Symbolic Data Analysis to Improve Completeness of Model Combination Methods
ADVANCES IN ARTIFICIAL INTELLIGENCE, AI 2023, PT II(2024)
摘要
A growing number of organizations are adopting a strategy of breaking down large data analysis problems into specific sub-problems, tailoring models for each. However, handling a large number of individual models can pose challenges in understanding organization-wide phenomena. Recent studies focus on using decision trees to create a consensus model by aggregating local decision trees into sets of rules. Despite efforts, the resulting models may still be incomplete, i.e., not able to cover the entire decision space. This paper explores methodologies to tackle this issue by generating complete consensus models from incomplete rule sets, relying on rough estimates of the distribution of independent variables. Two approaches are introduced: synthetic dataset creation followed by decision tree training and a specialized algorithm for creating a decision tree from symbolic data. The feasibility of generating complete decision trees is demonstrated, along with an empirical evaluation on a number of datasets.
更多查看译文
关键词
symbolic data,model completeness,consensus models
AI 理解论文
溯源树
样例
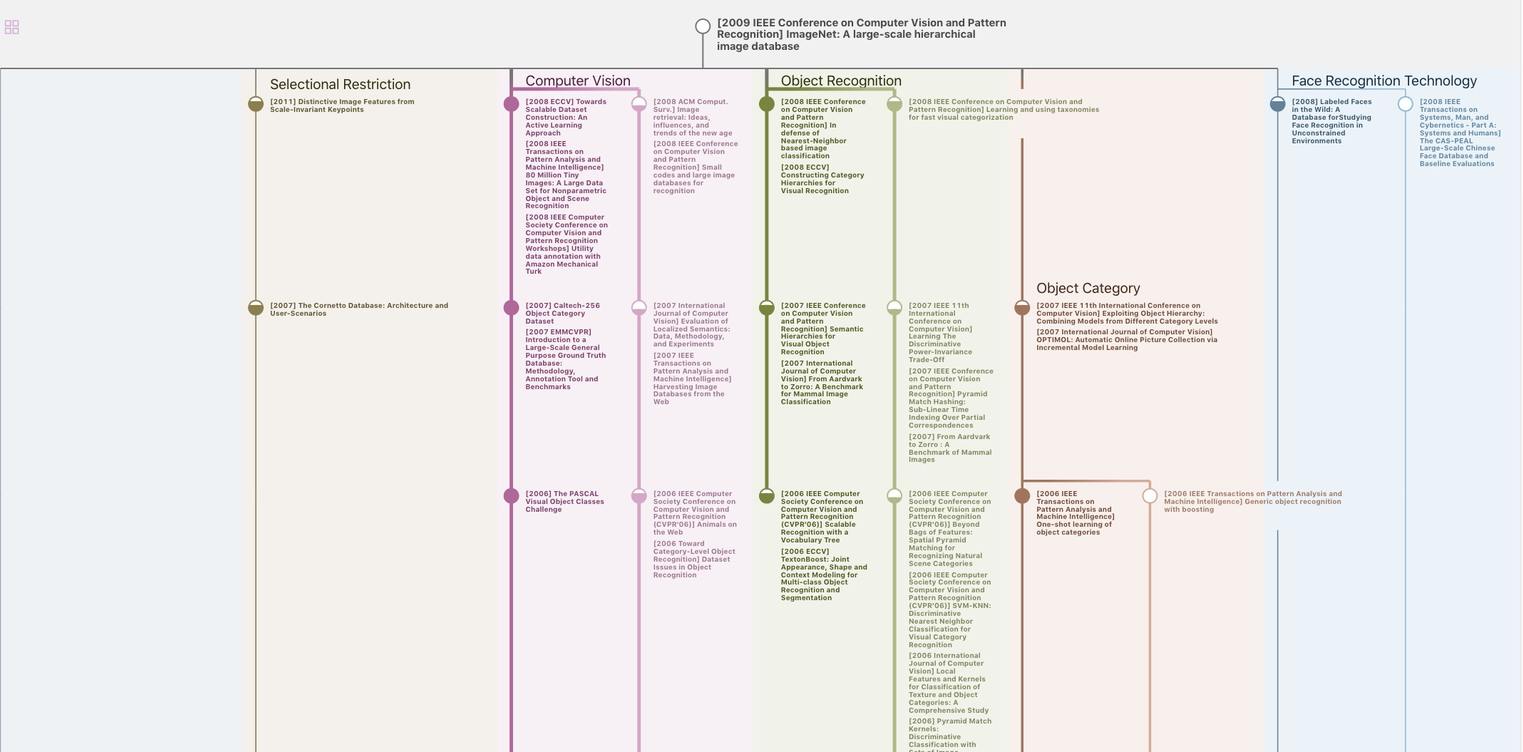
生成溯源树,研究论文发展脉络
Chat Paper
正在生成论文摘要