Iteratively reweighted accurate sparse low-rank matrix estimation algorithm for bearing fault diagnosis
MEASUREMENT(2024)
Abstract
Bearing fault diagnosis is significant to ensure the safe functioning of mechanical systems. One of the key issues in bearing fault diagnosis is the accurate and effective extraction of fault characteristic features. In order to further explore the construction of penalty function, further alleviate the problem of bias estimation and enhance the capabilities of the sparse low-rank (SLR) method in effectively diagnosing bearing faults, in this paper, an iteratively reweighted accurate sparse low-rank (IRASLR) matrix estimation algorithm is proposed. Taking the sparse and low-rank properties of fault transient signals in time-frequency domain into account, an optimization problem is formulated. Two penalty functions, derived from a core minimax-concave penalty (MCP), induce sparse and low-rank properties, respectively. Specifically, to facilitate the design of weighting schemes, we construct an equivalent variant of MCP (EVMCP). By separately extending EVMCP to matrix elements and singular values, we obtain equivalent weighted MCP and equivalent weighted matrix gamma - norm. Finally, based on the proximal operators of the two penalty functions, we deduce an IRASLR algorithm under the alternating direction method of the multipliers (ADMM) framework. The performance of IRASLR is verified by processing simulated and experimental signals, while the superiority of the algorithm is confirmed through comparative experiments.
MoreTranslated text
Key words
Bearing fault diagnosis,Sparse low -rank matrix estimation,Equivalent variant of minimax-concave penalty,(EVMCP),Weighting scheme
AI Read Science
Must-Reading Tree
Example
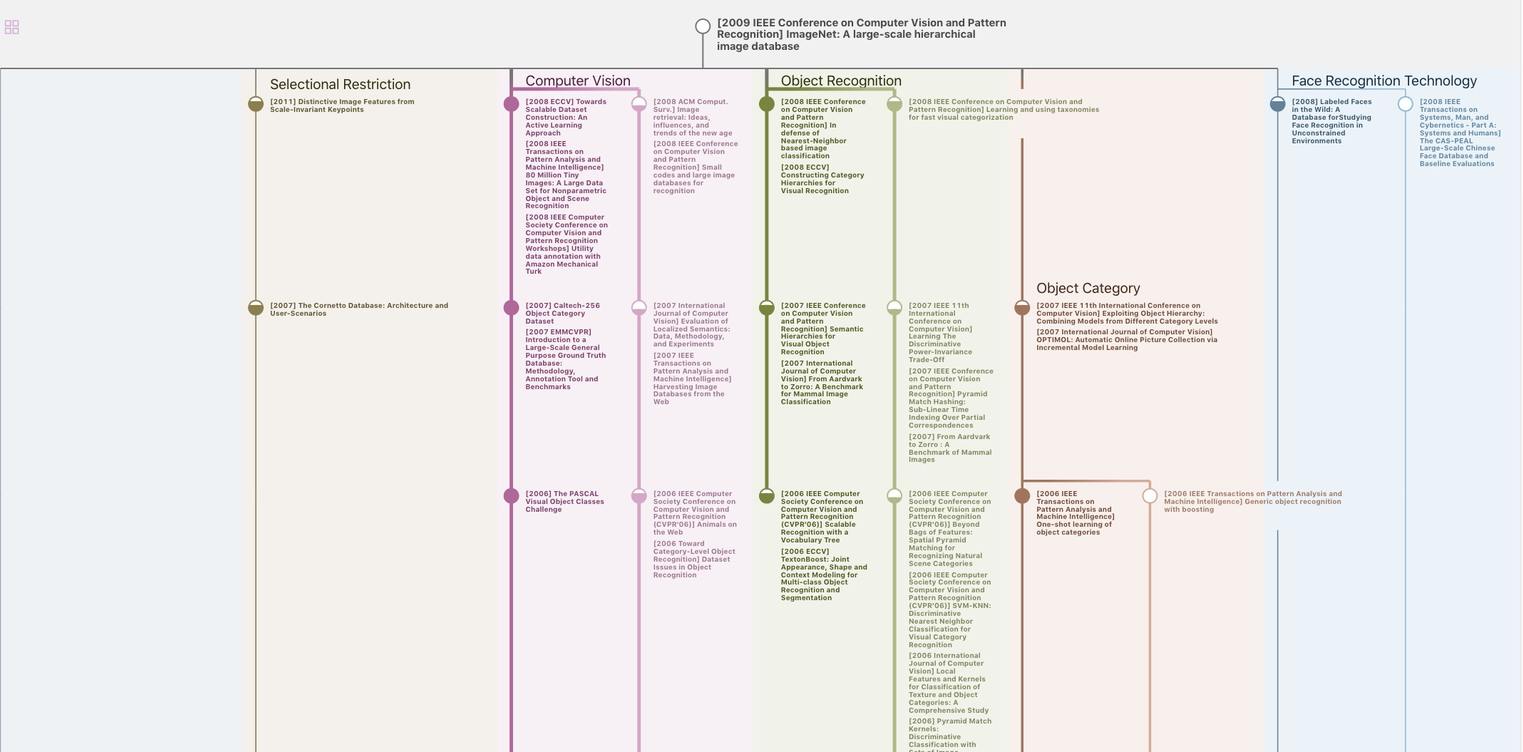
Generate MRT to find the research sequence of this paper
Chat Paper
Summary is being generated by the instructions you defined