An Integrated Federated Learning and Meta-Learning Approach for Mining Operations
ADVANCES IN ARTIFICIAL INTELLIGENCE, AI 2023, PT I(2024)
摘要
Mining operations are increasingly adopting advanced technologies to improve operations. These technologies include sensors, drones, robotic drills, and autonomous haul trucks and generate large quantities of data. Analyzing these large datasets can significantly improve mining practices by optimizing outcomes. For example, in drill and blast designs, we can optimize through learning from distributed data processing across multiple sites. However, mines are not equipped to process and handle large amounts of data. The advent of Machine Learning (ML) has enabled the effective handling of large volumes of complex data and the extraction of valuable insights. To accelerate the development of machine learning models for drill and blast design development, we propose an architecture based on two emerging fields of machine learning: Federated Learning (FL) and meta-learning. The proposed architecture facilitates collaboration and encourages sharing best practices among mines while ensuring the data remains local to each mine. In this research, we analyze the proposed architecture, detailing its components and functionalities. Specifically, we aim to determine the optimal learning rate and boosting rounds for unseen mine data using meta-learning. Our evaluation demonstrates that our learning rate and boosting rounds significantly decrease the Root Mean Squared Error (RMSE) value over a standard learning rate by 28.6%, showing the efficacy of the proposed architecture. We examine the potential advantages and inherent challenges of developing a robust system with our architecture, setting the groundwork for future research.
更多查看译文
关键词
Data Privacy,Drill and Blast Design,Federated Learning,Machine Learning,Meta-Learning,Mining
AI 理解论文
溯源树
样例
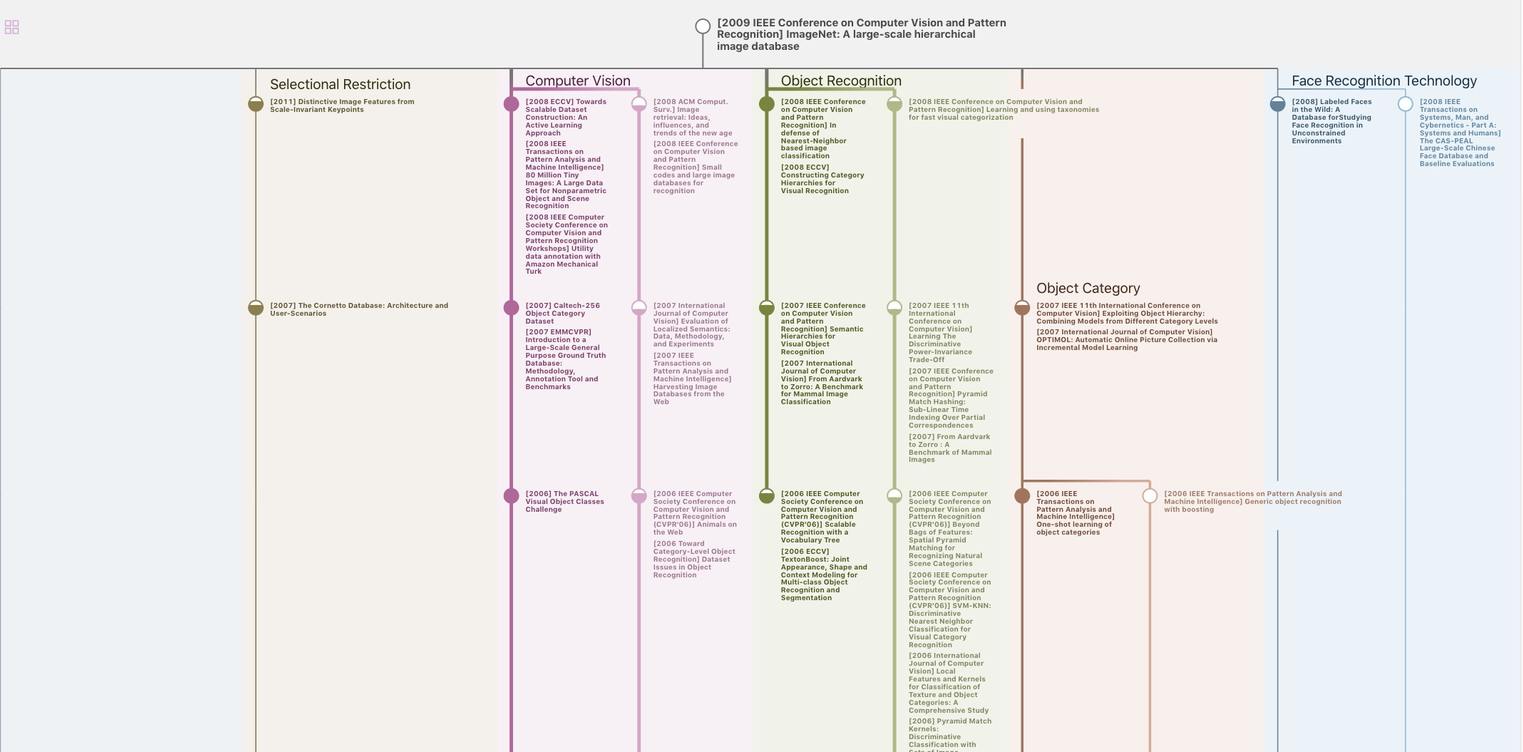
生成溯源树,研究论文发展脉络
Chat Paper
正在生成论文摘要