Global Joint Information Extraction Convolution Neural Network for Parkinson’s Disease Diagnosis
EXPERT SYSTEMS WITH APPLICATIONS(2024)
关键词
Parkinson’s diseases(PD),Gait features,Imageization,Deep learning
AI 理解论文
溯源树
样例
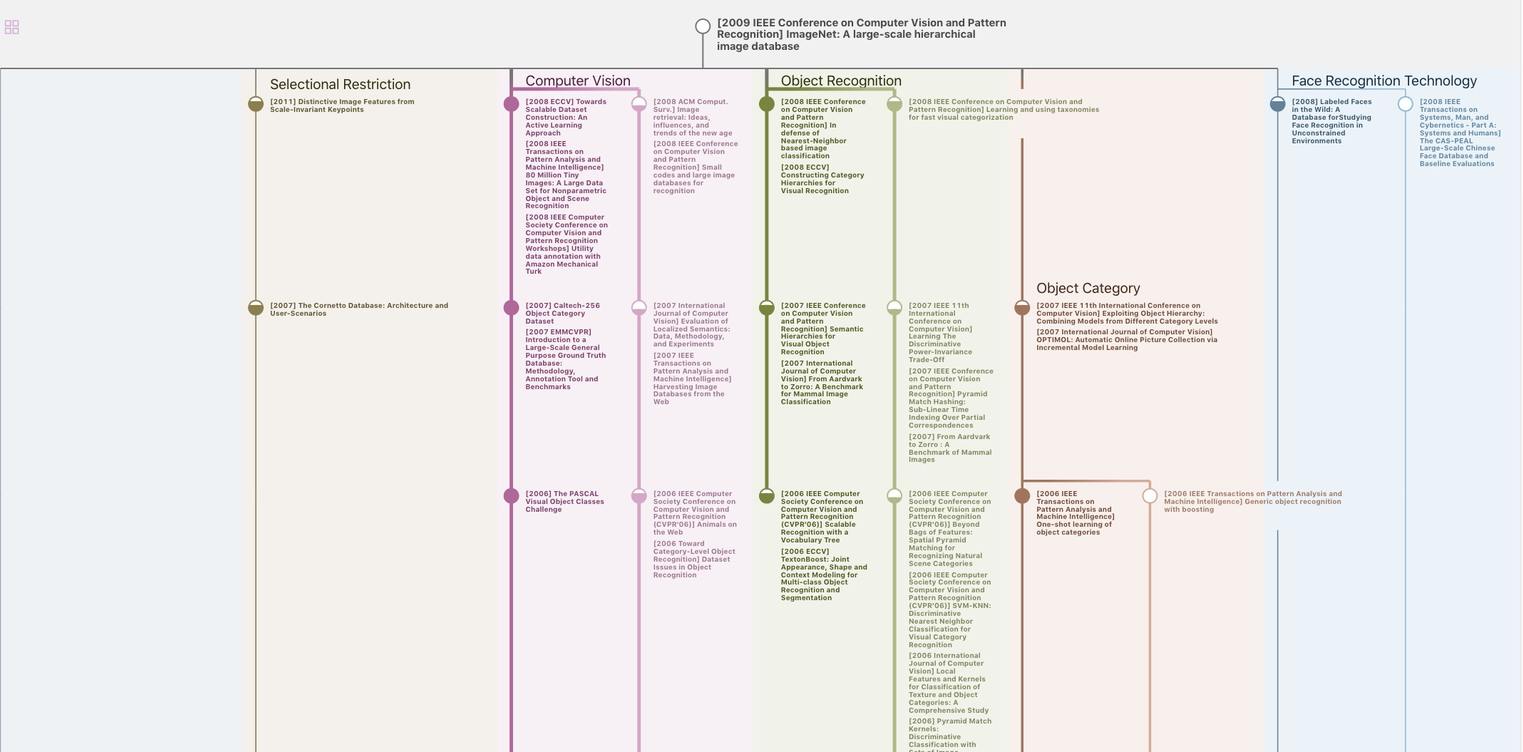
生成溯源树,研究论文发展脉络
Chat Paper
正在生成论文摘要