A Vehicle Pass Detection Method Based on YOLOv4 Improvements
2023 8th International Conference on Intelligent Informatics and Biomedical Sciences (ICIIBMS)(2023)
摘要
Vehicle pass detection plays a crucial role in various specialized scenarios in modern life. However, the existing models for vehicle pass detection have limitations in terms of their detection performance due to the diverse and complex nature of vehicle passes. Therefore, accurately detecting vehicle passes has become an urgent problem that needs to be addressed. In this article, we propose further improvements to the classic YOLOv4 model to enhance its detection capabilities. These improvements include abandoning the CSPDarket53 network as the backbone and using ConvNeXt-S network for feature extraction, substituting the CIoU loss with the SIoU loss, and incorporating the Global Attention Mechanism into YOLOv4. Experimental results on local datasets demonstrate that the proposed model surpasses other models such as Faster R-CNN, the classic YOLOv4, and YOLOv3 in terms of mean average precision (mAP). Specifically, the improved YOLOv4 model achieved an increase of 5.89%, 7.19%, and 2% in mAP compared to Faster R-CNN, YOLOv3, and the classic YOLOv4 models, respectively.
更多查看译文
关键词
vehicle pass detection,YOLOv4,ConvNeXt-S,GAM,SIoU
AI 理解论文
溯源树
样例
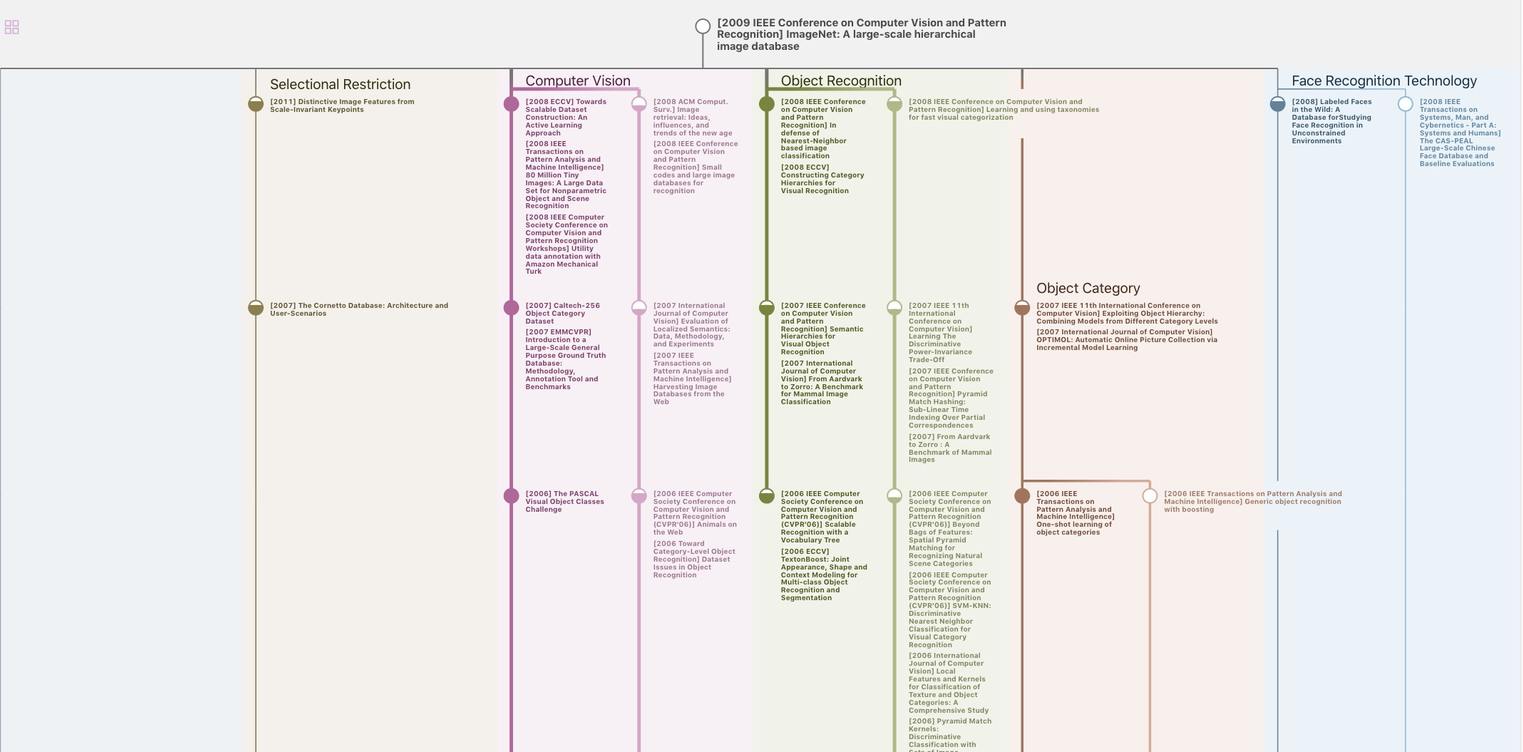
生成溯源树,研究论文发展脉络
Chat Paper
正在生成论文摘要