Filtering Safe Temporal Motifs in Dynamic Graphs for Dissemination Purposes
PROGRESS IN PATTERN RECOGNITION, IMAGE ANALYSIS, COMPUTER VISION, AND APPLICATIONS, CIARP 2023, PT I(2024)
摘要
In this paper, we address the challenges posed by dynamic networks in various domains, such as bioinformatics, social network analysis, and computer vision, where relationships between entities are represented by temporal graphs that respect a temporal order. To understand the structure and functionality of such systems, we focus on small subgraph patterns, called motifs, which play a crucial role in understanding dissemination processes in dynamic networks that can be a spread of fake news, infectious diseases or computer viruses. To address this, we propose a novel approach called temporal motif filtering for classifying dissemination processes in labeled temporal graphs. Our approach identifies and examines key temporal subgraph patterns, contributing significantly to our understanding of dynamic networks. To further enhance classification performance, we combined directed line transformations with temporal motif removal. Additionally, we integrate filtering motifs, directed edge transformations, and transitive edge reduction. Experimental results demonstrate that our proposed approaches consistently improve classification accuracy across various datasets and tasks. These findings hold the potential to unlock deeper insights into diverse domains and enable the development of more accurate and efficient strategies to address challenges related to spreading process in dynamic environments. Our work significantly contributes to the field of temporal graph analysis and classification, opening up new avenues for advancing our understanding and utilization of dynamic networks.
更多查看译文
关键词
Temporal motifs,temporal graphs,temporal graph classification
AI 理解论文
溯源树
样例
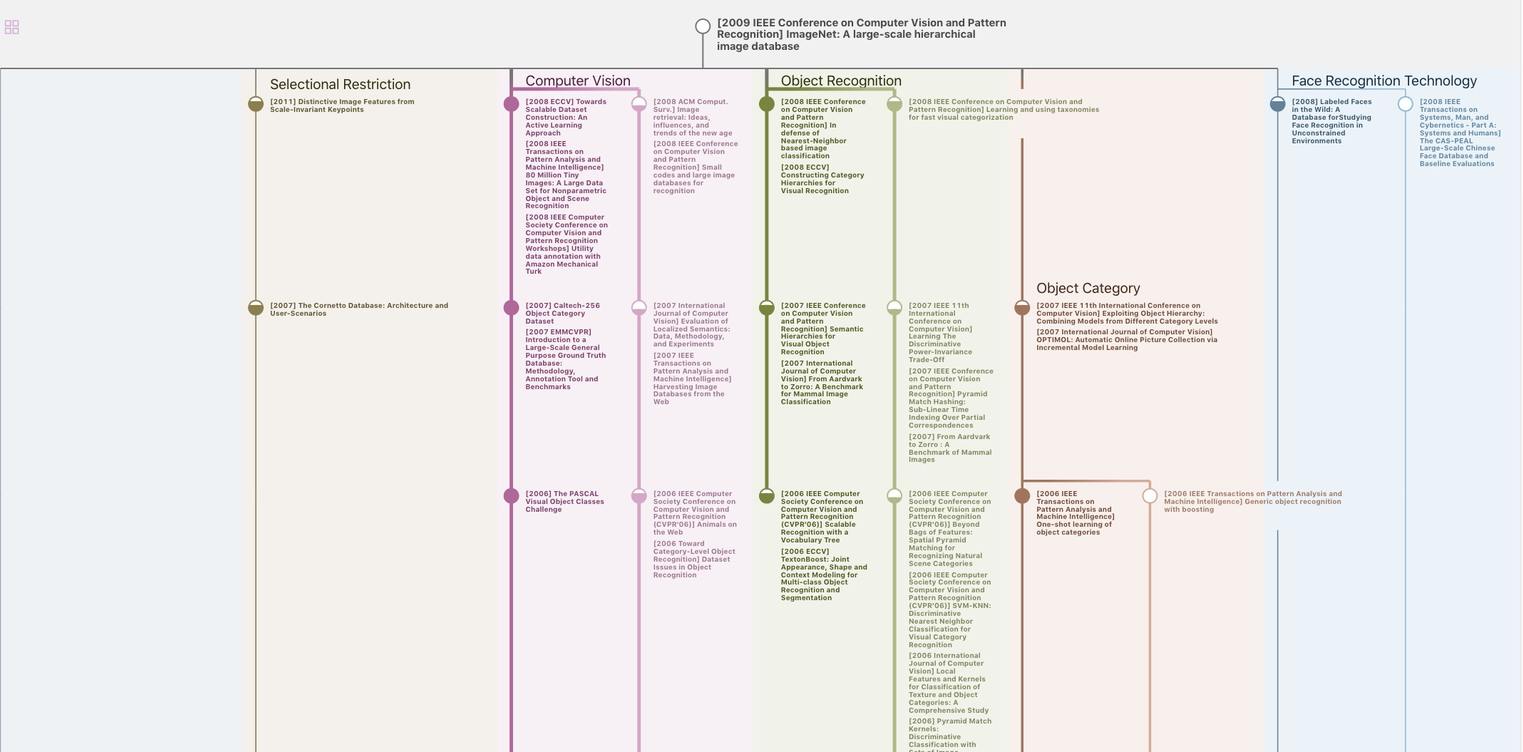
生成溯源树,研究论文发展脉络
Chat Paper
正在生成论文摘要