Vision-Language Models as a Source of Rewards
CoRR(2023)
摘要
Building generalist agents that can accomplish many goals in rich open-ended
environments is one of the research frontiers for reinforcement learning. A key
limiting factor for building generalist agents with RL has been the need for a
large number of reward functions for achieving different goals. We investigate
the feasibility of using off-the-shelf vision-language models, or VLMs, as
sources of rewards for reinforcement learning agents. We show how rewards for
visual achievement of a variety of language goals can be derived from the CLIP
family of models, and used to train RL agents that can achieve a variety of
language goals. We showcase this approach in two distinct visual domains and
present a scaling trend showing how larger VLMs lead to more accurate rewards
for visual goal achievement, which in turn produces more capable RL agents.
更多查看译文
AI 理解论文
溯源树
样例
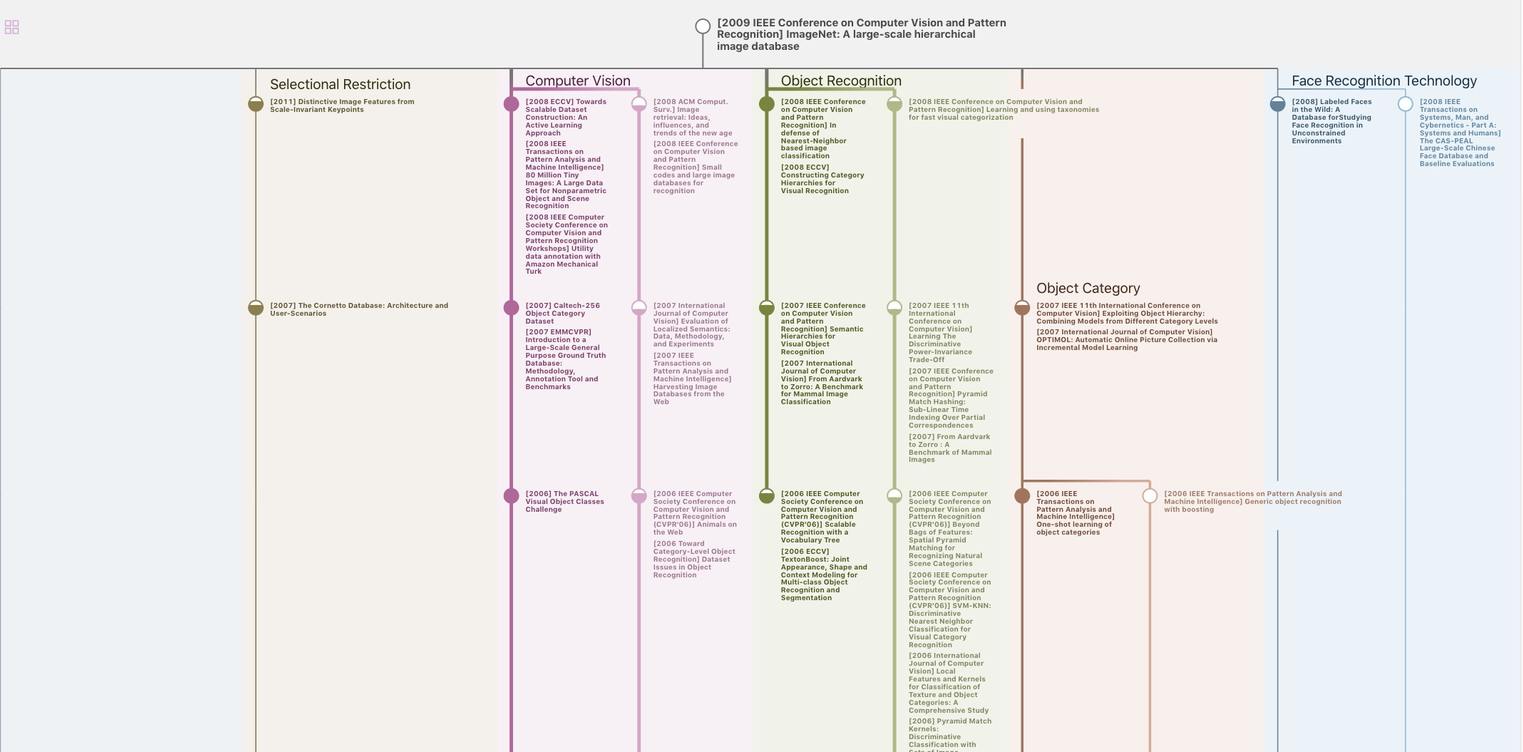
生成溯源树,研究论文发展脉络
Chat Paper
正在生成论文摘要