A transferred hybrid surrogate model integrating Gaussian membership virtual sample generation for small sample prediction: Applications in metal tube bending
ENGINEERING APPLICATIONS OF ARTIFICIAL INTELLIGENCE(2024)
摘要
The high-performance virtual sample generation (VSG) method has been extensively introduced to solve the problem of small sample sizes. Data distribution information is a key element of current VSG methods at the datadriven level. Herein, we propose an improved VSG method with a Gaussian distribution and explore the relationship between the Gaussian function and data expansion. To obtain more feasible virtual samples, information expanded based on the Gaussian membership function (GMIE) was established. For further improvement, a hybrid surrogate model based on transfer (THSM) is proposed, which differs from the general hybrid surrogate model (HSM) methods that only mix single models. Using a prevailing evaluation method, our proposed method, which combines a Gaussian membership function with a hybrid surrogate model, outperforms other competing approaches in 12 numerical cases owing to its feasibility and efficacy. Additionally, the proposed approach is applied to a metal tube rotary draw bending (RDB) prediction problem to illustrate its ability to support complex engineering designs.
更多查看译文
关键词
Virtual sample generation,Hybrid surrogate model,Rotary draw bending,Distortion prediction,Batch simulation
AI 理解论文
溯源树
样例
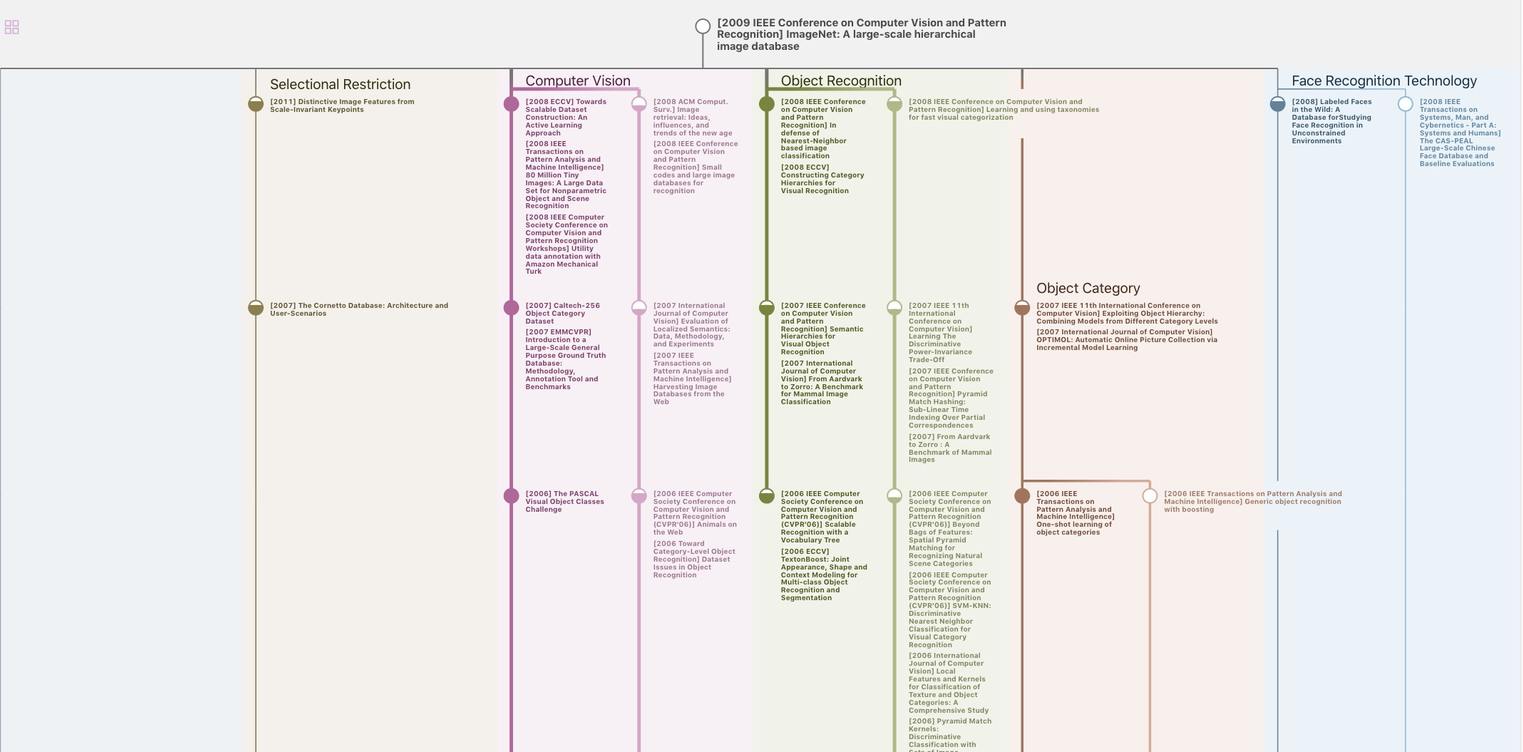
生成溯源树,研究论文发展脉络
Chat Paper
正在生成论文摘要