Benchmark of machine learning algorithms on transient stability prediction in renewable rich power grids under cyber-attacks
INTERNET OF THINGS(2024)
摘要
This study addresses the problem of ensuring accurate online transient stability prediction in modern power systems that are increasingly dependent on smart grid technology and are thus susceptible to cyber-attacks. Despite technological advancements, a considerable gap remains in the resilience of machine learning algorithms for stability prediction, which are not yet adequately equipped to counter the sophisticated and evolving nature of cyber threats. The research further assesses how cyber-attacks, alongside the integration of renewable energy and topological changes, affect these machine learning-based methods. Employed online, transient stability prediction methods are vital for real-time monitoring and forecasting of power system behavior in response to disturbances. Recognizing that attackers can disrupt communication and consequently manipulate the power system, the study simulates various scenarios to test the robustness of the proposed algorithms. Findings indicate that under cyber-attacks, machine learning algorithms underperform, leading to a significant decrease in the accuracy of transient stability predictions compared to those in normal operating conditions. This underlines the pressing need for advanced cybersecurity measures to safeguard the predictive capabilities of power systems.
更多查看译文
关键词
Transient stability prediction,Machine learning,False data injection attack,Protection relays,Renewable energy
AI 理解论文
溯源树
样例
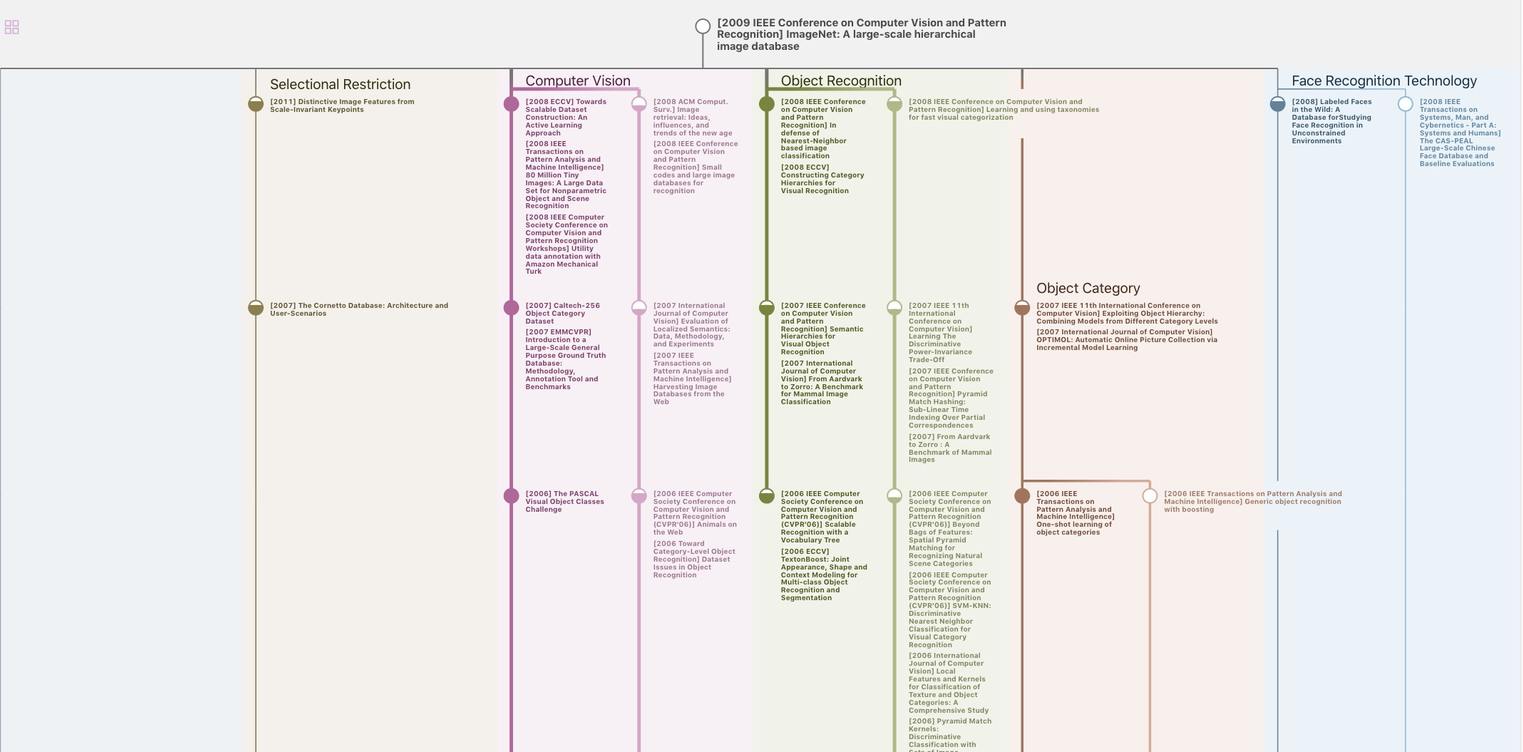
生成溯源树,研究论文发展脉络
Chat Paper
正在生成论文摘要