Object Localization Using Non-Euclidean Metrics.
2023 IEEE International Conference on Internet of Things and Intelligence Systems (IoTaIS)(2023)
摘要
In this paper, we proposed to use non-Euclidean statistical metrics to localize multiple 3D anatomical structures by estimating the object's position, orientation, and size in med-ical images. Precise orientation estimation is extremely important especially for model-based image segmentation algorithms because even a very small change in shape model orientation can lead to an inaccurate localization and segmentation. We statistically evaluated accuracy of orientation estimation using various metrics: Euclidean, Mean Hermitian, Log-Euclidean, Root-Euclidean, Cholesky decomposition, and Procrustes Size-and-Shape. Experimental results showed that non-Euclidean metrics, particularly Mean Hermitian and Cholesky decomposition, provided more accurate estimates than Euclidean metrics. We presented the effectiveness of the proposed method using abdominal and hand computed tomography (CT) images and magnetic resonance (MR) images of the foot. We obtained 3-to-6 times smaller RMSE (root mean square error) object localization errors with Non-Euclidean metrics compared to Euclidean metric.
更多查看译文
关键词
Object Localization,Segmentation,Pose Esti-mation,non-Euclidean metrics,Organ Detection
AI 理解论文
溯源树
样例
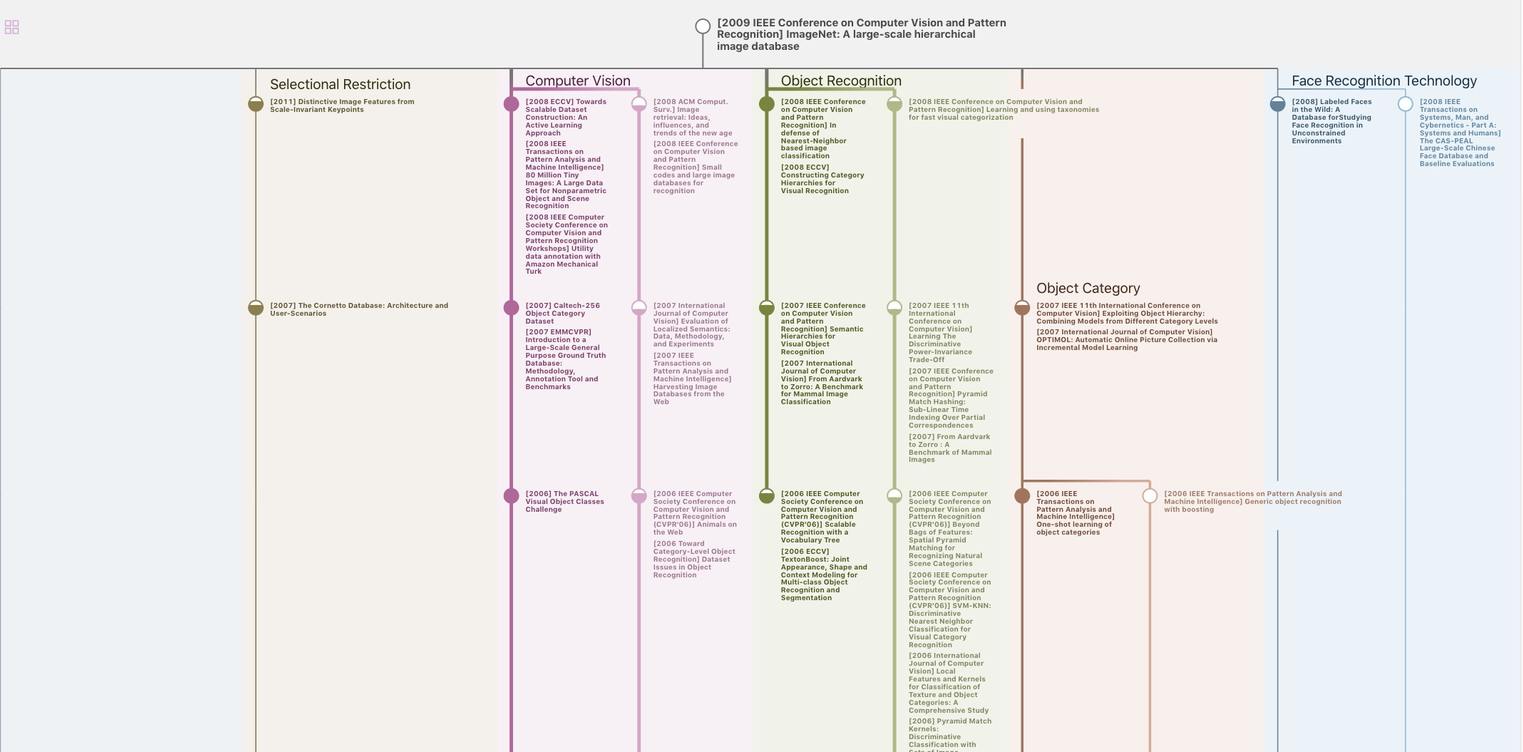
生成溯源树,研究论文发展脉络
Chat Paper
正在生成论文摘要