Cambricon-R: A Fully Fused Accelerator for Real-Time Learning of Neural Scene Representation
56TH IEEE/ACM INTERNATIONAL SYMPOSIUM ON MICROARCHITECTURE, MICRO 2023(2023)
摘要
Neural scene representation (NSR) initiates a new methodology of encoding a 3D scene with neural networks by learning from dozens of photos taken from different camera positions. NSR not only achieves significant improvement in the quality of novel view synthesis and 3D reconstruction but also reduces the camera cost from the expensive laser cameras to the cheap color cameras on the shelf. However, performing 3D scene encoding using NSR is far from real-time due to the extremely low hardware utilization (only 0.22% utilization of hardware peak performance), which greatly limits its applications in real-time AR/VR interactions In this paper, we propose Cambricon-R, a fully fused on-chip processing architecture for real-time NSR learning. Initially, by performing a thorough characterization of the computing model of NSR on a GPU, we find that the extremely low hardware utilization is mainly caused by the fragmentary stages and heavy irregular memory accesses. To address these issues, we propose Cambricon-R architecture with a novel fully-fused ray-based execution model to eliminate the computing and memory inefficiencies for real-time NSR learning. Concretely, Cambricon-R features a ray-level fused architecture that not only eliminates the intermediate memory traffics but also leverages the point sparsity in scenes to eliminate unnecessary computations. Additionally, a high throughput on-chip memory system based on Auto-Interpolation Bank Array (AIBA) is proposed to efficiently handle a large volume of irregular memory accesses. We evaluate Cambricon-R on 12 commonly-used datasets with the state-of-the-art representative algorithm, instant-ngp. The result shows that Cambricon-R achieves PE utilization of 67.3%, on average. Compared to the state-of-the-art solution on A100 GPU, Cambricon-R achieves 373.8x speedup and 256.6x energy saving, on average. More importantly, it enables real-time NSR learning with 69.0 scenes per second, on average.
更多查看译文
关键词
neural scene representation,hardware accelerator
AI 理解论文
溯源树
样例
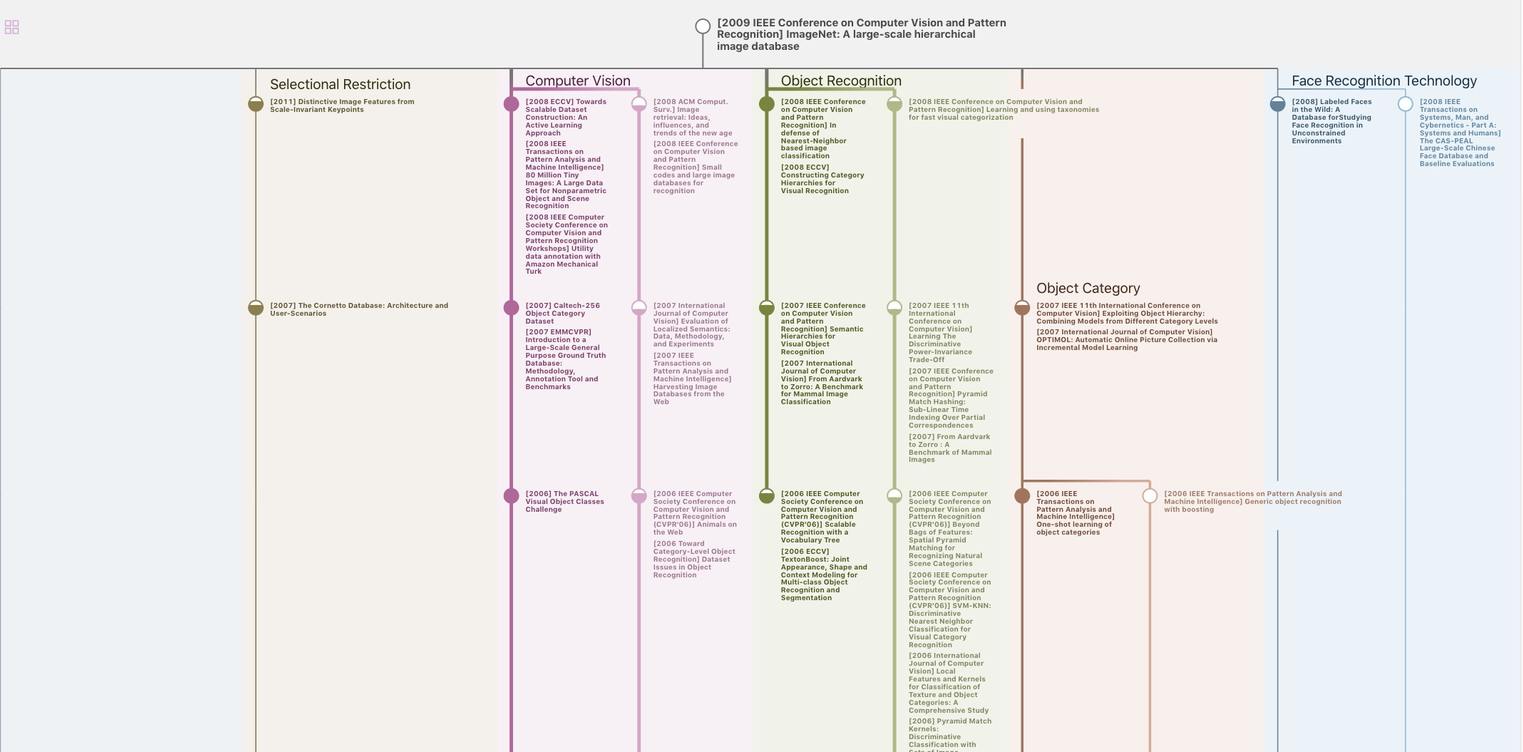
生成溯源树,研究论文发展脉络
Chat Paper
正在生成论文摘要