Ensembled Seizure Detection Based on Small Training Samples
IEEE TRANSACTIONS ON SIGNAL PROCESSING(2024)
摘要
This paper proposes an interpretable ensembled seizure detection procedure using electroencephalography (EEG) data, which integrates data driven features and clinical knowledge while being robust against artifacts interference. The procedure is built on the spatially constrained independent component analysis supplemented by a knowledge enhanced sparse representation of seizure waveforms to extract seizure intensity and waveform features. Additionally, a multiple change point detection algorithm is implemented to overcome EEG signal's non-stationarity and to facilitate temporal feature aggregation. The selected features are then fed into a random forest classifier for ensembled seizure detection. Compared with existing methods, the proposed procedure has the ability to identify seizure onset periods using only a small proportion of training samples. Empirical evaluations on publicly available datasets demonstrated satisfactory and robust performance of the proposed procedure.
更多查看译文
关键词
Change point detection,few-shot learning,expert system,independent component analysis
AI 理解论文
溯源树
样例
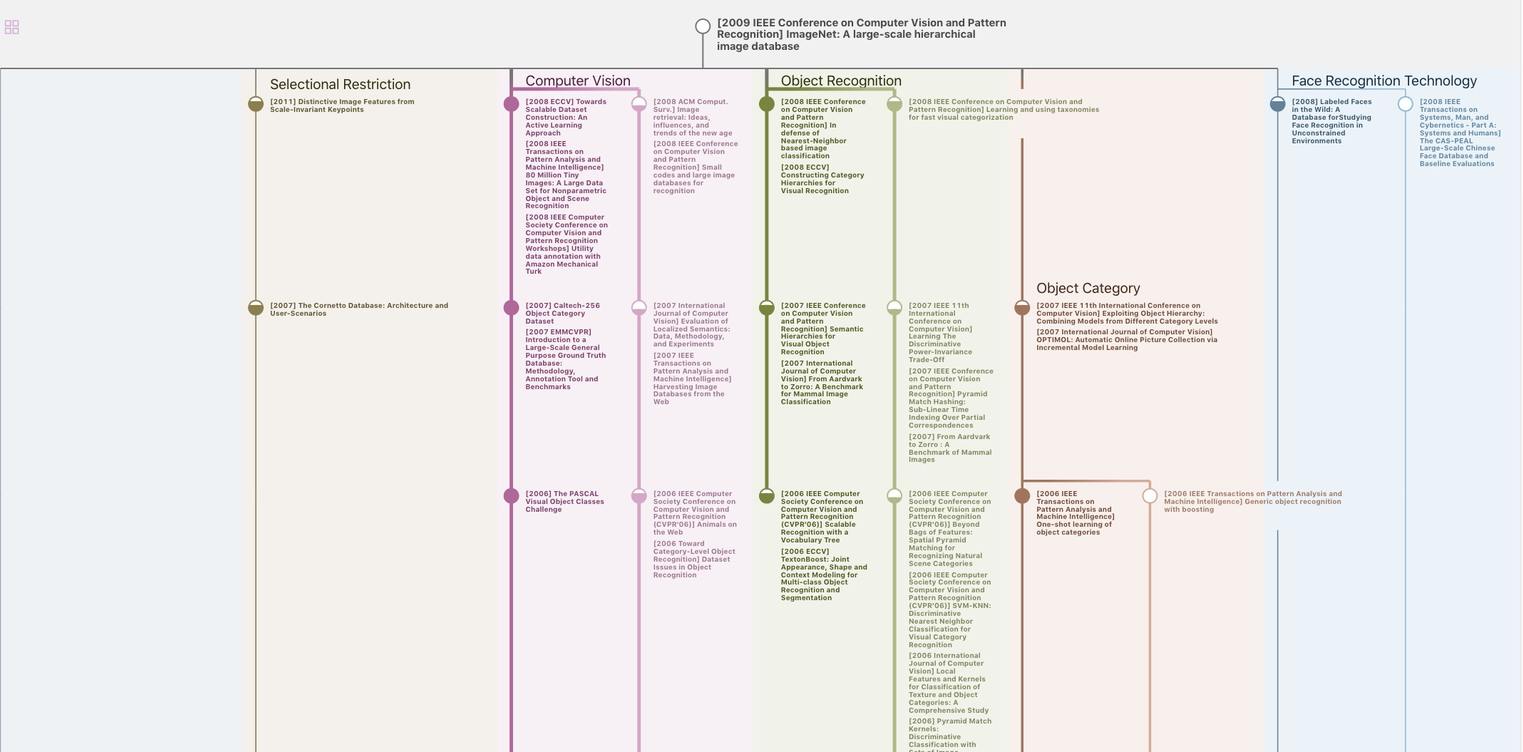
生成溯源树,研究论文发展脉络
Chat Paper
正在生成论文摘要