Fast, Memory-Efficient Spectral Clustering with Cosine Similarity
PROGRESS IN PATTERN RECOGNITION, IMAGE ANALYSIS, COMPUTER VISION, AND APPLICATIONS, CIARP 2023, PT I(2024)
摘要
Spectral clustering is a popular and effective method but known to face two significant challenges: scalability and out-of-sample extension. In this paper, we extend the work of Chen (ICPR 2018) on the speed scalability of spectral clustering in the setting of cosine similarity to deal with massive or online data that are too large to be fully loaded into computer memory. We start by assuming a small batch of data drawn from the full set and develop an efficient procedure that learns both the nonlinear embedding and clustering map from the sample and extends them easily to the rest of the data as they are gradually loaded. We then introduce an automatic approach to selecting the optimal value of the sample size. The combination of the two steps leads to a streamlined memory-efficient algorithm that only uses a small number of batches of data (as they become available), with memory and computational costs that are independent of the size of the data. Experiments are conducted on benchmark data sets to demonstrate the fast speed and excellent accuracy of the proposed algorithm. We conclude the paper by pointing out several future research directions.
更多查看译文
关键词
Spectral clustering,Cosine similarity,Speed scalability,Memory scalability
AI 理解论文
溯源树
样例
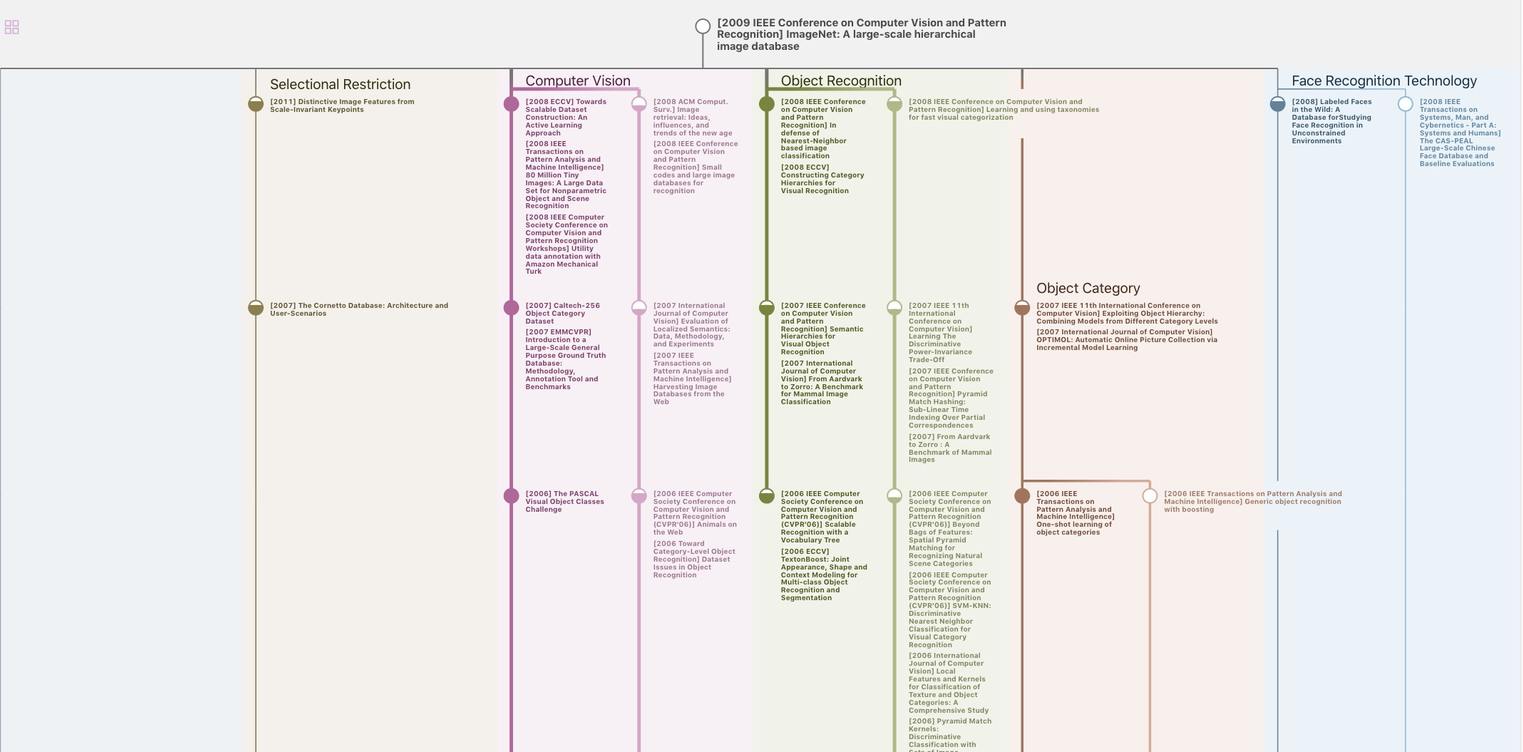
生成溯源树,研究论文发展脉络
Chat Paper
正在生成论文摘要