Rule-Based Detection of Anomalous Patterns in Device Behavior for Explainable IoT Security
IEEE TRANSACTIONS ON SERVICES COMPUTING(2023)
摘要
The behavioral analysis of smart devices plays a key role in enforcing security for IoT environments. In particular, anomalous patterns can be targeted in the behavior of smart devices as potential IoT cybersecurity threats. In this article, an explainable machine-learning approach is proposed for dealing with behavioral anomalies. Essentially, a rule-based classifier is inferred from the observed behavior of smart devices, to detect and explain patterns of behavioral anomalies. Predictive association modeling is adopted in the formulation of the classifier, to achieve superior effectiveness in detecting behavioral patterns and ensuring clear explanations of both these latter and their classifications. Moreover, the specifically-conceived design of the classifier reduces the number of tunable parameters to one. An extensive empirical evaluation is comparatively carried out on real-world benchmark data. The experimental results reveal the effectiveness, robustness, and scalability of the proposed approach.
更多查看译文
关键词
Anomaly detection,behavioral patterns,explainable machine learning,Internet of Things
AI 理解论文
溯源树
样例
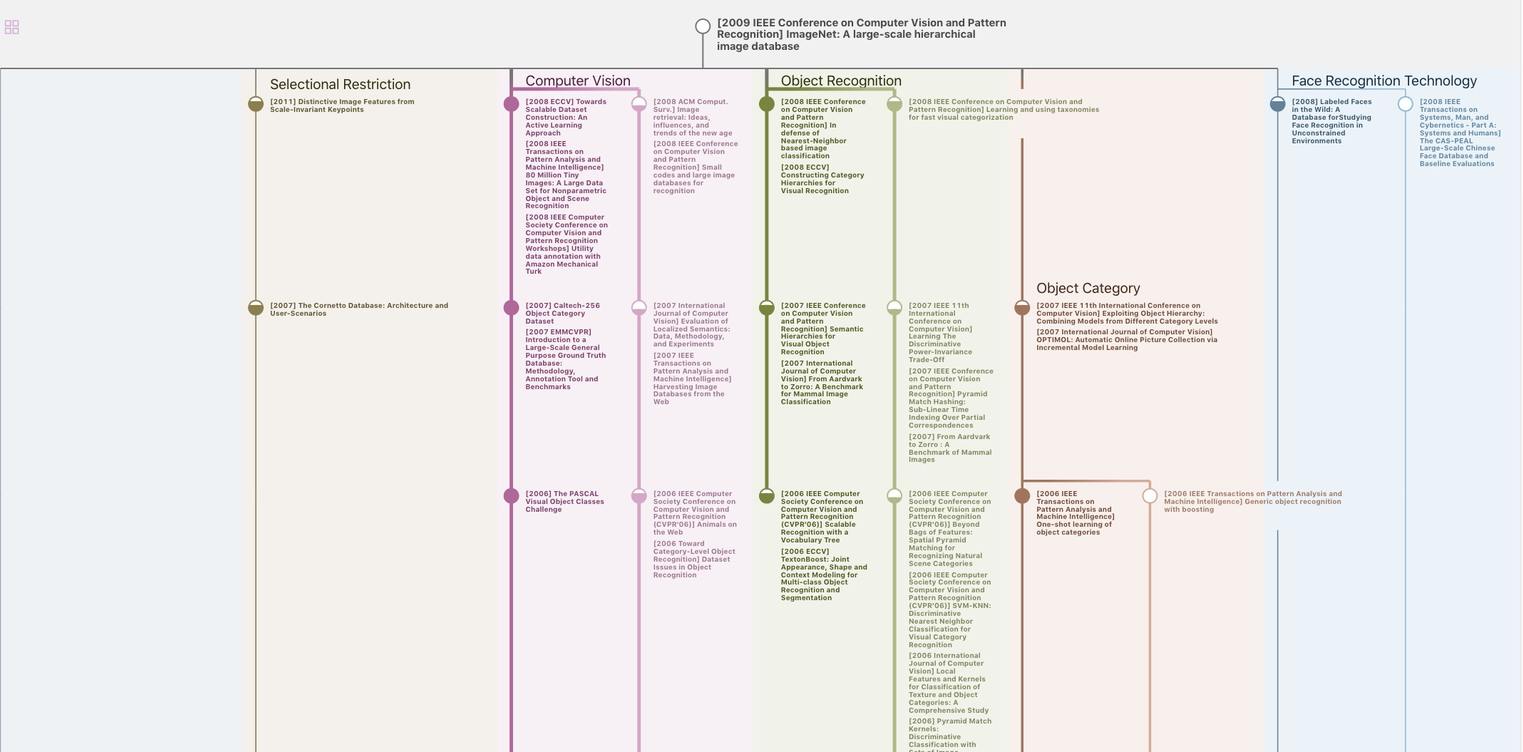
生成溯源树,研究论文发展脉络
Chat Paper
正在生成论文摘要