Prediction of regional carbon emissions using deep learning and mathematical-statistical model
JOURNAL OF AMBIENT INTELLIGENCE AND SMART ENVIRONMENTS(2023)
Abstract
Detecting carbon emissions is the key to carbon peaking and carbon neutrality goals. Existing research has focused on utilizing data-driven method to study carbon emissions off a single object. This study proposes a regional carbon emissions prediction method. The area objects are divided into dynamic objects for vehicles and static objects for buildings. For the dynamic object, carbon emissions is modeled using the vehicle location provided by the BeiDou satellite navigation system (BDS). For the static object, the neural network R3det (rotation remote sensing target detection) is used to identify the buildings in remote sensing images, and then the trained ARIMA time series model is used to predict the carbon emissions. The model is tested in an industrial park in Tangshan, Hebei Province in China. The result of the regional three-dimensional emission map shows that the method provided a novel and feasible idea for carbon emissions prediction.
MoreTranslated text
Key words
Deep learning,ARIMA time series model,remote sensing,BDS,regional carbon emissions
AI Read Science
Must-Reading Tree
Example
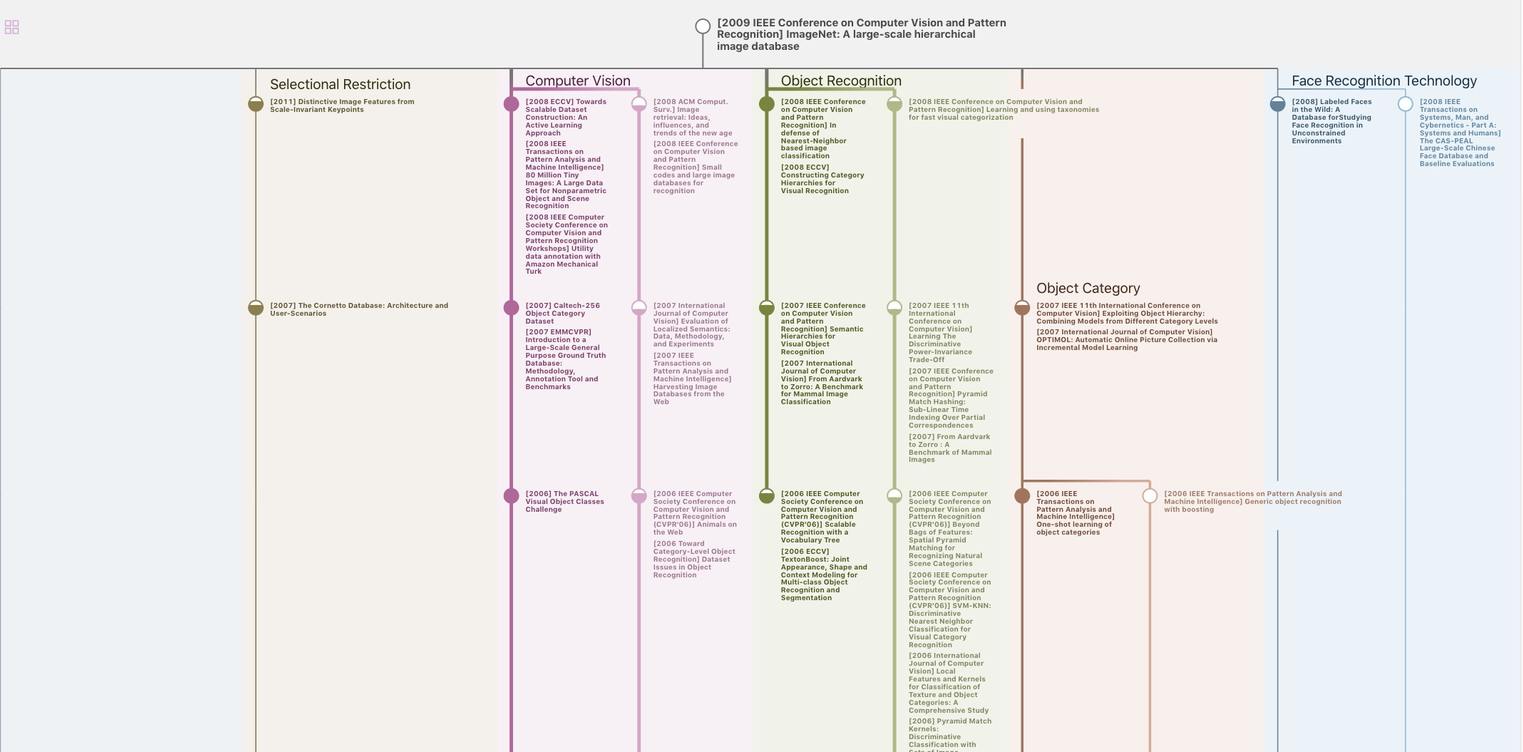
Generate MRT to find the research sequence of this paper
Chat Paper
Summary is being generated by the instructions you defined