Joint analysis and segmentation of time-varying data with outliers
DIGITAL SIGNAL PROCESSING(2024)
摘要
Principal-Component Analysis (PCA) is a fundamental tool in data science and machine learning, used for compressing, analyzing, visualizing, and processing large datasets. At the same time, temporal segmentation is important for coherent component analysis of big data collections generated by time-varying distributions. However, both segmentation and PCA can be critically affected and misled by corrupted points that often exist in big data collections. To address these issues, we propose a novel and robust method for joint segmentation and principal-component analysis of time-varying data, based on L1-norm formulations. Our proposed method estimates robust L1-norm principal components (L1-PCs) over different temporal horizons and combines them to perform outlier detection, data segmentation, and subspace estimation. Numerical studies on real-world data, including videos and smartphone-sensed human body motion measurements, corroborate the merits of the proposed method in terms of segmentation, PCA, and outlier detection/removal.
更多查看译文
关键词
Data segmentation,L1-norm principal-component analysis,Time series,Outliers,Subspace clustering
AI 理解论文
溯源树
样例
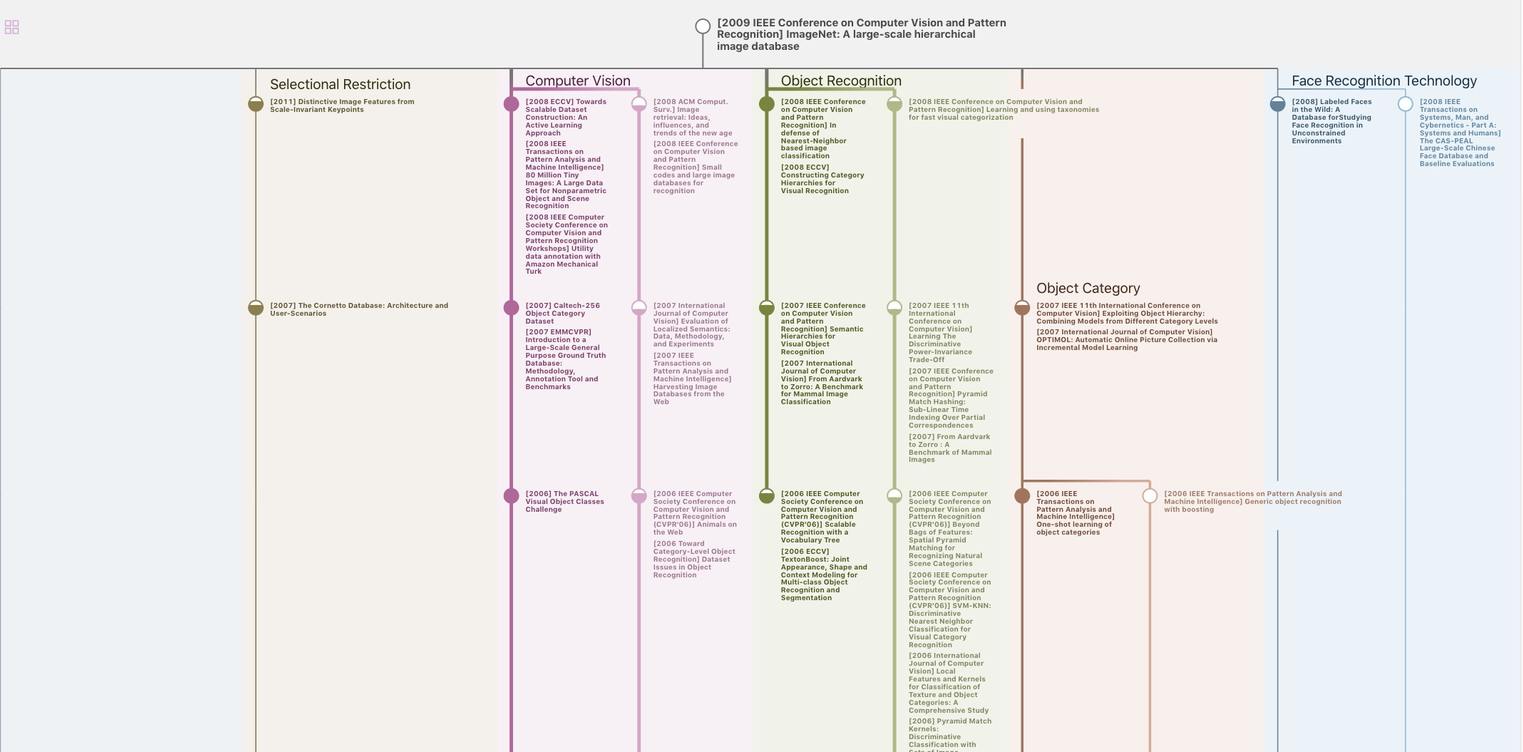
生成溯源树,研究论文发展脉络
Chat Paper
正在生成论文摘要