A Sim-to-Real Deep Learning-Based Framework for Autonomous Nano-Drone Racing
IEEE ROBOTICS AND AUTOMATION LETTERS(2024)
摘要
Autonomous drone racing competitions are a proxy to improve unmanned aerial vehicles' perception, planning, and control skills. The recent emergence of autonomous nano-sized drone racing imposes new challenges, as their similar to 10cm form factor heavily restricts the resources available onboard, including memory, computation, and sensors. This paper describes the methodology and technical implementation of the system winning the first autonomous nano-drone racing international competition: the IMAV 2022 Nanocopter AI Challenge. We developed a fully onboard deep learning approach for visual navigation trained only on simulation images to achieve this goal. Our approach includes a convolutional neural network for obstacle avoidance, a sim-to-real dataset collection procedure, and a navigation policy that we selected, characterized, and adapted through simulation and actual in-field experiments. Our system ranked 1st among seven competing teams at the competition. In our best attempt, we scored 115m of traveled distance in the allotted 5-minute flight, never crashing while dodging static and dynamic obstacles. Sharing our knowledge with the research community, we aim to provide a solid groundwork to foster future development in this field.
更多查看译文
关键词
Aerial systems: Perception and autonomy,embedded systems for robotic and automation,micro/nano robots
AI 理解论文
溯源树
样例
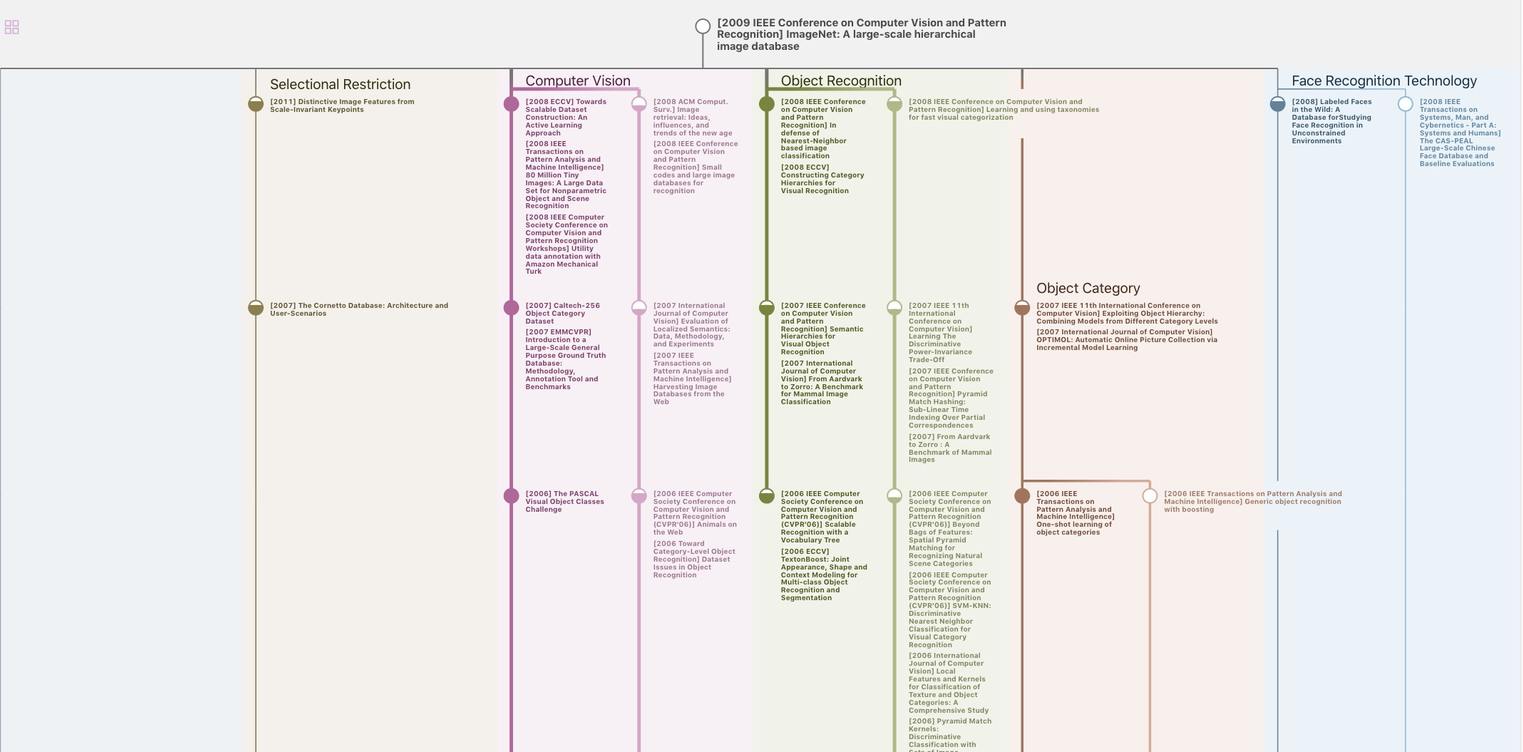
生成溯源树,研究论文发展脉络
Chat Paper
正在生成论文摘要