RM-STC: Row-Merge Dataflow Inspired GPU Sparse Tensor Core for Energy-Efficient Sparse Acceleration
56TH IEEE/ACM INTERNATIONAL SYMPOSIUM ON MICROARCHITECTURE, MICRO 2023(2023)
摘要
This paper proposes RM-STC, a novel GPU tensor core architecture designed for sparse Deep Neural Networks (DNNs) with two key innovations: (1) native support for both training and inference and (2) high efficiency for all sparsity degrees. To achieve the first goal, RM-STC employs a uniform sparse encoding scheme that natively supports all operations holistically in forward and backward passes, thereby eliminating the need for costly sparse encoding transformation in between. For the second goal, RM-STC takes inspiration from the row-merge dataflow and combines the input-gathering and output-scattering hardware features to minimize the energy overhead. Experiments show that RM-STC achieves significant speedups and energy efficiency improvements over dense tensor cores and previous sparse tensor cores.
更多查看译文
关键词
GPU,DNN Acceleration,Sparse Tensor Core
AI 理解论文
溯源树
样例
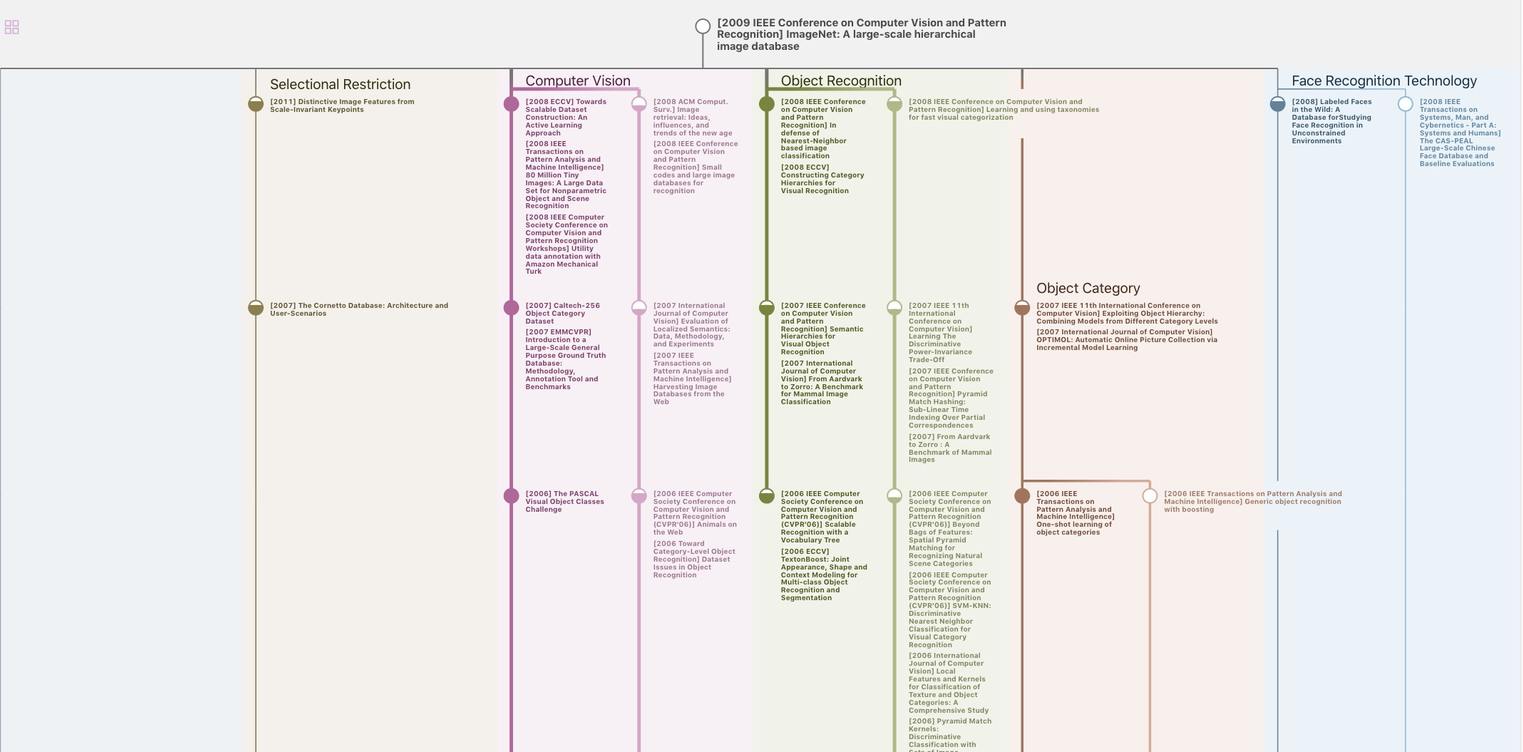
生成溯源树,研究论文发展脉络
Chat Paper
正在生成论文摘要