Non-stationarity Detection in Model-Free Reinforcement Learning via Value Function Monitoring
ADVANCES IN ARTIFICIAL INTELLIGENCE, AI 2023, PT II(2024)
摘要
The remarkable success achieved by Reinforcement learning (RL) in recent years is mostly confined to stationary environments. In realistic settings, RL agents can encounter non-stationarity when the environmental dynamics change over time. Detecting when this change occurs is crucial for activating adaptation mechanisms at the right time. Existing research on change detection mostly relies on model-based techniques which are challenging for tasks with large state and action spaces. In this paper, we propose a model-free, low-cost approach based on value functions (V or Q) for detecting non-stationarity. The proposed approach calculates the change in the value function (Delta V or Delta Q) and monitors the distribution of this change over time. Statistical hypothesis testing is used to detect if the distribution of Delta V or Delta Q changes significantly over time, reflecting non-stationarity. We evaluate the proposed approach in three benchmark RL environments and show that it can successfully detect non-stationarity when changes in the environmental dynamics are introduced at different magnitudes and speeds. Our experiments also show that changes in Delta V or Delta Q can be used for context identification leading to a classification accuracy of up to 88%.
更多查看译文
关键词
Deep Reinforcement Learning,Context Detection,Non-stationarity
AI 理解论文
溯源树
样例
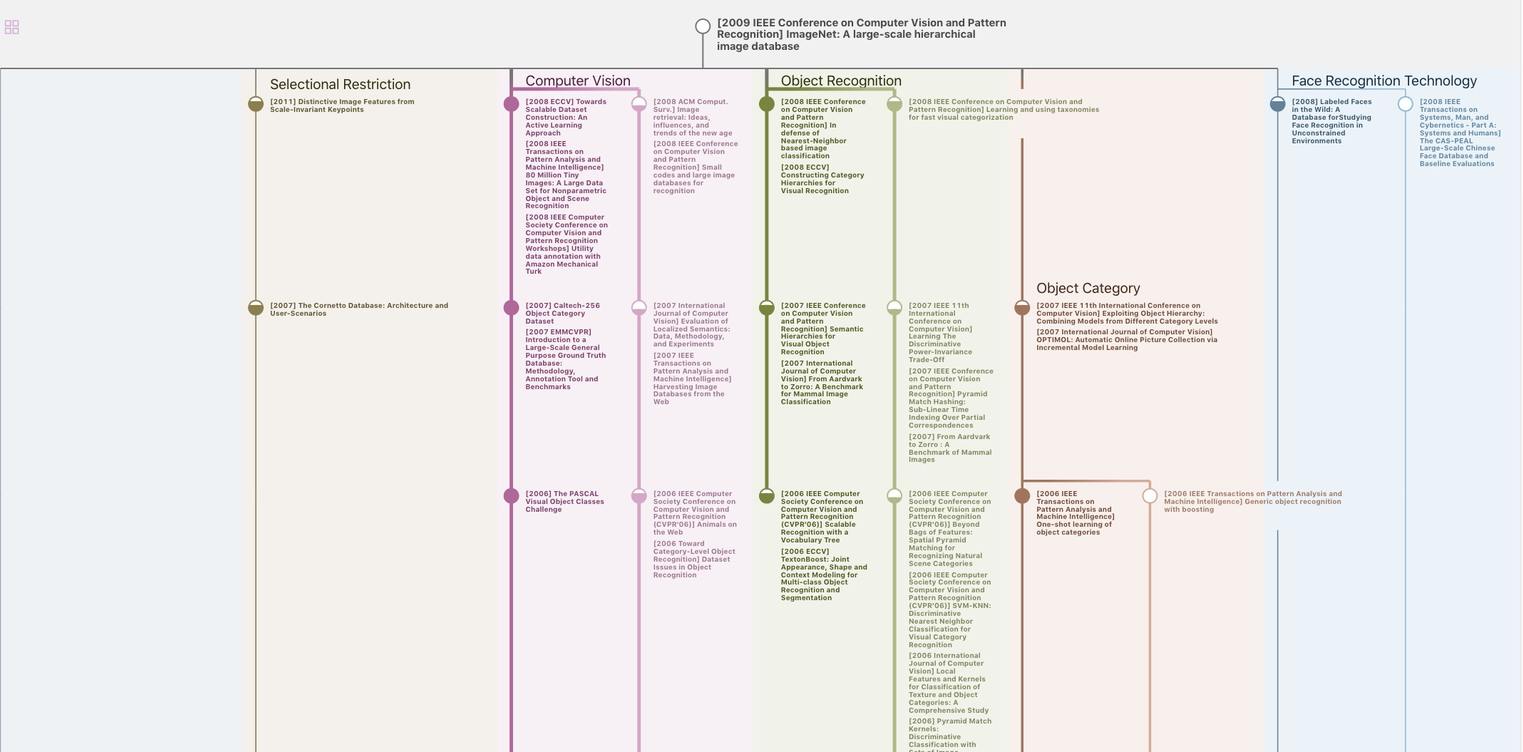
生成溯源树,研究论文发展脉络
Chat Paper
正在生成论文摘要