Model-Assisted Multi-source Fusion Hypergraph Convolutional Neural Networks for intelligent few-shot fault diagnosis to Electro-Hydrostatic Actuator
INFORMATION FUSION(2024)
摘要
Electro-Hydrostatic Actuator (EHA) is a critical electro-hydraulic actuator system widely used in aerospace equipment. To ensure its normal operation, the intelligent fault diagnosis of the EHA system has gained increasing attention. However, the EHA exhibits strong nonlinearity, high structural complexity, and difficulty obtaining fault samples. A Model-Assisted Multi-source Fusion Hypergraph Convolutional Neural Network (MAMF-HGCN) is proposed to address the few-shot intelligent fault diagnosis of EHA. Specifically, the fault data obtained from the hydraulic simulation model is used to establish the relationship among each channel signal. This assists in constructing a hypergraph structure for actual multi-source fault data under limited samples. Each node in the hypergraph employs message transmission to fuse signals from different channels. Subsequently, the hypergraph data are input into the constructed hypergraph convolutional neural network to perform fault classification. Finally, validating the EHA hydraulic system test rig from Nanjing University of Science and Technology illustrates the method's effectiveness in diagnosing hydraulic system problems under limited fault samples.
更多查看译文
关键词
Electro-Hydrostatic Actuator (EHA),Hypergraph,Data fusion,Model assist,Intelligent fault diagnosis
AI 理解论文
溯源树
样例
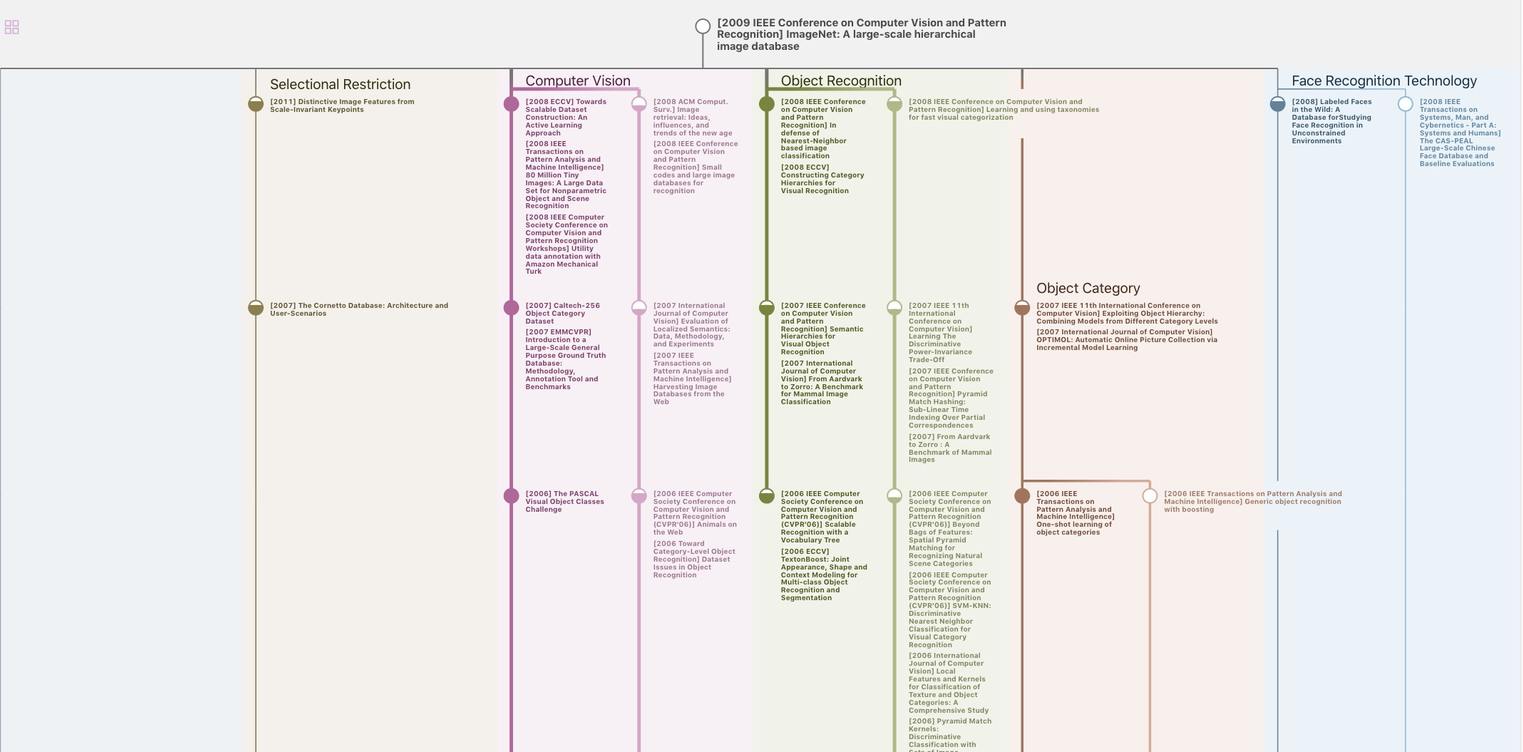
生成溯源树,研究论文发展脉络
Chat Paper
正在生成论文摘要