Single and ensemble explainable machine learning-based prediction of membrane flux in the reverse osmosis process
JOURNAL OF WATER PROCESS ENGINEERING(2024)
摘要
Reverse osmosis is the most popular membrane-based desalination process that accounts presently for more than half the worldwide desalination capacity. However, the complex involvement of a variety of factors in this process has hindered the efficient assessment of the process performance such as accurately determining the membrane flux. It is therefore indispensable to search for reliable and flexible tools for the estimation of membrane flux in reverse osmosis such as machine learning. In this study, for the first time, nine different machine learning algorithms, ranging from simple white box models to complex black box models, were investigated for the accurate prediction of membrane flux in reverse osmosis using a large dataset of 401 experimental points retrieved from literature with 8 distinct features. The investigation has shown superior predictive performance for ensemble models over single models. In addition, extreme gradient boosting stood out as the best-performing ensemble model for the prediction of membrane flux due to having the lowest statistical errors (MAE = 1.78 LMH, MAPE = 8.88 %, and RMSE = 2.32 LMH) and strongest correlations with R2 = 98.2 %, IA = 99.55 %, and KGE = 98.84 %, in the test dataset. The Unified Shapley Additive Explanation technique was also employed to determine the influence of the input features, and the most impactful parameters were found to be the feedwater flow rate and applied pressure. The results of the present study suggest that machine learning algorithms, especially ensemble ones, are powerful tools in forecasting the membrane flux of the reverse osmosis process.
更多查看译文
关键词
Reverse osmosis,Membrane flux,Machine learning,Single models,Ensemble models
AI 理解论文
溯源树
样例
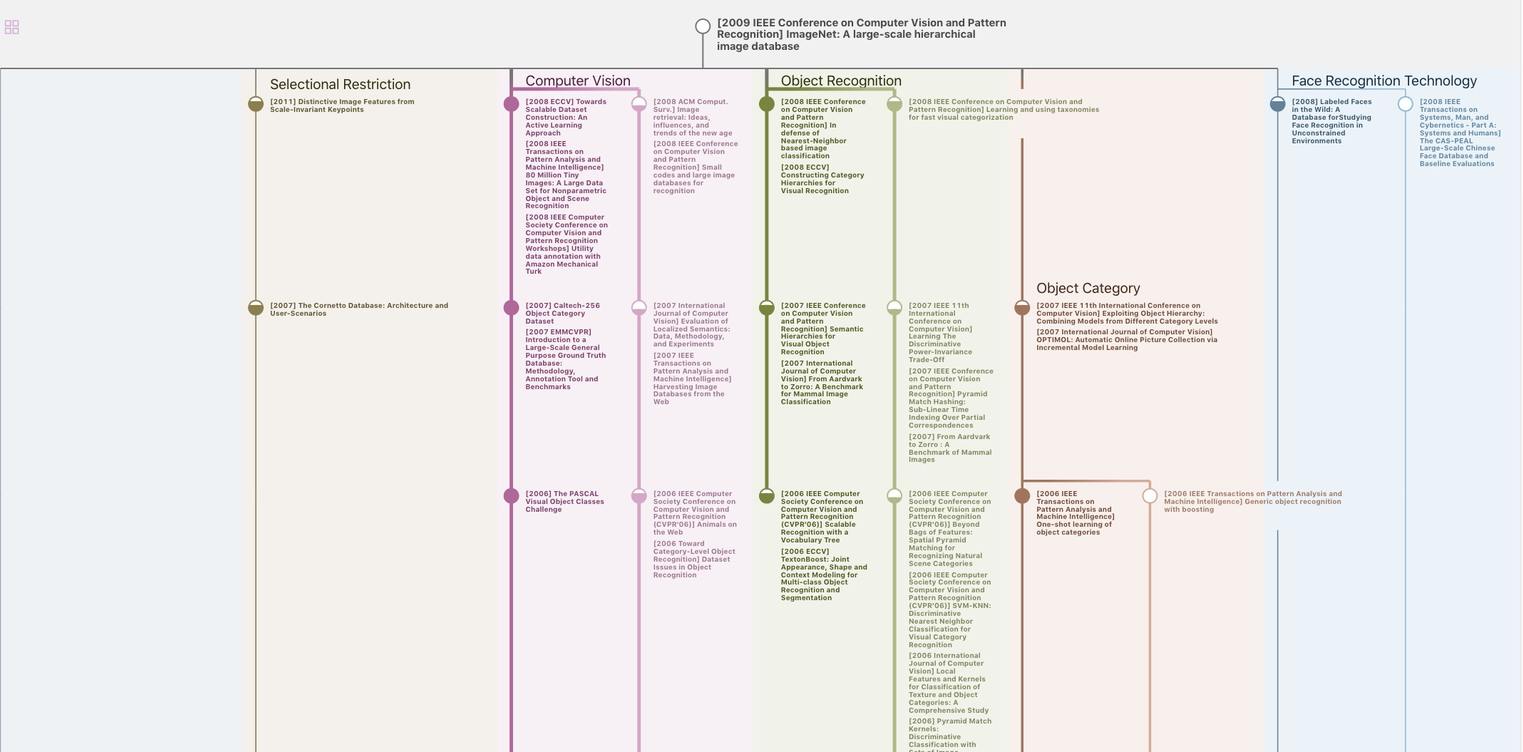
生成溯源树,研究论文发展脉络
Chat Paper
正在生成论文摘要