Vision Transformer-Based Deep Learning for Histologic Classification of Endometrial Cancer
arxiv(2023)
摘要
Endometrial cancer, the fourth most common cancer in females in the United
States, with the lifetime risk for developing this disease is approximately
2.8
endometrial cancer is important for effective patient management and
determining the best treatment modalities. This study introduces EndoNet, which
uses convolutional neural networks for extracting histologic features and a
vision transformer for aggregating these features and classifying slides based
on their visual characteristics into high- and low- grade. The model was
trained on 929 digitized hematoxylin and eosin-stained whole-slide images of
endometrial cancer from hysterectomy cases at Dartmouth-Health. It classifies
these slides into low-grade (Endometroid Grades 1 and 2) and high-grade
(endometroid carcinoma FIGO grade 3, uterine serous carcinoma, carcinosarcoma)
categories. EndoNet was evaluated on an internal test set of 110 patients and
an external test set of 100 patients from the public TCGA database. The model
achieved a weighted average F1-score of 0.91 (95
0.95 (95
F1-score and 0.86 (95
further validation, EndoNet has the potential to support pathologists without
the need of manual annotations in classifying the grades of gynecologic
pathology tumors.
更多查看译文
AI 理解论文
溯源树
样例
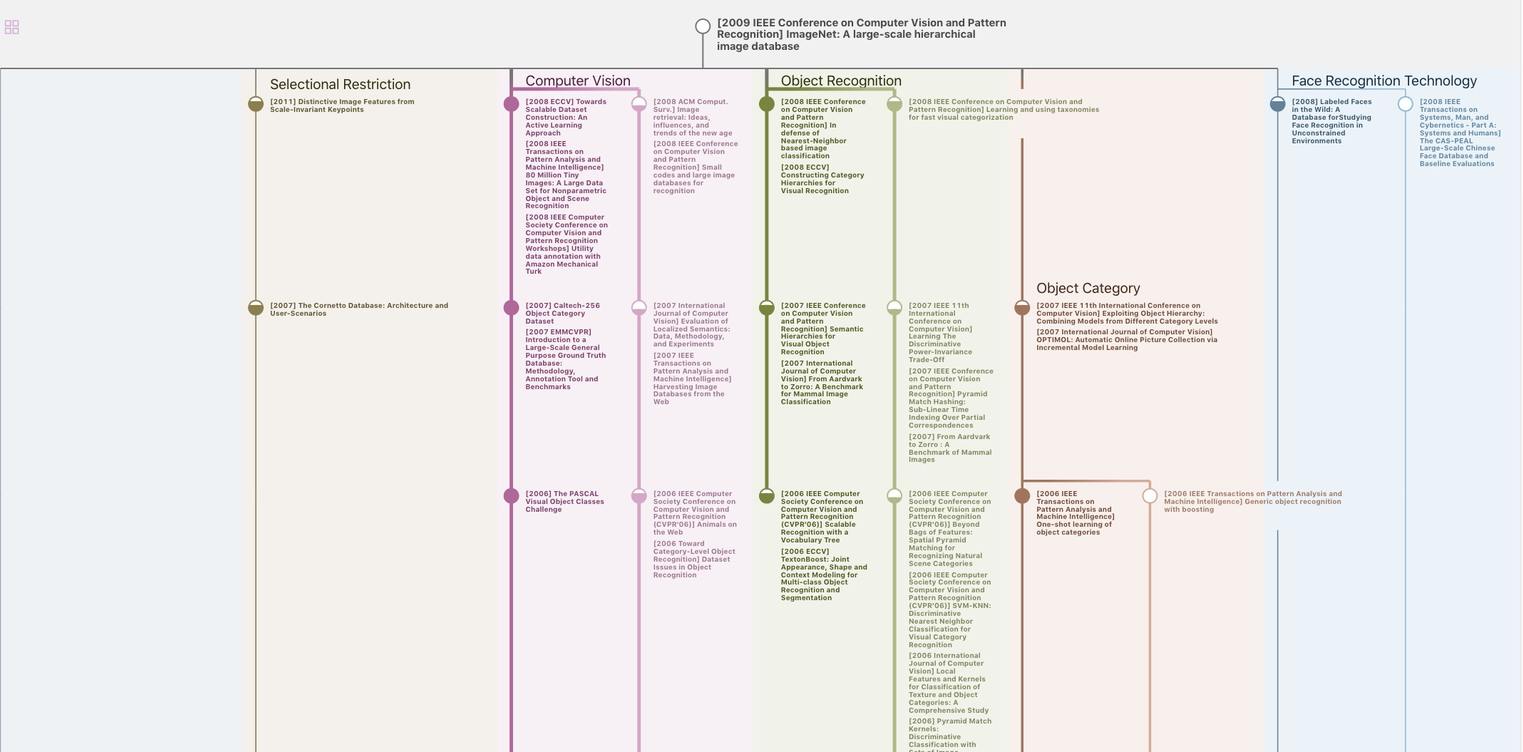
生成溯源树,研究论文发展脉络
Chat Paper
正在生成论文摘要