BliMSR: Blind Degradation Modelling for Generating High-Resolution Medical Images
MEDICAL IMAGE UNDERSTANDING AND ANALYSIS, MIUA 2023(2024)
Abstract
A persisting problem with existing super-resolution (SR) models is that they cannot produce minute details of anatomical structures, pathologies, and textures critical for proper diagnosis. This is mainly because they assume specific degradations like bicubic downsampling or Gaussian noise, whereas, in practice, the degradations can be more complex and hence need to be modelled "blindly". We propose a novel attention-based GAN model for medical image super-resolution that models the degradation in a data-driven agnostic way ("blind") to achieve better fidelity of diagnostic features in medical images. We introduce a new ensemble loss in the generator that boosts performance and a spectral normalisation in the discriminator to enhance stability. Experimental results on lung CT scans demonstrate that our model, BliMSR, produces super-resolved images with enhanced details and textures and outperforms recent competing models, including a diffusion model for generating super-resolution images, thus establishing a state-of-the-art. The code is available at https://github.com/Samiran-Dey/BliMSR.
MoreTranslated text
AI Read Science
Must-Reading Tree
Example
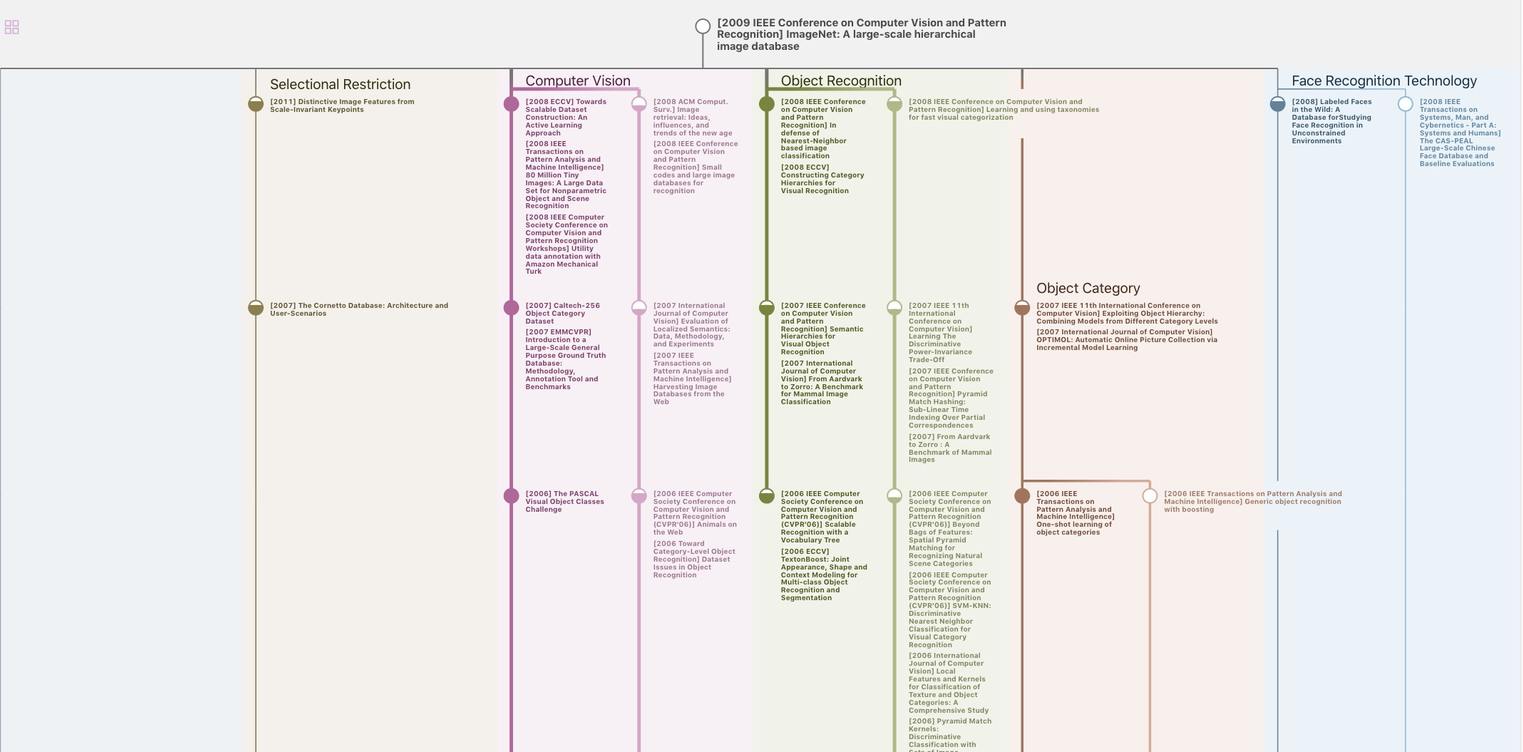
Generate MRT to find the research sequence of this paper
Chat Paper
Summary is being generated by the instructions you defined