Deep Style Transfer to Deal with the Domain Shift Problem on Spheroid Segmentation
Neurocomputing(2024)
摘要
Spheroids are the most widely used 3D models for studying the effects of different micro-environmental characteristics on tumor behaviour, and for testing different preclinical and clinical treatments. Image analysis of spheroids is crucial to understand the formation and behaviour of such tumors, but when performed manually is a time-consuming task. Deep convolutional models can help in the analysis of spheroid images since they provide accurate results for the segmentation of tumors, a task that is necessary to further study features of spheroids. However, the images from tumor spheroids greatly vary depending on the experimental conditions, and also on the equipment (microscopes) and conditions (focus and magnification) employed to capture the images. This issue, known as domain shift, hinders the applicability of deep learning models for spheroid segmentation. In this work, we address this problem by studying style transfer methods based on both convolutional neural networks and generative adversarial networks to transfer the style from the images used for training segmentation models to the images from a new domain. This approach successfully handled the domain shift problem in the context of spheroid segmentation for 6 different deep learning models. Finally, we developed a high-level API that facilitates the of our approach to other contexts where the domain-shift problem might occur.
更多查看译文
关键词
Domain adaption,Style transfer,GAN,Segmentation,Spheroids
AI 理解论文
溯源树
样例
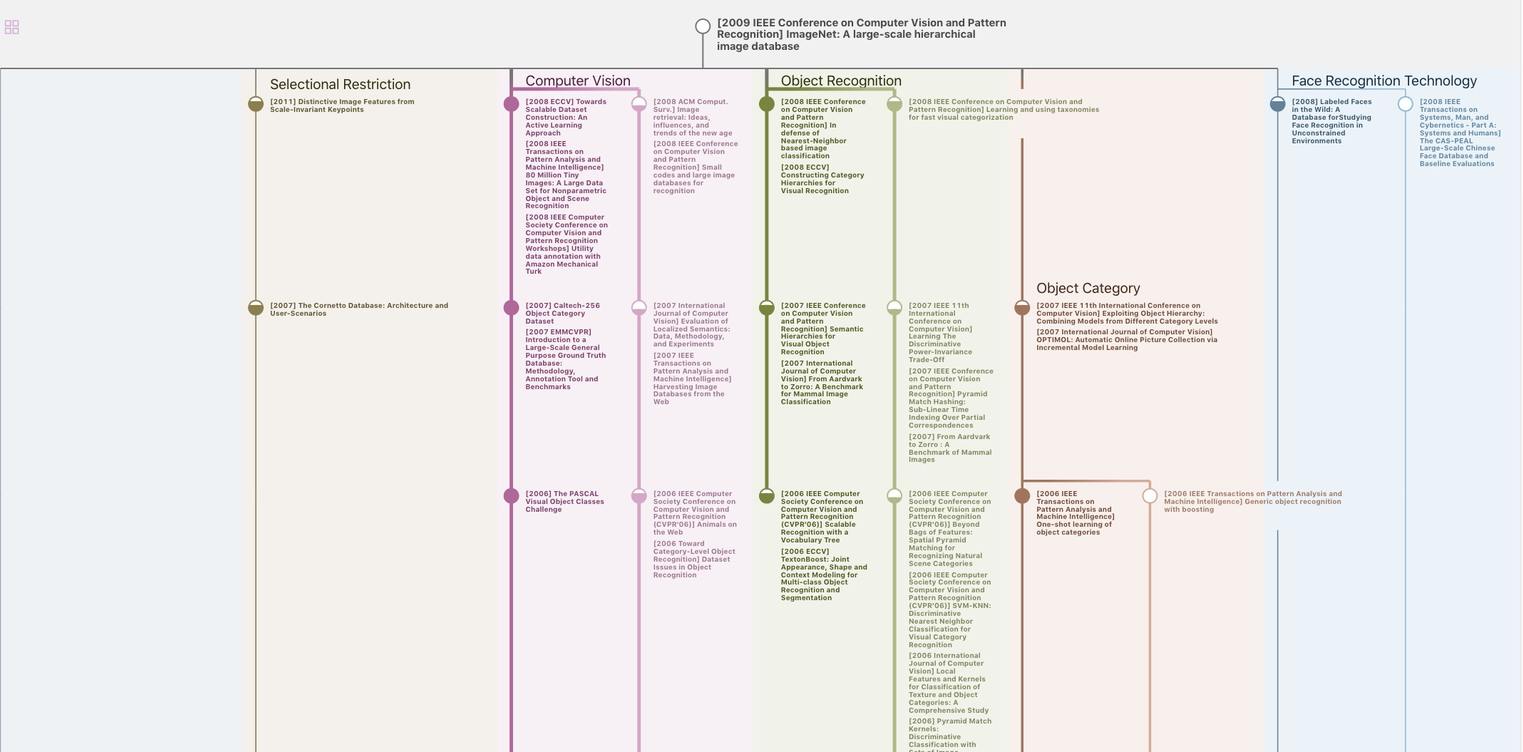
生成溯源树,研究论文发展脉络
Chat Paper
正在生成论文摘要