Machine learning-assisted high precision predictive modelling of convective heat transfer in fluid channels fabricated by laser powder bed fusion
INTERNATIONAL JOURNAL OF THERMAL SCIENCES(2024)
摘要
Laser powder bed fusion (LPBF) has proven to be an effective tool in fabricating heat transfer devices with improved efficiency. However, the accurate prediction of convective heat transfer in LPBF-fabricated fluid channels remains a challenge. The classical Gnielinski model is regarded as the most accurate correlation for predicting forced convective heat transfer in traditional pipes. However, whether it is applicable to LPBFfabricated pipes is yet to be determined. To address this challenge, in this study, pipe samples with diameters of 3 mm, 4 mm, and 5 mm were designed and fabricated using LPBF along the building angles of 0 degrees, 45 degrees, and 90 degrees. The pressure loss and heat transfer characteristics of these samples were experimentally measured. Results showed that there was a maximum prediction error of 72.1 % between the classical Gnielinski model and experimental results. To improve the prediction accuracy, a corrected Colebrook model with improved prediction accuracy of the friction factor was developed, introduced into the classical Gnielinski model, and reduced the maximum prediction error down to 36.8 %. To further improve the prediction accuracy, a gradient descentbased machine learning method was adopted to reconstruct the Gnielinski model, which achieved a high prediction accuracy with prediction errors of below 10 %. With the assistance of machine learning, a high precision predictive model of convective heat transfer in LPBF-fabricated fluid channels was established.
更多查看译文
关键词
Laser powder bed fusion,Convective heat transfer,Friction factor,Fluid channels,Predictive model,Machine learning
AI 理解论文
溯源树
样例
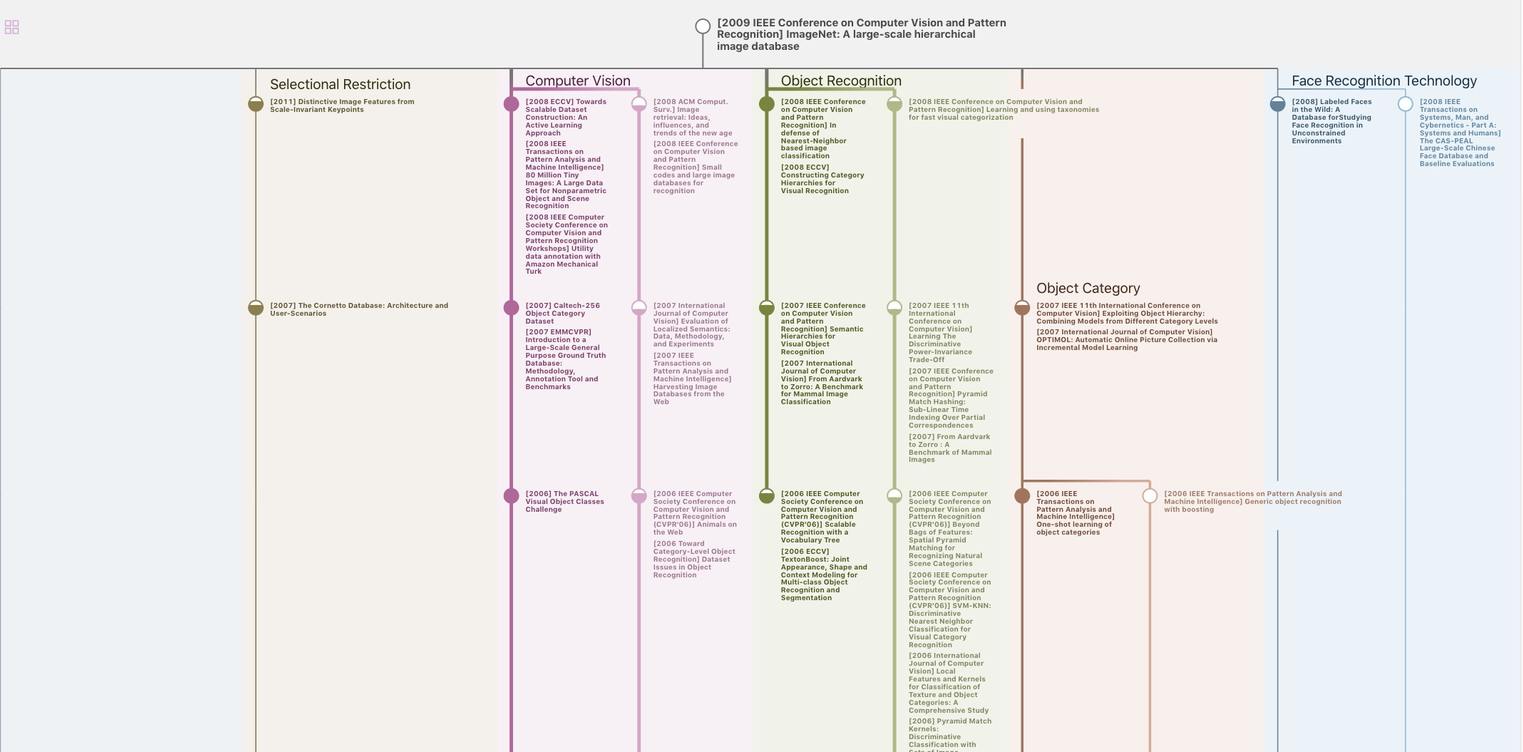
生成溯源树,研究论文发展脉络
Chat Paper
正在生成论文摘要