Defending Against Free-Riders Attacks in Distributed Generative Adversarial Networks
FINANCIAL CRYPTOGRAPHY AND DATA SECURITY, FC 2023, PT II(2024)
摘要
Generative Adversarial Networks (GANs) are increasingly adopted by the industry to synthesize realistic images using competing generator and discriminator neural networks. Due to data not being centrally available, Multi-Discriminator (MD)-GANs training frameworks employ multiple discriminators that have direct access to the real data. Distributedly training a joint GAN model entails the risk of free-riders, i.e., participants that aim to benefit from the common model while only pretending to participate in the training process. In this paper, we first define a free-rider as a participant without training data and then identify three possible actions: not training, training on synthetic data, or using pre-trained models for similar but not identical tasks that are publicly available. We conduct experiments to explore the impact of these three types of free-riders on the ability of MD-GANs to produce images that are indistinguishable from real data. We consequently design a defense against free-riders, termed DFG, which compares the performance of client discriminators to reference discriminators at the server. The defense allows the server to evict clients whose behavior does not match that of a benign client. The result shows that even when 67% of the clients are free-riders, the proposed DFG can improve synthetic image quality by up to 70.96%, compared to the case of no defense.
更多查看译文
关键词
Multi-Discriminator GANs,Free-rider attack,Anomaly detection,Defense
AI 理解论文
溯源树
样例
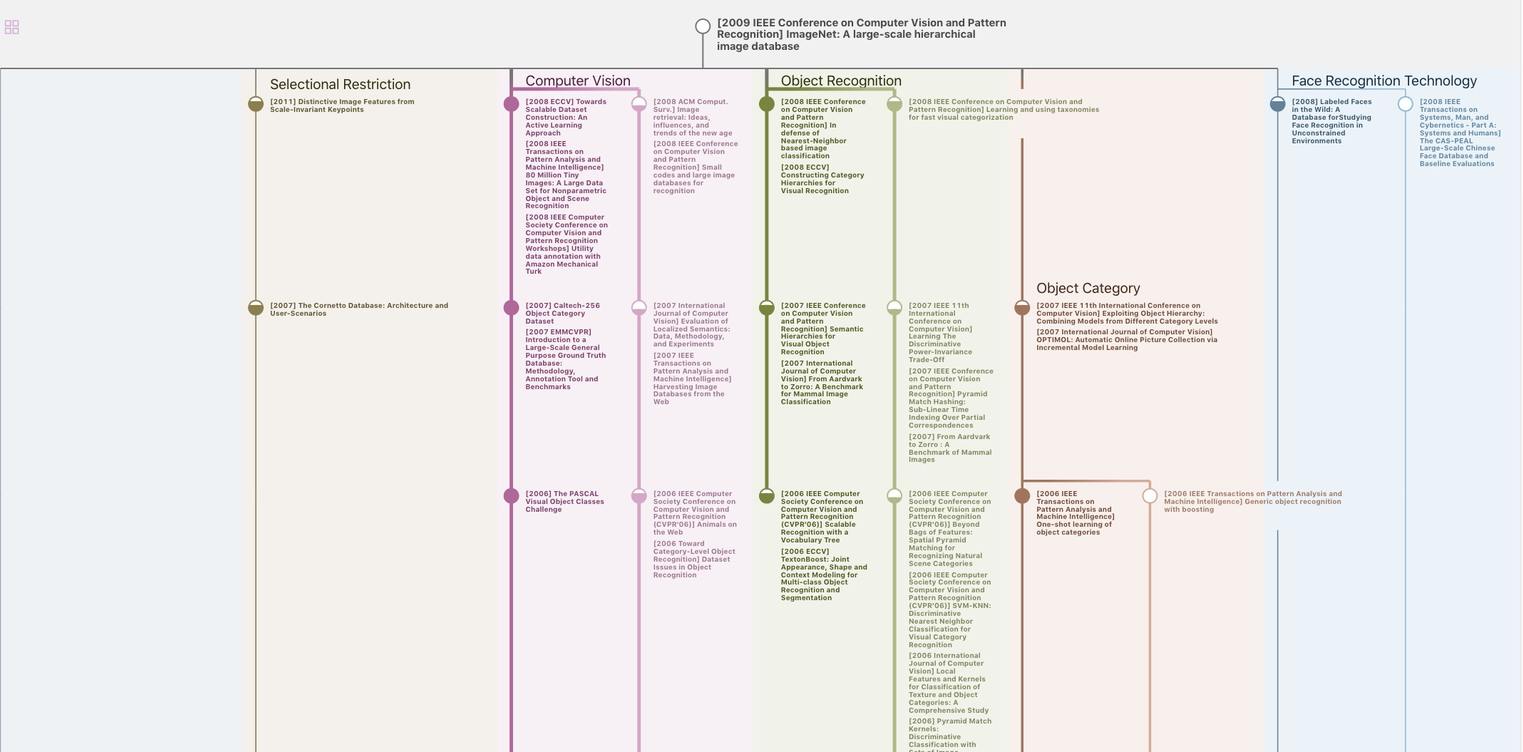
生成溯源树,研究论文发展脉络
Chat Paper
正在生成论文摘要