Impact force localization and reconstruction via gated temporal convolutional network
AEROSPACE SCIENCE AND TECHNOLOGY(2024)
摘要
Techniques for identifying impact forces are crucial for improving the operational reliability and safety of aerospace composite structures. To address the challenges of theoretical modeling that arise from the complexity of composite structures, we propose a Gated Temporal Convolutional Network (GTCN) method. This method establishes inverse mapping relationships between structural vibration responses and impact forces. The GTCN increases the receptive field through dilated convolution, concurrently employing gating mechanisms to extract pivotal features from input signals in the vertical direction. Simulations and experiments are conducted to robustly validate the efficacy and applicability of the proposed impact force identification method. The simulation results demonstrate that the GTCN method is capable of obtaining robust impact force localization and reconstruction results, even amidst conditions of elevated noise levels. The average localization error of the GTCN is kept within 20 mm even at a noise level of 5 dB. Meanwhile, the GTCN method achieves satisfactory identification results for different types of impact forces (Gaussian, semi-cosine, and sawtooth), with an average localization accuracy above 95%. The applicability of the GTCN method to real-world structure is verified in the experiments. The average relative error of the reconstructed forces by GTCN is less than 15%. GTCN achieves stable identification of impact forces when the number of sensors is larger than 2. In both simulations and experiments, the GTCN performs better than Temporal Convolutional Network (TCN) and Convolutional Neural Network (CNN) with higher identification accuracy. The proposed method demonstrates considerable potential for application in the health monitoring of aircraft composite structures.
更多查看译文
关键词
Impact force reconstruction,Impact force localization,Gated temporal convolutional network,Composite structures,Structural health monitoring
AI 理解论文
溯源树
样例
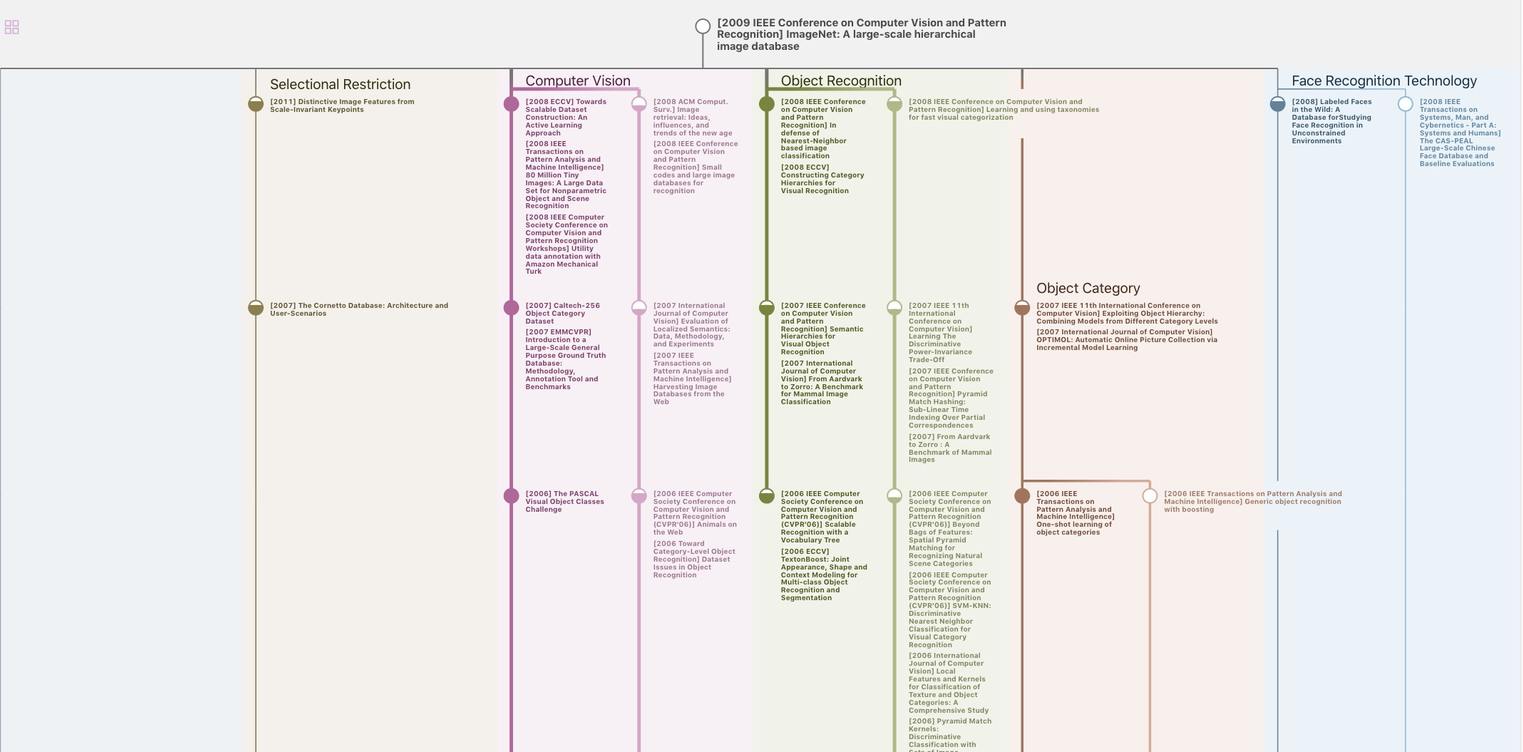
生成溯源树,研究论文发展脉络
Chat Paper
正在生成论文摘要