Multi-branch reverse attention semantic segmentation network for building extraction
EGYPTIAN JOURNAL OF REMOTE SENSING AND SPACE SCIENCES(2024)
摘要
Extraction of color and texture features of buildings from high-resolution remote sensing images often encounters the problems of interference of background information and varying target scales. In addition, most of the current attention mechanisms focus on building key feature selection for building extraction optimization, but ignore the influence of the complex background. Hence, we propose incorporating a novel reverse attention module into the network. The innovative module enables the model to selectively extract crucial building features while suppressing the impact of intricate background noise. It mitigates the influence of uniform spectral and structurally similar heterogeneous background targets on building segmentation and extraction. As a result, the overall generalizability of the model is improved. The reverse attention can also emphasize and amplify the specific details pertaining to the boundaries of the target. Furthermore, we couple a new multi-branch convolution block into the encoder, integrating dilated convolutions with multiple dilation rates. Compared to other methods that use only one multi-scale module to extract multi-scale information from high-level features, we use different receptive field convolutions to simultaneously capture multi-scale targets from multi-level features, effectively improving the ability of the model to extract multi-scale building features. The experimental findings demonstrate that our proposed multi-branch reverse attention semantic segmentation network achieves IoU of 90.59% and 81.79% on the well-known WHU building and Inria aerial image datasets, respectively.
更多查看译文
关键词
Reverse attention,Multi-branch convolution block,Semantic segmentation,Building extraction,High spatial resolution imagery
AI 理解论文
溯源树
样例
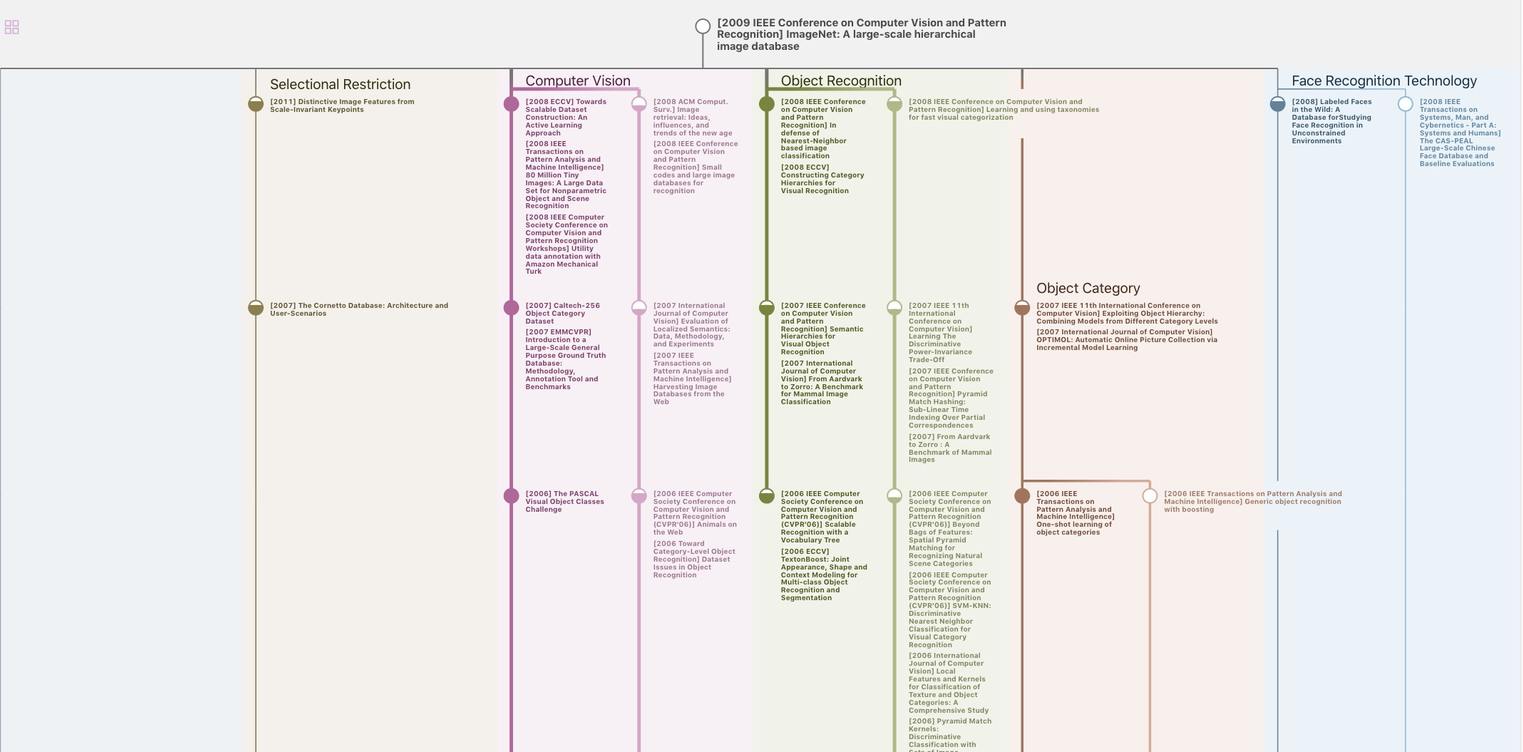
生成溯源树,研究论文发展脉络
Chat Paper
正在生成论文摘要