Enhanced YOLOv7 integrated with small target enhancement for rapid detection of objects on water surfaces
FRONTIERS IN NEUROROBOTICS(2023)
摘要
Unmanned surface vessel (USV) target detection algorithms often face challenges such as misdetection and omission of small targets due to significant variations in target scales and susceptibility to interference from complex environments. To address these issues, we propose a small target enhanced YOLOv7 (STE-YOLO) approach. Firstly, we introduce a specialized detection branch designed to identify tiny targets. This enhancement aims to improve the multi-scale target detection capabilities and address difficulties in recognizing targets of different sizes. Secondly, we present the lite visual center (LVC) module, which effectively fuses data from different levels to give more attention to small targets. Additionally, we integrate the lite efficient layer aggregation networks (L-ELAN) into the backbone network to reduce redundant computations and enhance computational efficiency. Lastly, we use Wise-IOU to optimize the loss function definition, thereby improving the model robustness by dynamically optimizing gradient contributions from samples of varying quality. We conducted experiments on the WSODD dataset and the FIOW-Img dataset. The results on the comprehensive WSODD dataset demonstrate that STE-YOLO, when compared to YOLOv7, reduces network parameters by 14% while improving AP50 and APs scores by 2.1% and 1.6%, respectively. Furthermore, when compared to five other leading target detection algorithms, STE-YOLO demonstrates superior accuracy and efficiency.
更多查看译文
关键词
object detection,water target detection,YOLOv7,unmanned surface vessel,small object detection
AI 理解论文
溯源树
样例
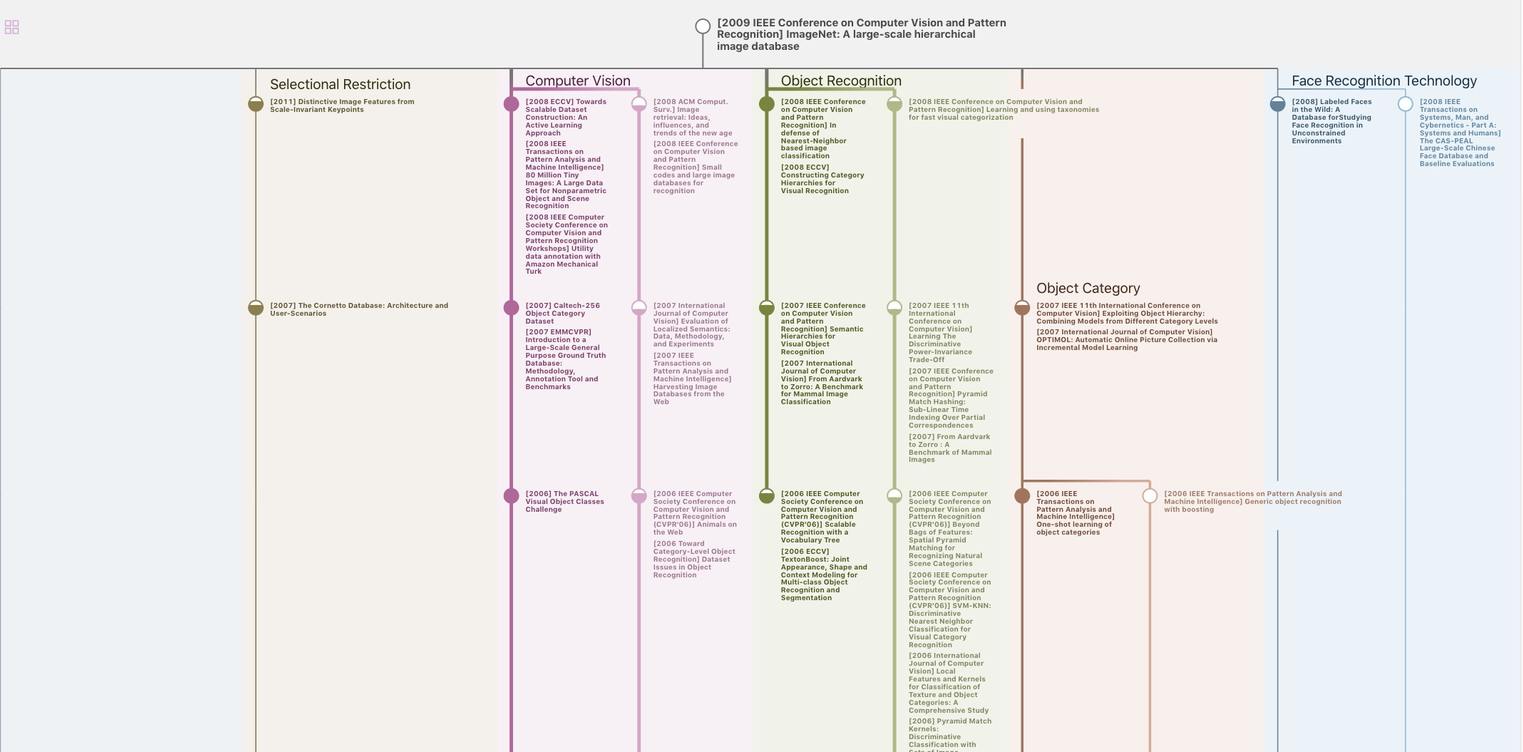
生成溯源树,研究论文发展脉络
Chat Paper
正在生成论文摘要