Towards Automatic Data Augmentation for Disordered Speech Recognition
CoRR(2023)
摘要
Automatic recognition of disordered speech remains a highly challenging task
to date due to data scarcity. This paper presents a reinforcement learning (RL)
based on-the-fly data augmentation approach for training state-of-the-art
PyChain TDNN and end-to-end Conformer ASR systems on such data. The handcrafted
temporal and spectral mask operations in the standard SpecAugment method that
are task and system dependent, together with additionally introduced minimum
and maximum cut-offs of these time-frequency masks, are now automatically
learned using an RNN-based policy controller and tightly integrated with ASR
system training. Experiments on the UASpeech corpus suggest the proposed
RL-based data augmentation approach consistently produced performance superior
or comparable that obtained using expert or handcrafted SpecAugment policies.
Our RL auto-augmented PyChain TDNN system produced an overall WER of 28.79% on
the UASpeech test set of 16 dysarthric speakers.
更多查看译文
关键词
Speech Disorders,Speech Recognition,Data Augmentation,Reinforcement Learning,SpecAugment
AI 理解论文
溯源树
样例
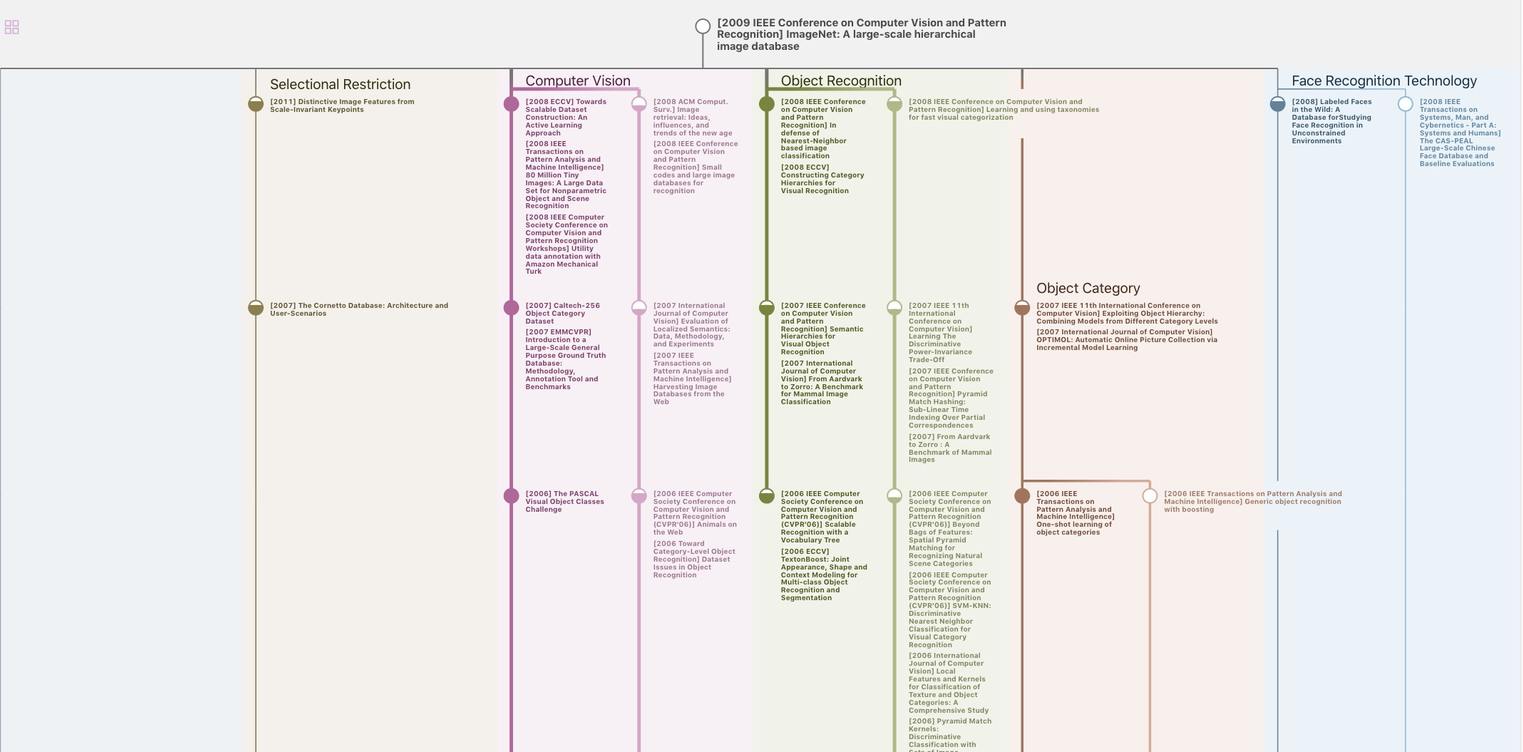
生成溯源树,研究论文发展脉络
Chat Paper
正在生成论文摘要