Incremental semi-supervised graph learning NMF with block-diagonal
ENGINEERING APPLICATIONS OF ARTIFICIAL INTELLIGENCE(2024)
摘要
Non-negative matrix factorization (NMF), as a good data dimensionality reduction method, is widely used in the field of image recognition. Incremental non-negative matrix factorization (INMF) as an improvement solves the problem of inefficiency caused by repeated running of data samples during online learning. However, in the traditional incremental non-negative matrix factorization algorithm, the newly added training samples do not contain label information. Some samples may be marked in both the initial sample and the new training sample in the real application scenario. In order to make full use of the label information carried by the dataset, in this paper, we propose a semi-supervised non-negative matrix factorization model for batch incremental data, incremental semi-supervised graph learning NMF with block diagonal (ISGDNMF). The model is divided into three cases according to the degree of label-carrying for the new batch data: all label-carrying, no label-carrying, and partial label-carrying. The label information is also used to add a diagonal structure to the coefficient matrix, which makes it possible to have stronger discriminatory ability and to distinguish different classes of images more easily. And graph regularization information is added in order to maintain the spatial-geometric structure of the data. Experiments on six image datasets show that this algorithm has superior performance relative to the other seven NMF-based algorithms.
更多查看译文
关键词
Incremental non-negative matrix factorization,Dimensionality reduction,Semi-supervised learning
AI 理解论文
溯源树
样例
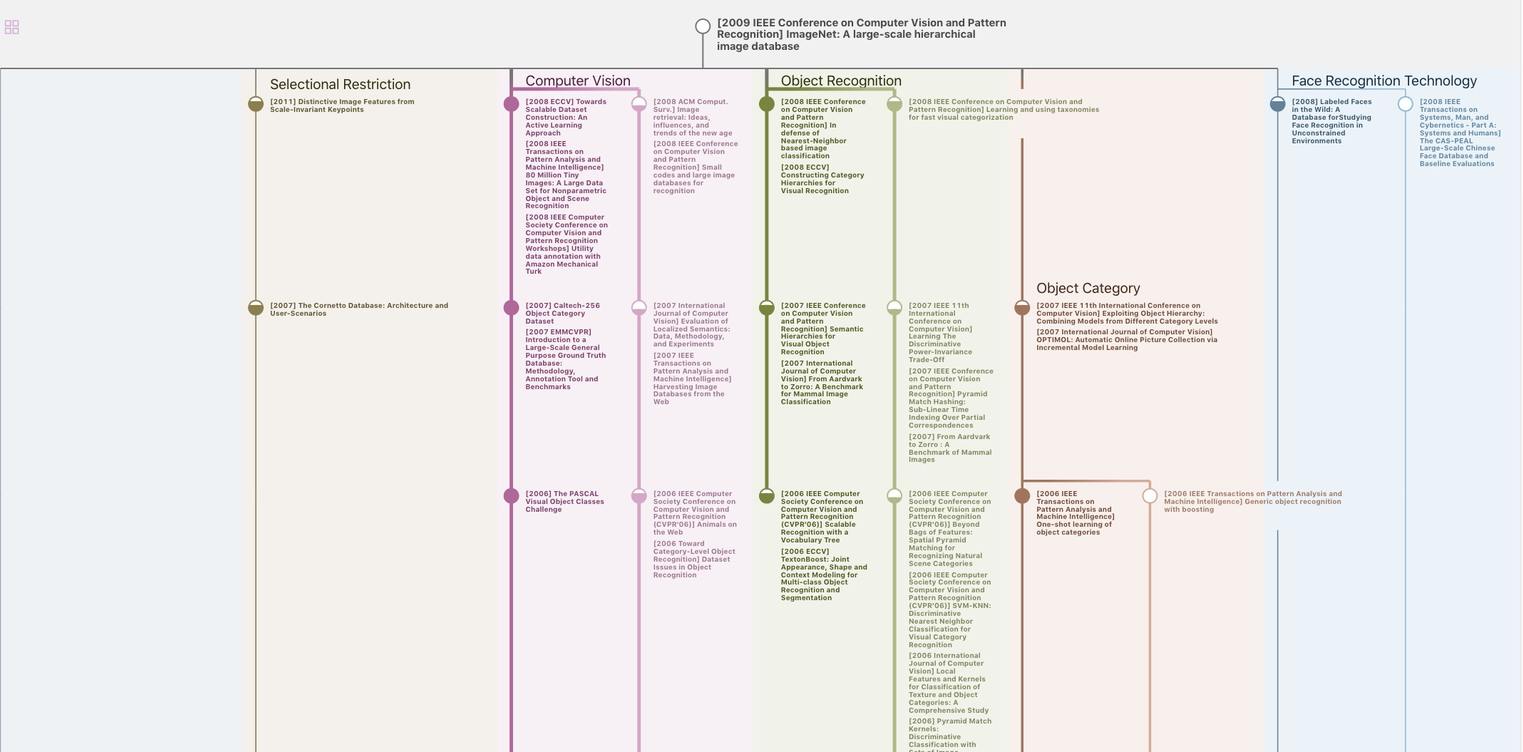
生成溯源树,研究论文发展脉络
Chat Paper
正在生成论文摘要