On the Expressivity of Recurrent Neural Cascades
AAAI 2024(2024)
摘要
Recurrent Neural Cascades (RNCs) are the recurrent neural networks with no cyclic dependencies among recurrent neurons. This class of recurrent networks has received a lot of attention in practice. Besides training methods for a fixed architecture such as backpropagation, the cascade architecture naturally allows for constructive learning methods, where recurrent nodes are added incrementally one at a time, often yielding smaller networks. Furthermore, acyclicity amounts to a structural prior that even for the same number of neurons yields a more favourable sample complexity compared to a fully-connected architecture.
A central question is whether the advantages of the cascade architecture come at the cost of a reduced expressivity. We provide new insights into this question. We show that the regular languages captured by RNCs with sign and tanh activation with positive recurrent weights are the star-free regular languages. In order to establish our results we developed a novel framework where capabilities of RNCs are assessed by analysing which semigroups and groups a single neuron is able to implement. A notable implication of our framework is that RNCs can achieve the expressivity of all regular languages by introducing neurons that can implement groups.
更多查看译文
关键词
KRR: Geometric, Spatial, and Temporal Reasoning,KRR: Action, Change, and Causality,KRR: Other Foundations of Knowledge Representation & Reasoning,ML: Learning Theory,ML: Neuro-Symbolic Learning,ML: Other Foundations of Machine Learning,ML: Time-Series/Data Streams
AI 理解论文
溯源树
样例
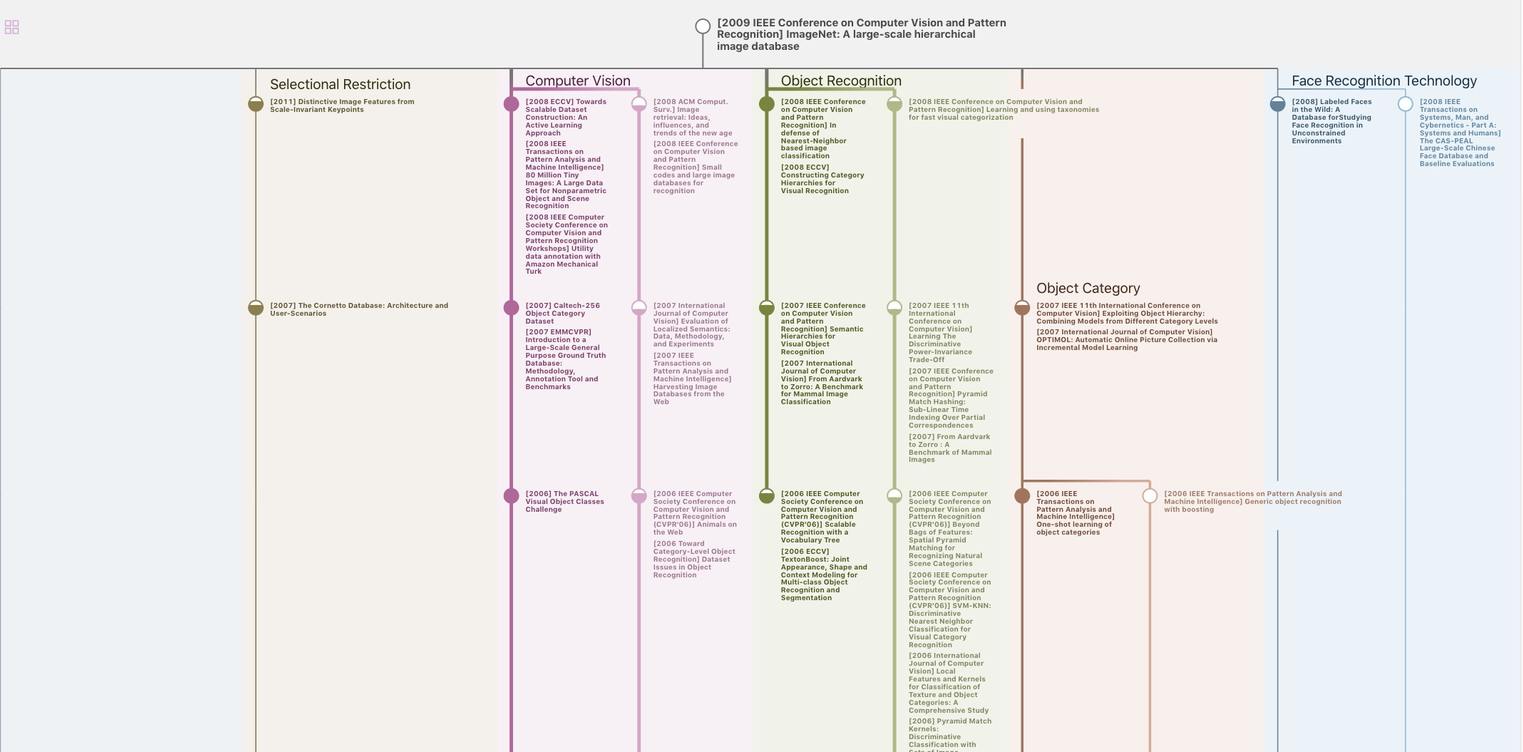
生成溯源树,研究论文发展脉络
Chat Paper
正在生成论文摘要