Expert-augmented machine learning to accelerate the discovery of copolymers for anion exchange membrane
JOURNAL OF MEMBRANE SCIENCE(2024)
摘要
We constructed an automated machine learning pipeline to screen the broad chemical space of candidate co-polymers for anion exchange membranes (AEMs). The pipeline utilizes a genetic algorithm integrated twelve machine learning algorithms, to screen copolymers that are potentially capable to improve AEM performance through the evolution of polymer hydrophobic and hydrophilic backbones and cation groups. An exhaustive data mining accumulated 749 experimentally reported AEMs, then the pipeline automatically generated over 172 million hypothetical copolymers, and 2519 potential candidates were screened out according to their predicted performance metrics including the OH- conductivity, the conductivity - dimensional stability trade-off coefficient, and the LUMO of cations in Pareto frontier set. Expert experience was adopted in the construction of robust regression and classification predictive models, and the importance of physical, chemical and topological fea-tures were illustrated from Shapley additive explanations (SHAP) analysis. We have released a standalone software that integrates these predictive models and the screened copolymer candidates with predicted AEM performance metrics at https://github.com/polySML/polySML-AEM. This study can facilitate the development of advanced AEMs through rational design of copolymers.
更多查看译文
关键词
Automated machine learning pipeline,Anion exchange membrane (AEM),Conductivity-Dimensional stability trade-off,High throughput screening,SHAP analysis
AI 理解论文
溯源树
样例
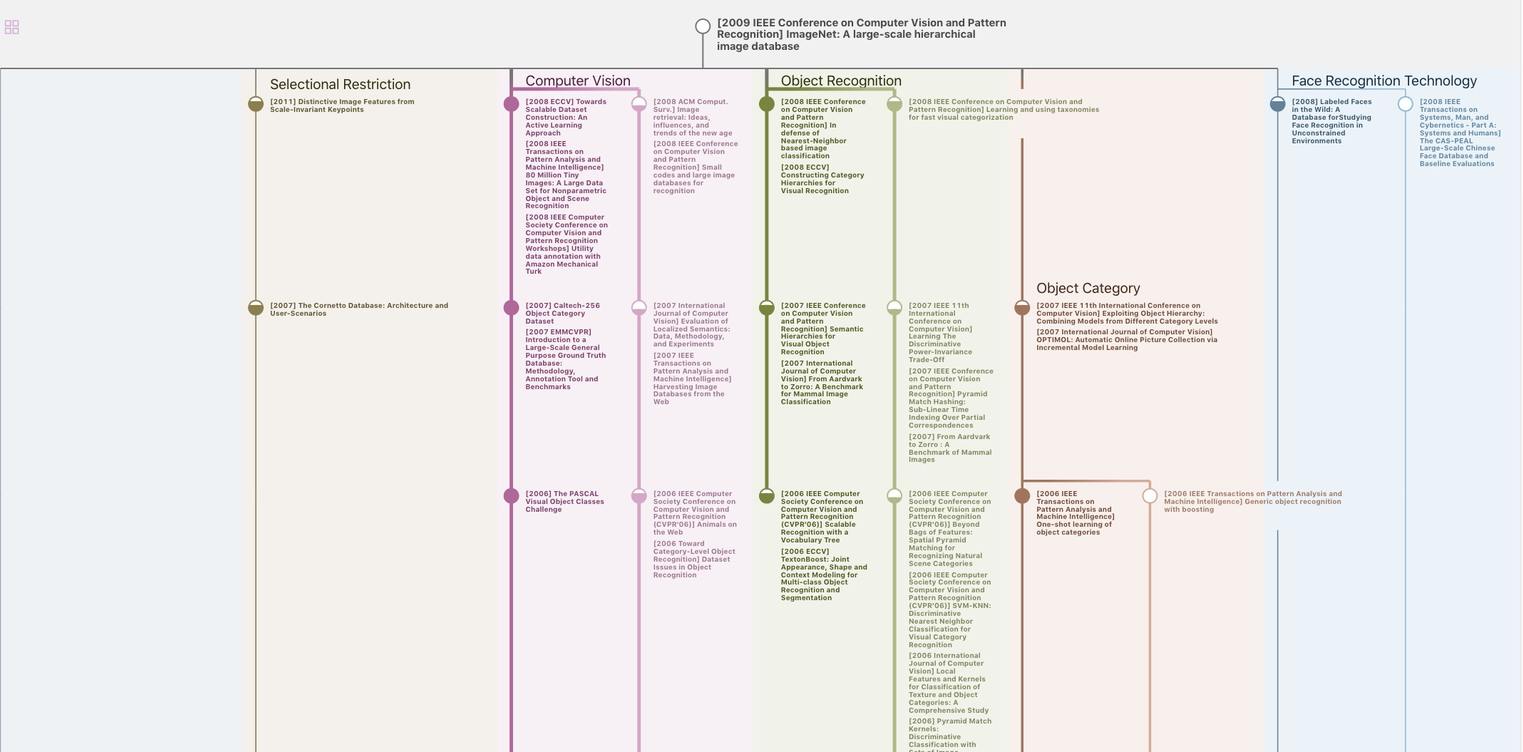
生成溯源树,研究论文发展脉络
Chat Paper
正在生成论文摘要