chronODE: A framework to integrate time-series multi-omics data based on ordinary differential equations combined with machine learning
biorxiv(2023)
摘要
Most functional genomic studies are conducted in steady-state conditions, therefore providing a description of molecular processes at a particular moment of cell differentiation or organismal development. Longitudinal studies can offer a deeper understanding of the kinetics underlying epigenetic events and their contribution to defining cell-type-specific transcriptional programs. Here we develop chronODE, a mathematical framework based on ordinary differential equations that uniformly models the kinetics of temporal changes in gene expression and chromatin features. chronODE employs biologically interpretable parameters that capture tissue-specific kinetics of genes and regulatory elements. We further integrate this framework with a neural-network architecture that can link and predict changes across different data modalities by solving multivariate time-series regressions. Next, we apply this framework to investigate region-specific kinetics of epigenome rewiring in the developing mouse brain, and we demonstrate that changes in chromatin accessibility within regulatory elements can accurately predict changes in the expression of putative target genes over the same time period. Finally, by integrating single-cell ATAC-seq data generated during the same time course, we show that regulatory elements characterized by fast activation kinetics in bulk measurements are active in early-appearing cell types, such as radial glial and other neural progenitors, whereas elements characterized by slow activation kinetics are specific to more differentiated cell types that emerge at later stages of brain development.
### Competing Interest Statement
The authors have declared no competing interest.
更多查看译文
AI 理解论文
溯源树
样例
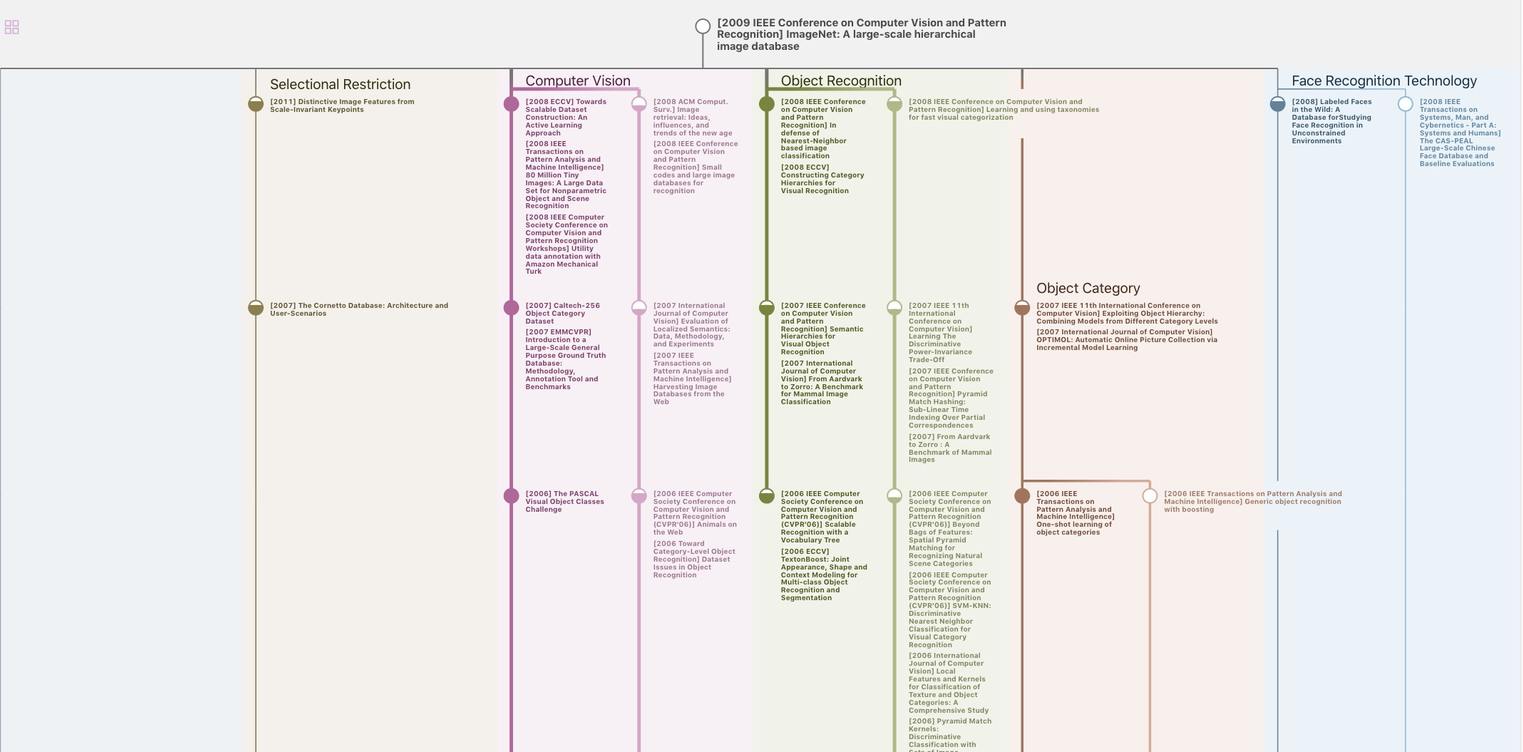
生成溯源树,研究论文发展脉络
Chat Paper
正在生成论文摘要