Probabilistic Local Equivalence Certification for Robustness Evaluation
ADVANCES IN VISUAL COMPUTING, ISVC 2023, PT I(2023)
摘要
To evaluate the robustness of non-classifier models, we propose probabilistic local equivalence, based on the notion of randomized smoothing, as a way to quantitatively evaluate the robustness of an arbitrary function. For a given function f, probabilistic local equivalence evaluates whether, when sampling a normally-distributed point x' in a neighborhood of a point x, there is a probability > 0.5 that f(x') is equivalent to f(x), according to a user-defined notion of equivalence. We use probabilistic local equivalence to evaluate the effect of data augmentation methods for improving robustness, including adversarial training, on a model's performance. We also use probabilistic local equivalence to evaluate the effect on robustness of model architecture, number of parameters, pre-training, quantization, and other model properties.
更多查看译文
关键词
Computer Vision,Robustness,Evaluation
AI 理解论文
溯源树
样例
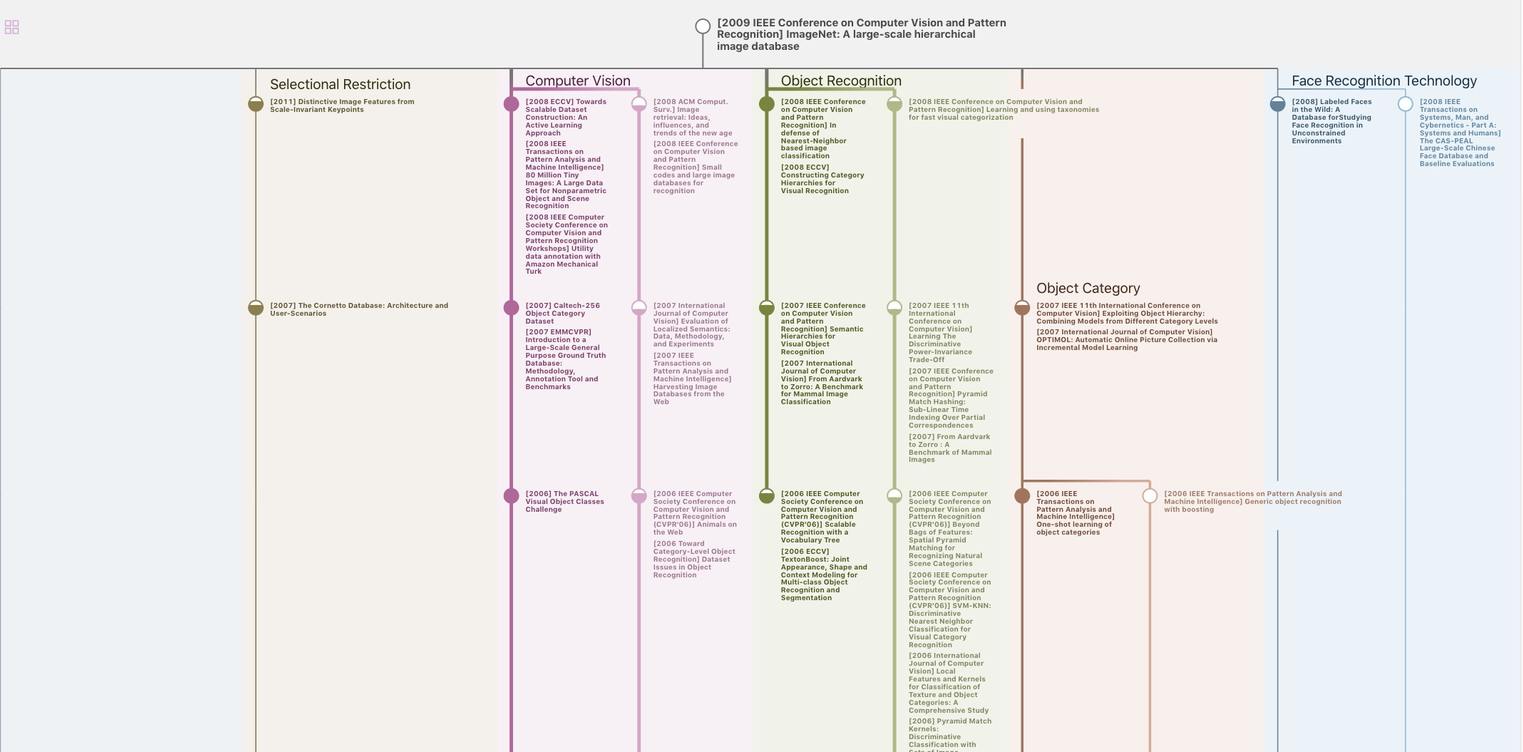
生成溯源树,研究论文发展脉络
Chat Paper
正在生成论文摘要