Knowledge Distillation with Feature Enhancement Mask
ARTIFICIAL NEURAL NETWORKS AND MACHINE LEARNING, ICANN 2023, PT VIII(2023)
摘要
Knowledge distillation transfers knowledge from the teacher model to the student model, aiming to improve the student's performance. Previous methods mainly focus on effective feature transformations and alignments to improve distillation efficiency and reduce information loss. However, these approaches ignore the differences between different pixels and layers, which contribute differently during distillation. To this end, we propose the novel knowledge distillation with feature enhancement mask (FEM). The FEM consists of two components: pixel-level feature enhancement mask and layer-level dynamic importance. The pixel-level feature enhancement mask treats target object and non-target object differently during distillation to help the student capture the teacher's crucial features. The layer-level dynamic importance dynamically regulates the effect of each layer in distillation. Extensive experiments on CIFAR-100 indicate that FEM can help the student capture the teacher's crucial features and outperforms previous One-to-One distillation methods even One-to-Many distillation methods.
更多查看译文
关键词
Knowledge distillation,Feature enhancement mask,Crucial feature,Dynamic importance
AI 理解论文
溯源树
样例
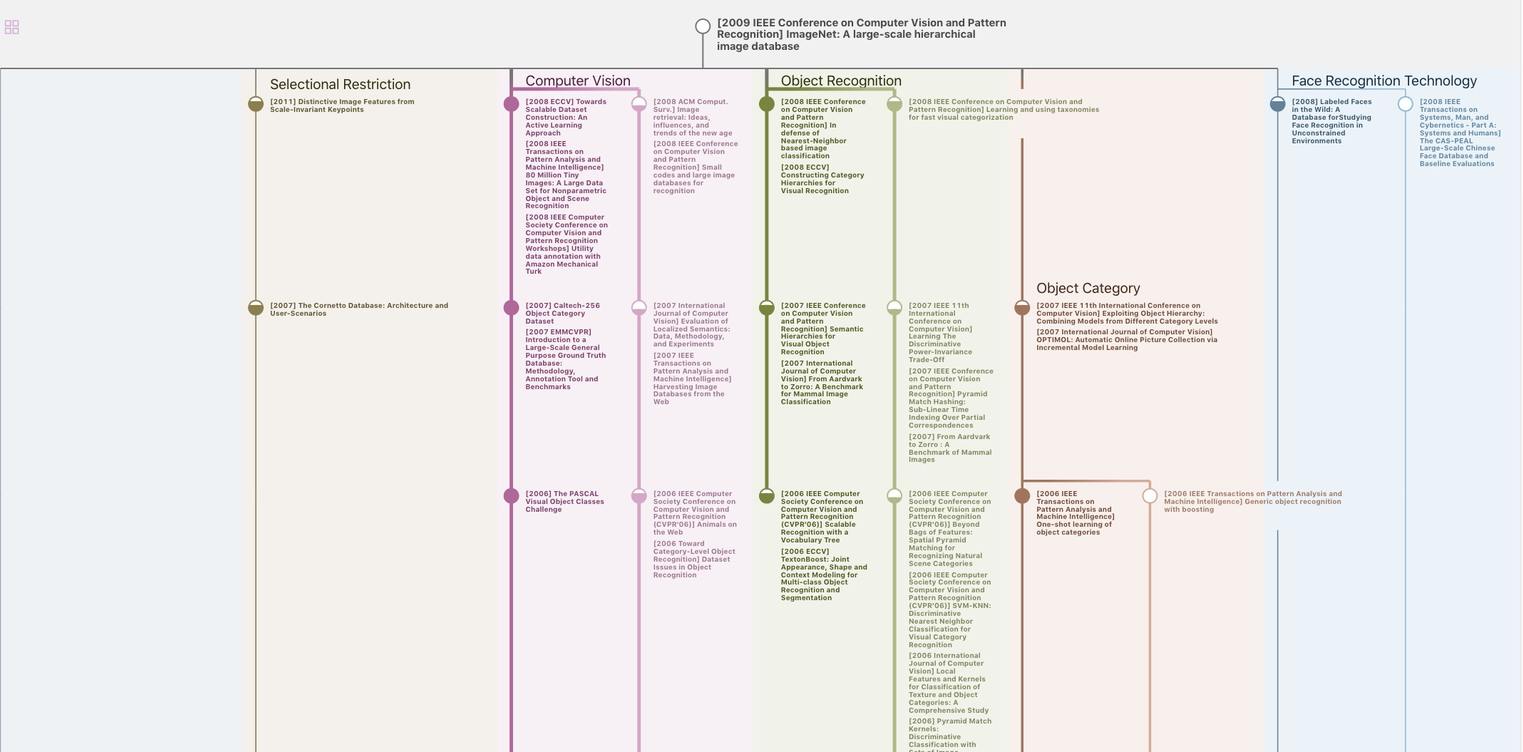
生成溯源树,研究论文发展脉络
Chat Paper
正在生成论文摘要