Modified dual attention triplet-supervised hashing network for image retrieval
Signal, Image and Video Processing(2023)
Abstract
In view of the problems of insufficient feature extraction and ineffective capture of correlation between deep features in existing image retrieval methods, a modified dual attention triplet-supervised hashing network (MDATSH) is proposed. A modified dual attention module is added after the deep neural network, that is, based on dual attention, where two attention modules are, respectively, equipped with a local branch. Specifically, a local spatial attention branch is added to the position attention module and a local channel attention branch is added to the channel attention module, which captures global dependencies while avoiding the loss of local information and effectively capturing the correlation between deep features of the image. By combining these two attention mechanisms, the network is capable of effectively extracting crucial information from input images, thereby enhancing the robustness of image feature representation. Meanwhile, a dynamic cross-entropy loss function is introduced to dynamically adjust the loss weights during model training, which is combined with the triple loss function to enhance the class separability of image hash codes while maintaining semantic similarity. The experimental results on three public datasets show that the performance of MDATSH is effectively improved in image retrieval.
MoreTranslated text
Key words
Image retrieval,Deep learning,Supervised hashing,Attention mechanism
AI Read Science
Must-Reading Tree
Example
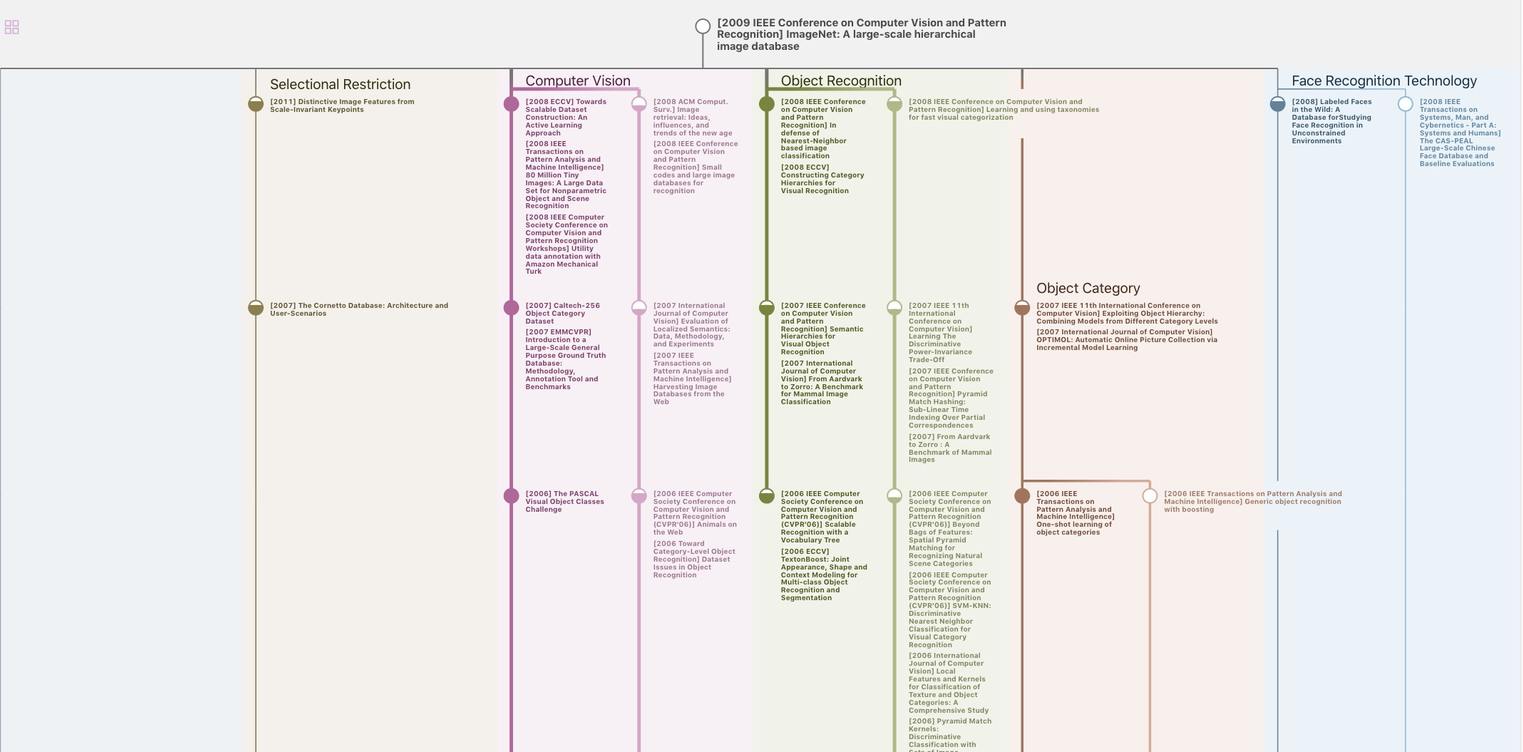
Generate MRT to find the research sequence of this paper
Chat Paper
Summary is being generated by the instructions you defined