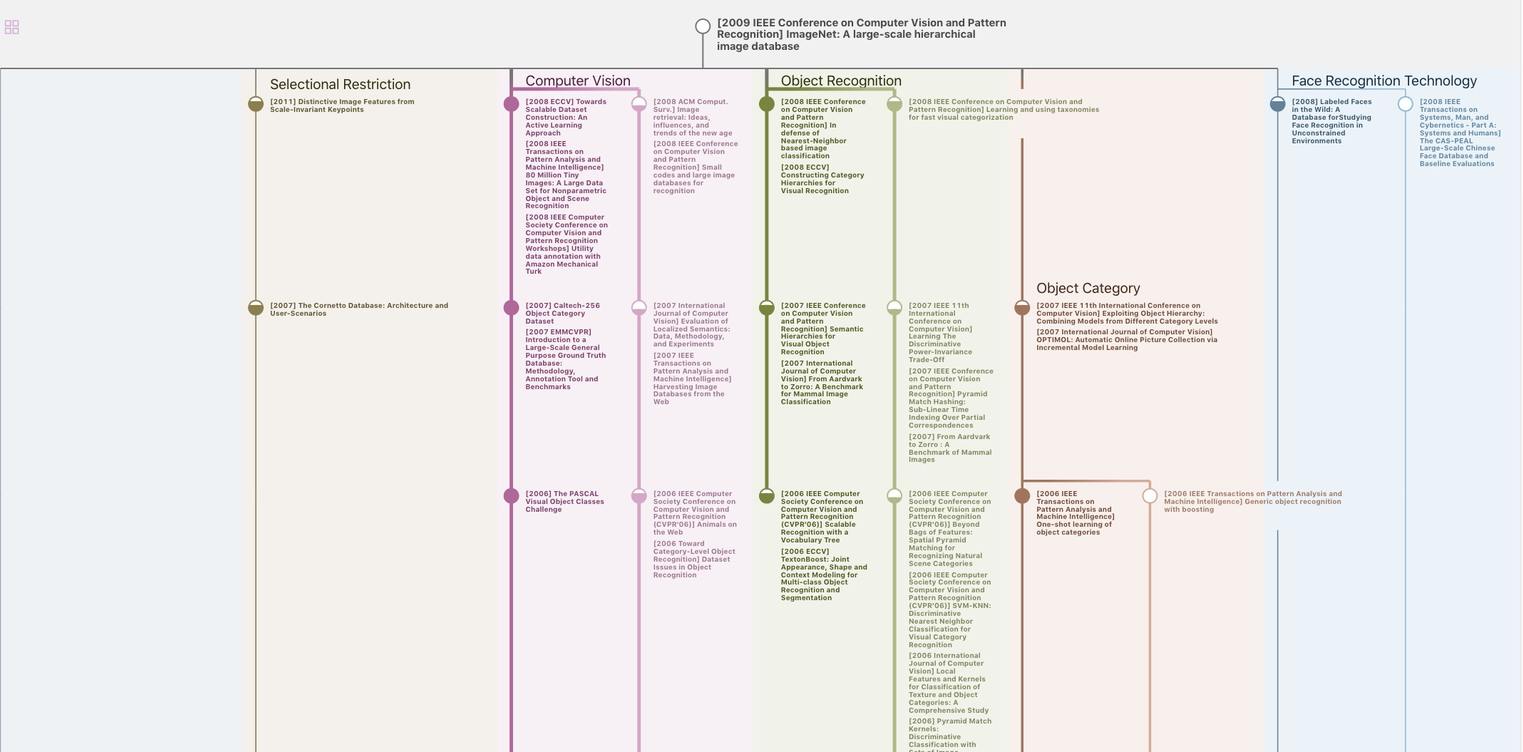
Generate MRT to find the research sequence of this paper
Chat Paper
Summary is being generated by the instructions you defined