Mortality Prediction via Logistic Regression in Intensive Care Unit Patients with Pneumonia
PROGRESS IN PATTERN RECOGNITION, IMAGE ANALYSIS, COMPUTER VISION, AND APPLICATIONS, CIARP 2023, PT II(2024)
Abstract
This work focuses on the problem of mortality prediction in patients with pneumonia after admission into an intensive care unit, by addressing it via logistic regression. This approach can model the relationship between clinical correlates and the probability of the binary outcome, with obvious advantages such as simplicity and interpretability of the predictive models. This work further inspects the potential of localized models, an approach based on different (parallel) predictive models each one constructed in clusters automatically identified in the training set. The predicted outcome is then obtained via membership separation (M, which corresponds to the outcome of the closest localized model) or weights (W, outcome as the weight average of localized outcomes via inverse distance). The results point out a similar balanced accuracy of 0.73 for the global model M24-48PS (without oversampling) and the W M24-48PSC model (weighted average of localized models without oversampling), which is partially explained by the small separability between the identified clusters. Therefore, a proof of concept was performed to support the usefulness of localized models in more separable data. This study considered a small amount of data for training and testing (chosen as that closest to the centroids of the identified clusters) and the results suggest that the localized approach can outperform the global one in more separable data.
MoreTranslated text
Key words
Pneumonia Mortality,Machine Learning,Logistic Regression,Localized Models
AI Read Science
Must-Reading Tree
Example
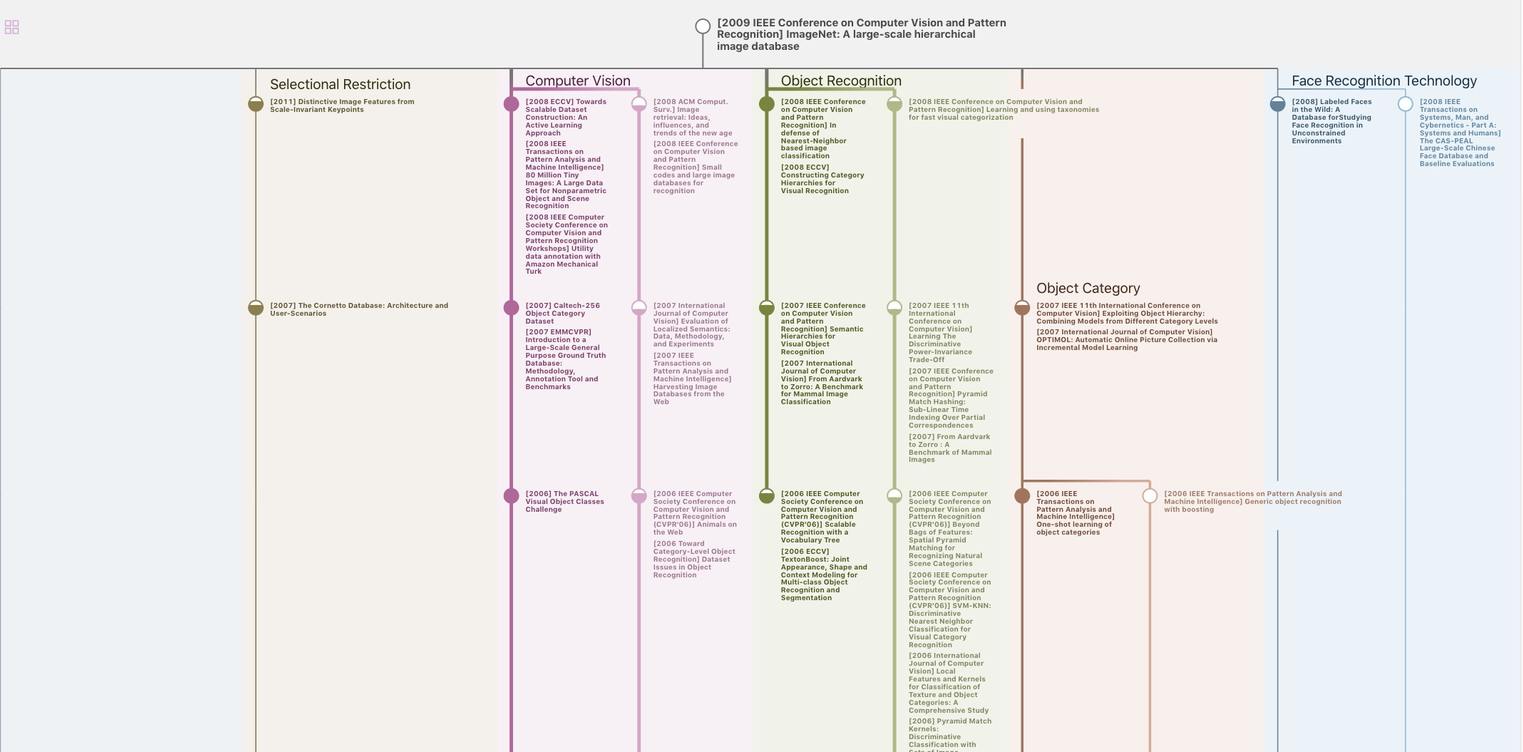
Generate MRT to find the research sequence of this paper
Chat Paper
Summary is being generated by the instructions you defined