Incremental reinforcement learning and optimal output regulation under unmeasurable disturbances
Automatica(2024)
摘要
In this paper, we propose novel data-driven optimal dynamic controller design frameworks, via both state-feedback and output-feedback, for solving optimal output regulation problems of linear discrete-time systems subject to unknown dynamics and unmeasurable disturbances using reinforcement learning (RL). Fundamentally different from existing research on optimal output regulation problems and RL, the proposed procedures can determine both the optimal control gain and the optimal dynamic compensator simultaneously instead of presetting a non-optimal dynamic compensator. Moreover, we present incremental dataset-based RL algorithms to learn the optimal dynamic controllers that do not require the measurements of the external disturbance and the exostate during learning, which is of great practical importance. Besides, we show that the proposed incremental dataset-based learning methods are more robust to a class of measurement noises with arbitrary magnitudes than routine RL algorithms. Comprehensive simulation results validate the efficacy of our methodologies.
更多查看译文
关键词
Reinforcement learning,Optimal control,Output regulation,Incremental dataset
AI 理解论文
溯源树
样例
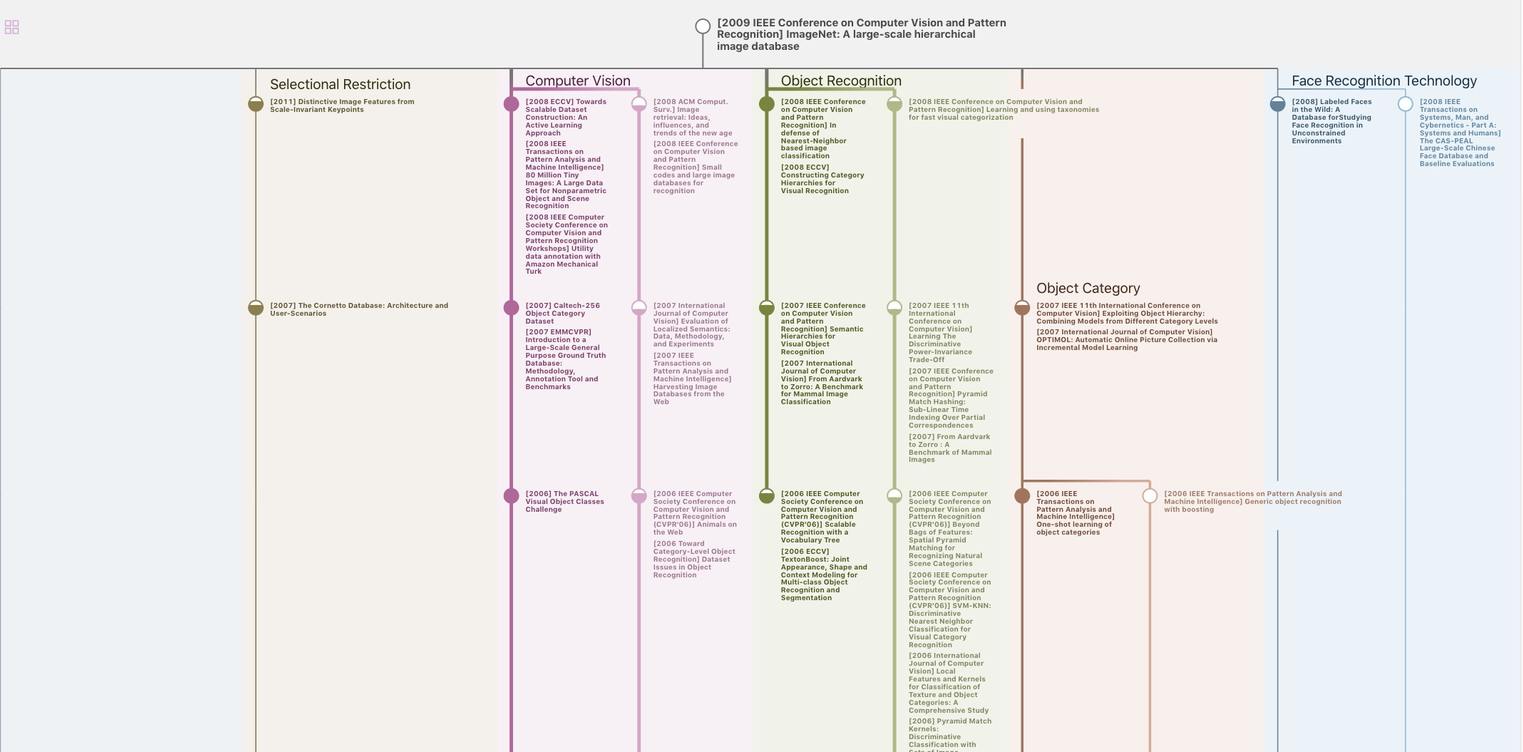
生成溯源树,研究论文发展脉络
Chat Paper
正在生成论文摘要