End-to-End Training of Neural Networks for Automotive Radar Interference Mitigation
2023 IEEE International Radar Conference (RADAR)(2023)
摘要
In this paper we propose a new method for training neural networks (NNs) for
frequency modulated continuous wave (FMCW) radar mutual interference
mitigation. Instead of training NNs to regress from interfered to clean radar
signals as in previous work, we train NNs directly on object detection maps. We
do so by performing a continuous relaxation of the cell-averaging constant
false alarm rate (CA-CFAR) peak detector, which is a well-established algorithm
for object detection using radar. With this new training objective we are able
to increase object detection performance by a large margin. Furthermore, we
introduce separable convolution kernels to strongly reduce the number of
parameters and computational complexity of convolutional NN architectures for
radar applications. We validate our contributions with experiments on
real-world measurement data and compare them against signal processing
interference mitigation methods.
更多查看译文
关键词
Neural networks,machine learning,FMCW radar,radar interference mitigation,end-to-end training,convolutional neural networks,separable convolutions,object detection
AI 理解论文
溯源树
样例
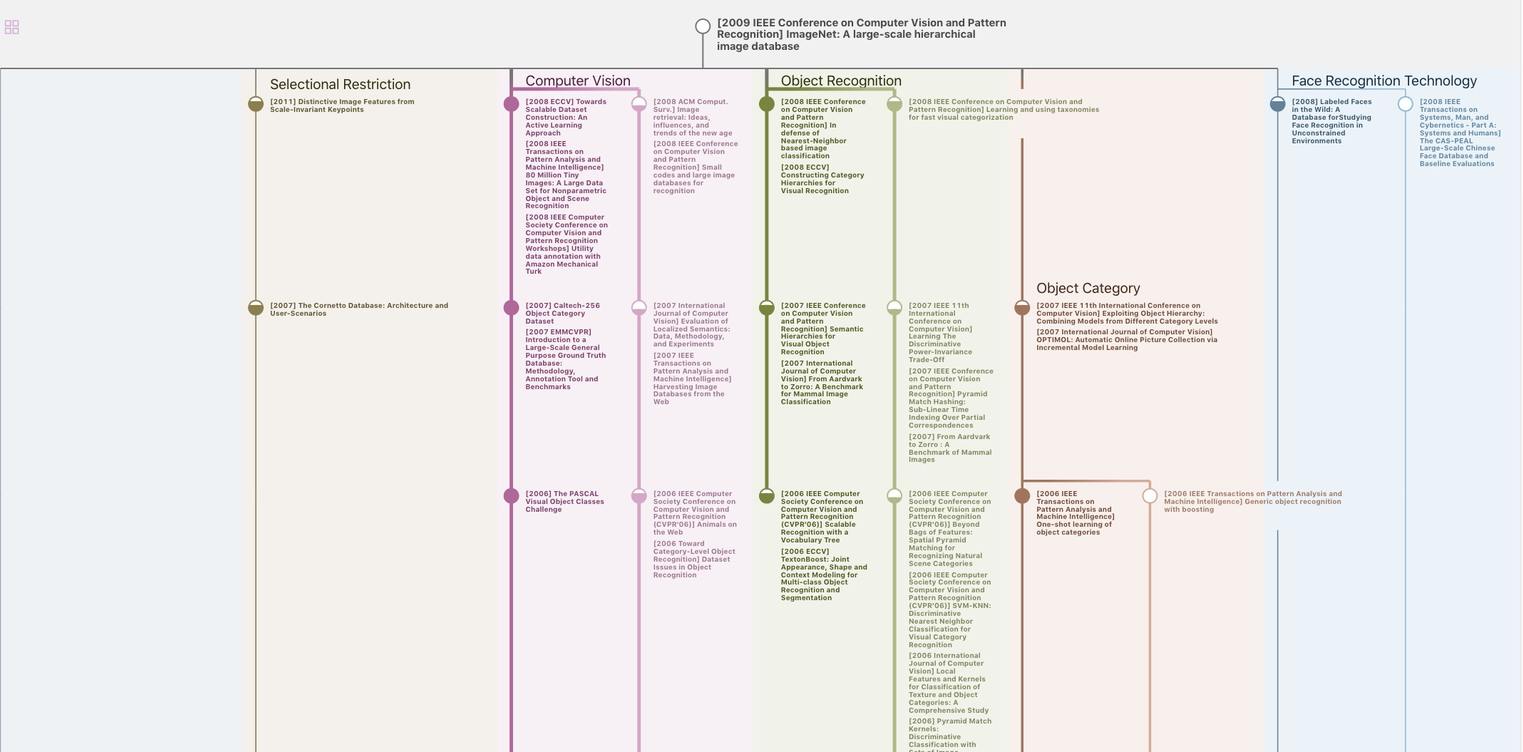
生成溯源树,研究论文发展脉络
Chat Paper
正在生成论文摘要