Multi-organ spatiotemporal information aware model for sepsis mortality prediction
ARTIFICIAL INTELLIGENCE IN MEDICINE(2024)
摘要
Background: Sepsis is a syndrome involving multi-organ dysfunction, and the mortality in sepsis patients correlates with the number of lesioned organs. Precise prognosis models play a pivotal role in enabling healthcare practitioners to administer timely and accurate interventions for sepsis, thereby augmenting patient outcomes. Nevertheless, the majority of available models consider the overall physiological attributes of patients, overlooking the asynchronous spatiotemporal interactions among multiple organ systems. These constraints hinder a full application of such models, particularly when dealing with limited clinical data. To surmount these challenges, a comprehensive model, denoted as recurrent Graph Attention Network-multi Gated Recurrent Unit (rGAT-mGRU), was proposed. Taking into account the intricate spatiotemporal interactions among multiple organ systems, the model predicted in-hospital mortality of sepsis using data collected within the 48-hour period post-diagnosis. Material and methods: Multiple parallel GRU sub-models were formulated to investigate the temporal physiological variations of single organ systems. Meanwhile, a GAT structure featuring a memory unit was constructed to capture spatiotemporal connections among multi-organ systems. Additionally, an attention-injection mechanism was employed to govern the data flowing within the network pertaining to multi-organ systems. The proposed model underwent training and testing using a dataset of 10,181 sepsis cases extracted from the Medical Information Mart for Intensive Care III (MIMIC-III) database. To evaluate the model's superiority, it was compared with the existing common baseline models. Furthermore, ablation experiments were designed to elucidate the rationale and robustness of the proposed model. Results: Compared with the baseline models for predicting mortality of sepsis, the rGAT-mGRU model demonstrated the largest area under the receiver operating characteristic curve (AUROC) of 0.8777 +/- 0.0039 and the maximum area under the precision-recall curve (AUPRC) of 0.5818 +/- 0.0071, with sensitivity of 0.8358 +/- 0.0302 and specificity of 0.7727 +/- 0.0229, respectively. The proposed model was capable of delineating the varying contribution of the involved organ systems at distinct moments, as specifically illustrated by the attention weights. Furthermore, it exhibited consistent performance even in the face of limited clinical data. Conclusion: The rGAT-mGRU model has the potential to indicate sepsis prognosis by extracting the dynamic spatiotemporal interplay information inherent in multi-organ systems during critical diseases, thereby providing clinicians with auxiliary decision-making support.
更多查看译文
关键词
Sepsis prognosis,Spatiotemporal information,Multi-organ systems,Graph attention network
AI 理解论文
溯源树
样例
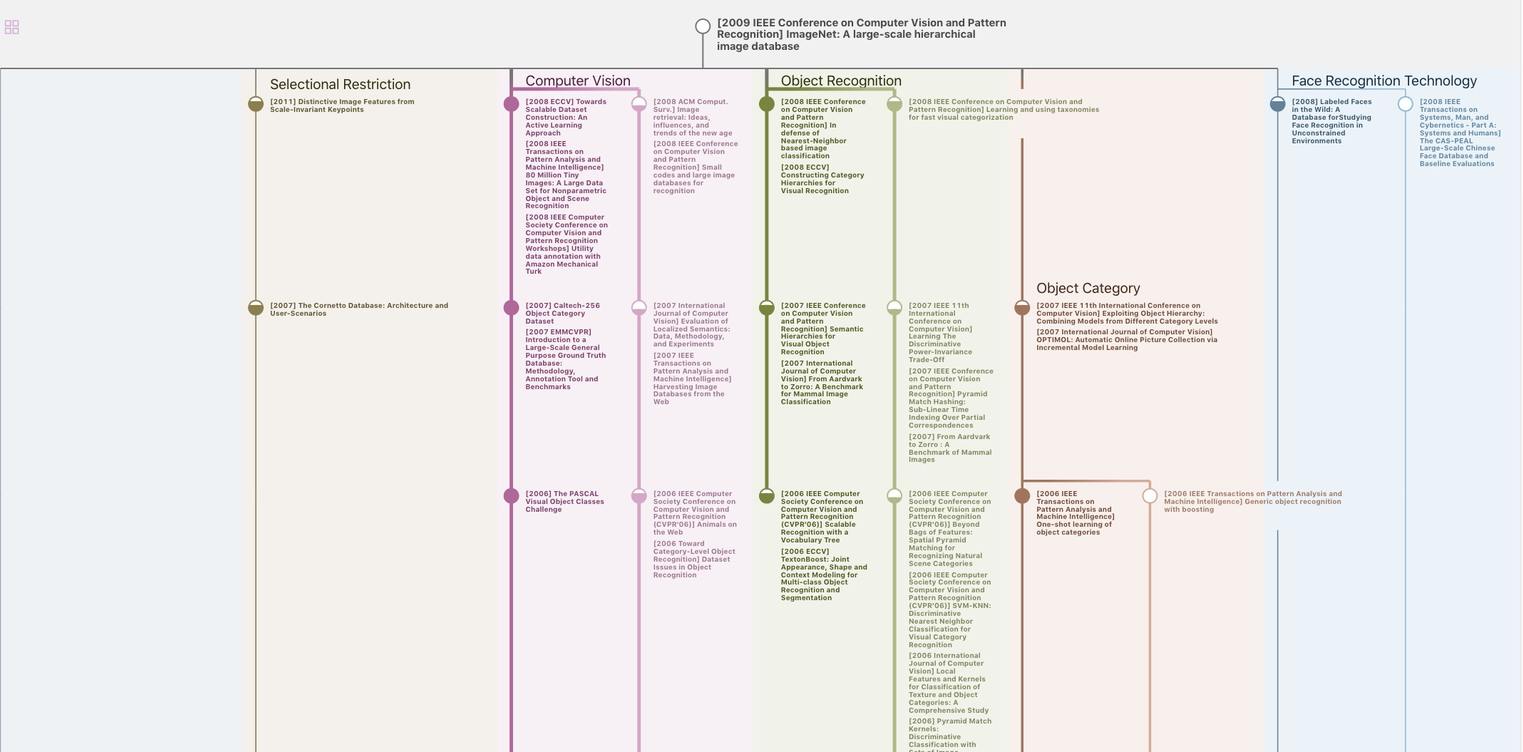
生成溯源树,研究论文发展脉络
Chat Paper
正在生成论文摘要