KD loss: Enhancing discriminability of features with kernel trick for object detection in VHR remote sensing images
ENGINEERING APPLICATIONS OF ARTIFICIAL INTELLIGENCE(2024)
摘要
The classification accuracy of the object detection relies on the network's ability to learn discriminative features on very high resolution (VHR) remote sensing images. Popular optimization of the feature representation is implemented with loss functions by minimizing intra-class distance and maximizing inter-class distance in the original feature space. However, existing loss functions may be insufficient in capturing the complex nonlinear relationships inherent in the data, requiring further improvement to achieve accurate classification. To address this issue, we propose a new loss function, referred to as the kernel-based discriminative loss (KD loss), by constructing weight term using the kernel trick and applying them to re-weight the softmax cross-entropy loss. Specifically, the KD loss maps the class centers of deep features to the Hilbert space and calculates the distance between each class center using the Mercer kernel. Besides, the KD loss also employs kernel function to learn the similarity between the deep features and their corresponding class center, thereby facilitating the model in acquiring more discriminative and class-specific feature representations. Compared to existing loss functions, the KD loss improves the feature discriminability in the high-dimensional Hilbert space for the first time. Extensive experiments on the DOTA and RSOD datasets demonstrate that the KD loss can significantly improve the performance of VHR remote sensing object detection.
更多查看译文
关键词
Object detection,Convolutional neural network,Transformer,Remote sensing
AI 理解论文
溯源树
样例
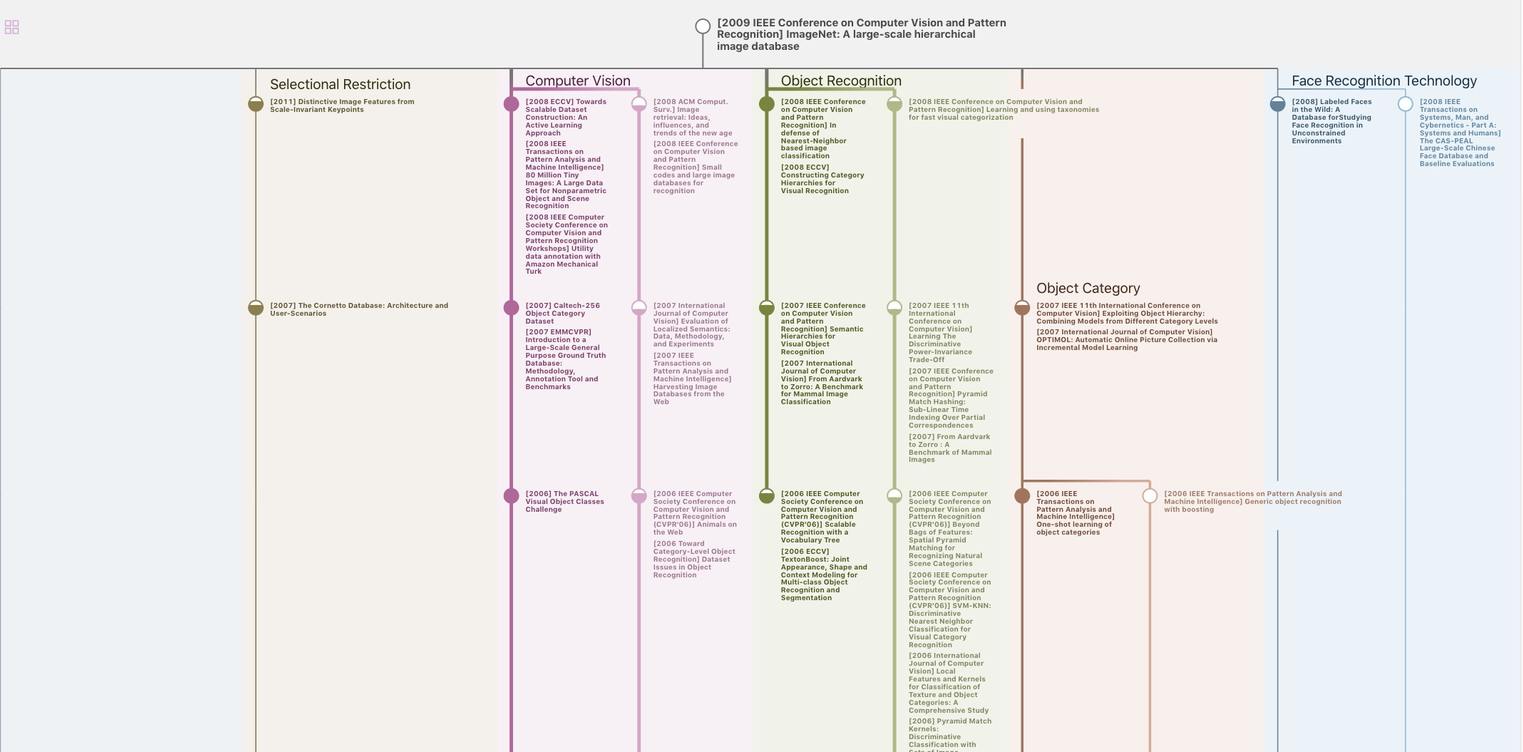
生成溯源树,研究论文发展脉络
Chat Paper
正在生成论文摘要