CrystalMind: A surrogate model for predicting 3D models with recrystallization in open-die hot forging including an optimization framework
MECHANICS OF MATERIALS(2024)
摘要
This work introduces CrystalMind, a surrogate model developed to accurately reconstruct 3D models along with recrystallization induced by the open-die hot forging process. CrystalMind employs two types of data inputs, a 3D Model depicting pre-stroke workpiece geometry, and a forging vector outlining the proposed forging strategy. This forging strategy consists of bite infeed, bite offset, number of offsets and initial position. Moreover, a machine learning architecture with two different units, MLP-based (Multi-Layer Perceptron) and PointNET++-based, was implemented and compared, demonstrating similar performance in terms of recrystallization and deformation errors. However, MLP proved 36 times faster in computational time reaching an average computational time of 5 ms pro run. Furthermore, CrystalMind adheres to the volume conservation condition and limits recrystallization error to less than 2% and deformation error to less than 0.1 mm or 0.9% for the test data. Finally, CrystalMind is employed in conjunction with an optimization algorithm, leading to remarkable enhancements in time efficiency. The optimization framework can effectively optimize a forging vector(s) for one, two, or three strokes. For instance, in the case of three strokes, CrystalMind enables the optimization process to be completed within an average of 7 min, a stark contrast to the approximately 2.3 years required when utilizing FEM simulation. Overall, CrystalMind provides fast and accurate predictions of deformed 3D models with corresponding recrystallization per point caused by a forging process, something not to be found in the state of the art so far.
更多查看译文
关键词
Machine learning,Forging,FEM,MLP,PointNET plus plus,Surrogate model,Dual annealing
AI 理解论文
溯源树
样例
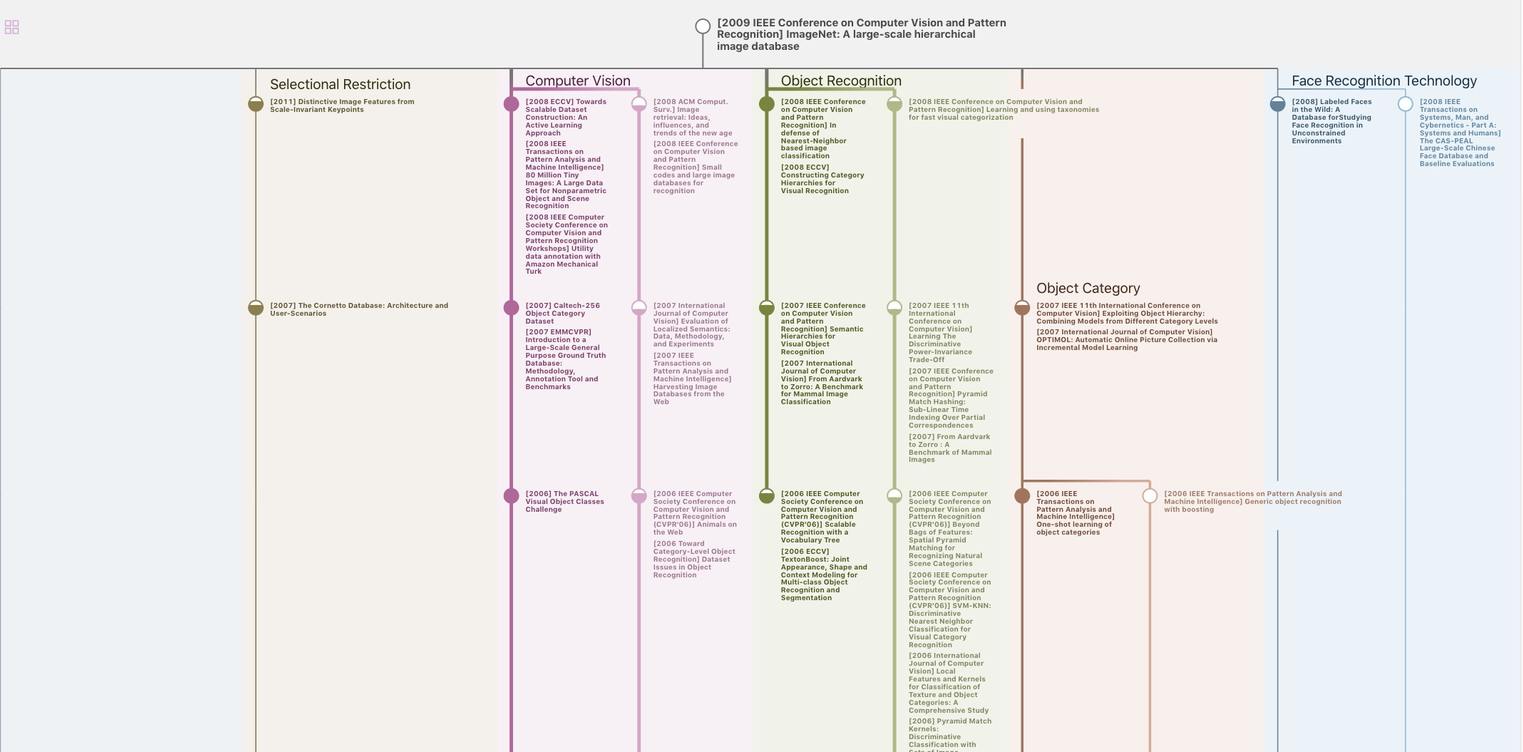
生成溯源树,研究论文发展脉络
Chat Paper
正在生成论文摘要