Information and disinformation in hydrological data across space: The case of streamflow predictions using machine learning
JOURNAL OF HYDROLOGY-REGIONAL STUDIES(2024)
摘要
Study region: A total of 461 watersheds across the USA. Study Focus: This study aimed to assess the usefulness of data from donor watersheds to predict streamflow in parent watersheds. For this purpose, Long Short-Term Memory network (LSTM) was used as an information extraction algorithm because of its state-of-the art performance in terms of predicting streamflow. Out of the 461 watersheds used in this study, 57 watersheds were selected as the parent watersheds. The quantity 'optimal number of donor watersheds (NT)' and 'changes in NSE' were used as a practical measures of information content in donor watersheds. Several LSTM models were developed by using the data from different number of donor watersheds, varying from 1 to 128, to train the models. New Hydrological Insights for the Region: Increasing the number of donor watersheds beyond some optimal NT resulted in a statistically insignificant and, in several cases, hydrologically irrelevant gain in accuracy.In some cases, the Nash-Sutcliff Efficiency (NSE) slightly decreased when NT was increased beyond the optimal value. In several watersheds using a large number of donor watersheds might result in excessively rainfall sensitive LSTM models. Further, data from donor watersheds do not seem to provide information for low flow predictions. Thus, this study offers a nuanced and sobering perspective on the usefulness of data from multiple donor watersheds in terms of streamflow predictions in any given watershed.
更多查看译文
关键词
Hydrological information,Streamflow,Rainfall-runoff modeling,Long short-term memory network (LSTM),Machine learning
AI 理解论文
溯源树
样例
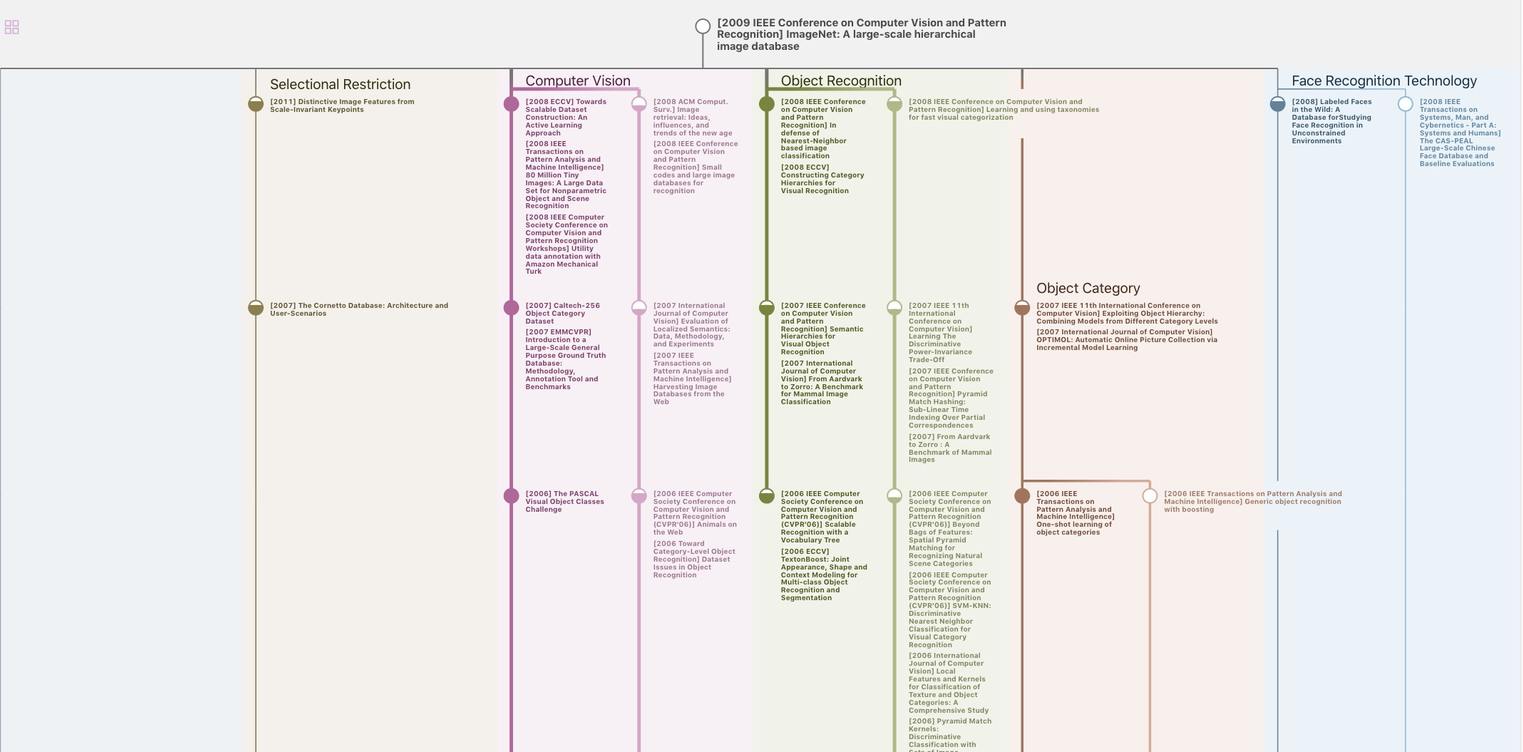
生成溯源树,研究论文发展脉络
Chat Paper
正在生成论文摘要