DOSA: Differentiable Model-Based One-Loop Search for DNN Accelerators
56TH IEEE/ACM INTERNATIONAL SYMPOSIUM ON MICROARCHITECTURE, MICRO 2023(2023)
摘要
In the hardware design space exploration process, it is critical to optimize both hardware parameters and algorithm-to-hardware mappings. Previous work has largely approached this simultaneous optimization problem by separately exploring the hardware design space and the mapspace-both individually large and highly nonconvex spaces-independently. The resulting combinatorial explosion has created significant difficulties for optimizers. In this paper, we introduce DOSA, which consists of differentiable performance models and a gradient descent-based optimization technique to simultaneously explore both spaces and identify high-performing design points. Experimental results demonstrate that DOSA outperforms random search and Bayesian optimization by 2.80x and 12.59x, respectively, in improving DNN model energy-delay product, given a similar number of samples. We also demonstrate the modularity and flexibility of DOSA by augmenting our analytical model with a learned model, allowing us to optimize buffer sizes and mappings of a real DNN accelerator and attain a 1.82x improvement in energy-delay product.
更多查看译文
关键词
Design space exploration,Machine learning accelerators
AI 理解论文
溯源树
样例
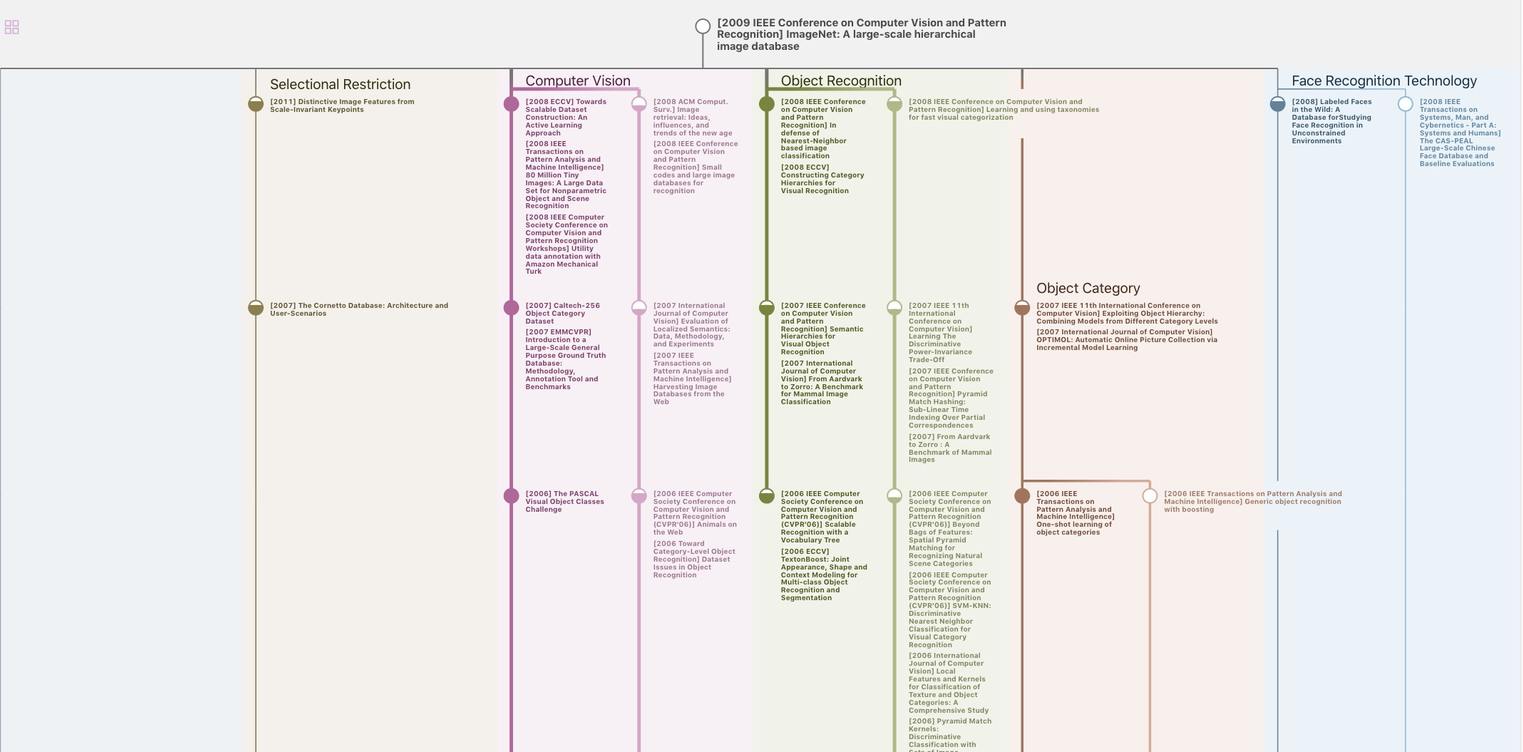
生成溯源树,研究论文发展脉络
Chat Paper
正在生成论文摘要