Multiple Teacher Model for Continual Test-Time Domain Adaptation
ADVANCES IN ARTIFICIAL INTELLIGENCE, AI 2023, PT I(2024)
摘要
Test-time adaptation (TTA) without accessing the source data provides a practical means of addressing distribution changes in testing data by adjusting pre-trained models during the testing phase. However, previous TTA methods typically assume a static, independent target domain, which contrasts with the actual scenario of the target domain changing over time. Using previous TTA methods for long-term adaptation often leads to problems of error accumulation or catastrophic forgetting, as it relies on the capability of a single model, leading to performance degradation. To address these challenges, we propose a multiple teacher model approach (MTA) for continual test-time domain adaptation. Firstly, we reduce error accumulation and leverage the robustness of multiple models by implementing a weighted and averaged multiple teacher model that provides pseudo-labels for enhanced prediction accuracy. Then, we mitigate catastrophic forgetting by logging mutation gradients and randomly restoring some parameters to the weights of the pretrained model. Our comprehensive experiments demonstrate that MTA outperforms other state-of-the-art methods in continual time adaptation.
更多查看译文
关键词
Domain Adaptation,Test-time Adaptation
AI 理解论文
溯源树
样例
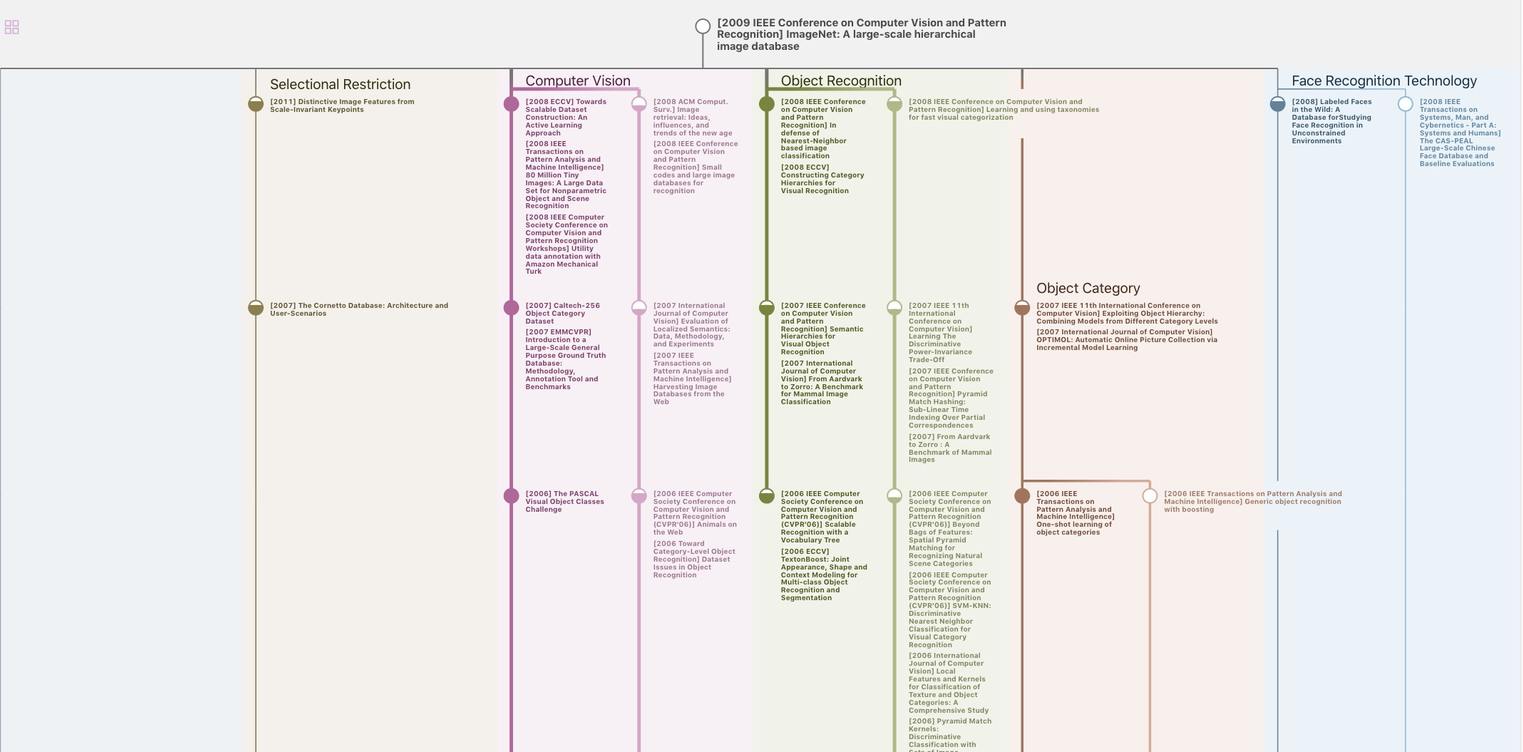
生成溯源树,研究论文发展脉络
Chat Paper
正在生成论文摘要